Understanding Seeding Density in 384 Well Plates
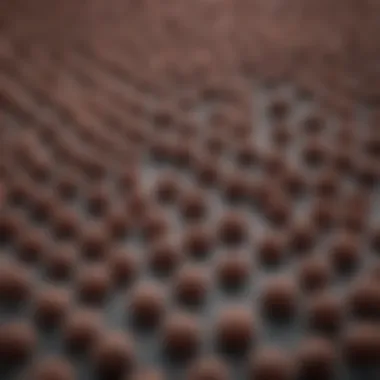
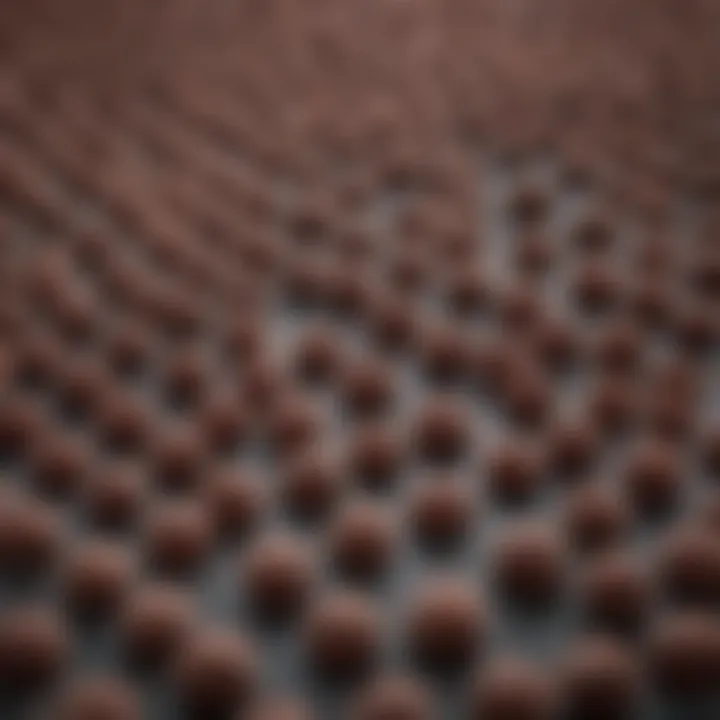
Intro
The subject of seeding density is of particular importance in high-throughput screening, especially when utilizing 384 well plates. These plates offer an efficient way to perform numerous experiments simultaneously, but the success of such experiments often hinges on understanding the appropriate seeding density. This article will explore the intricacies of seeding density, discussing its implications on cell behavior, experimental reproducibility, and best practices in various scientific fields.
Research Overview
Summary of Key Findings
One of the main observations in studies related to seeding density is that it directly affects cellular interactions and subsequent behaviors. High densities can lead to overcrowding, which may inhibit cell growth and alter phenotypic expressions. Conversely, too low of a density may fail to represent physiological conditions, thus skewing results. Research shows that optimal seeding densities vary widely based on the cell type and experimental goal. The right balance is essential for obtaining reproducible outcomes.
Methodologies Employed
Various methods have been employed to assess the impact of seeding density on cell behaviors. Some studies utilized real-time imaging to monitor cell growth and interactions over time. Others focused on endpoint assays to evaluate cellular responses at set intervals. These methodologies provide valuable data that help in determining best seeding practices across different applications, ensuring that researchers can tailor their approaches to specific experimental needs.
In-Depth Analysis
Detailed Examination of Results
In reviews of literature, it becomes evident that seeding density influences not only cell viability but also gene expression profiles. This correlation can be particularly significant when studying phenomena like drug responses or genetic modifications. Optimal densities can differ significantly; for instance, adherent cell types often require different conditions compared to suspension cells. Understanding these dynamics is crucial for researchers aiming for accuracy in their studies.
Comparison with Previous Studies
When examining past research, it is clear that recommendations often vary. Some studies advocate for a starting density based on empirical observations, while others lean towards theoretical models grounded in cellular biology. This inconsistency underlines the necessity for researchers to conduct preliminary experiments to determine the most suitable seeding density for their unique applications.
"Proper seeding density is fundamental. It can make or break an experiment, influencing both validity and reproducibility."
There is ongoing discourse on how best to calculate initial densities. Common approaches include the use of standard curves or historical datasets from prior experiments. Each method comes with its advantages and considerations, adding layers of decision-making for researchers.
Prelims to Well Plates
384 well plates play a significant role in contemporary laboratories, particularly in high-throughput screening applications. These plates facilitate numerous experiments in a compact format, thus optimizing resource use. Their popularity stems from their capacity to enhance efficiency and enable parallel processing of multiple samples. Researchers can conduct a variety of assays, from drug discovery to cell biology, maximizing the amount of data collected while minimizing the time needed for experiments.
One important consideration about 384 well plates is the uniformity of the environment each well provides. This uniformity is essential when analyzing cellular responses and making comparisons across experiments. However, achieving consistent results relies heavily on the proper management of seeding density.
Definition and Uses
A 384 well plate is a microplate containing 384 separate wells that can hold liquids for experimental purposes. Each well typically has a standardized volume, allowing researchers to conduct assays with controlled amounts of substances. Their small scale enables high-density setups, which is invaluable in research areas such as pharmacology, molecular biology, and toxicology.
The primary use of 384 well plates is in high-throughput screening, where thousands of tests can be performed simultaneously. This capability makes it easier to identify drug candidates, screen for potential toxins, and analyze gene activity. The design includes features that facilitate automated handling, making it easier to integrate these plates into robotic systems for further efficiency.
Historical Context
The advent of microplates dates back to the 1960s, but the 384 well format emerged later as laboratories sought to improve throughput and efficiency. As technology advanced, the need for more wells per plate became apparent. Early versions of microplates were limited to 96 wells, which presented constraints for researchers conducting high-volume assays.
With improvements in manufacturing processes and increases in demand for high-throughput screening, the 384 well plate gained traction in the late 1990s. This format has continually evolved, now incorporating features like optical clarity for imaging and specific surface treatments to enhance cell attachment. Its development has been crucial in pushing forward various fields of research, demonstrating the ongoing importance of well-designed experimental tools in scientific inquiry.
Understanding Seeding Density
Seeding density is a foundational concept in cell culture that holds significant implications for experimental outcomes, especially when working with 384 well plates. Determining the appropriate seeding density can greatly influence cell behavior, proliferation rates, and the overall validity of experimental results. Ignoring this critical factor may lead to unreliable data and skewed interpretations.
Defining Seeding Density in Cell Culture
In the realm of cell culture, seeding density refers to the number of cells planted per unit area or volume of culture medium. It is typically expressed as cells per square centimeter (cells/cmΒ²) or cells per milliliter (cells/mL). The significance of seeding density lies in its direct relationship with the conditions in which cells grow and interact. Higher densities can lead to competition for nutrients and space, while low densities may not provide sufficient cell-cell signaling or metabolic activity. Understanding how to accurately define and measure seeding density is essential, particularly in high-throughput systems where precision and consistency are key.
Importance of Seeding Density
The importance of seeding density cannot be overstated. It profoundly affects cell growth, differentiation, and experimental reproducibility. A few notable considerations include:
- Cell Growth Dynamics: The density at which cells are seeded can determine their growth phase and metabolic activity. For instance, cancer cells might exhibit different growth characteristics when seeded at varying densities, impacting drug response studies.
- Experimental Reproducibility: Maintaining consistent seeding densities across experiments is critical for reproducibility. Variations in cell numbers can yield divergent results, leading to challenges in data interpretation and scalability.
- Assay Conditions: Many assays are designed with specific seeding density parameters in mind. For example, during a cell viability assay, if the cell density is too high, it may result in overcrowding, which can mask true cell death rates. Conversely, a density that is too low may not generate sufficient signals for accurate detection.
"Optimal seeding densities are not just a technical detail; they are a cornerstone of experimental design that ensures validity and reliability of results."
While it may seem straightforward, achieving the right seeding density requires careful consideration of multiple factors, including cell type, medium composition, and environmental conditions. Taking these elements into account can enhance both the effectiveness of your cell culture practices and the insights drawn from your research.
Factors Influencing Seeding Density
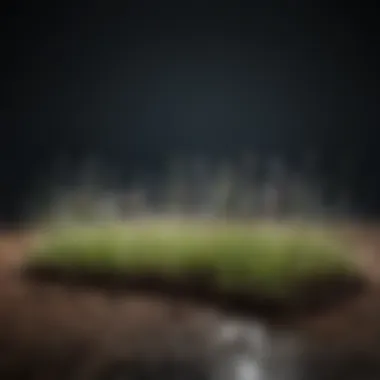
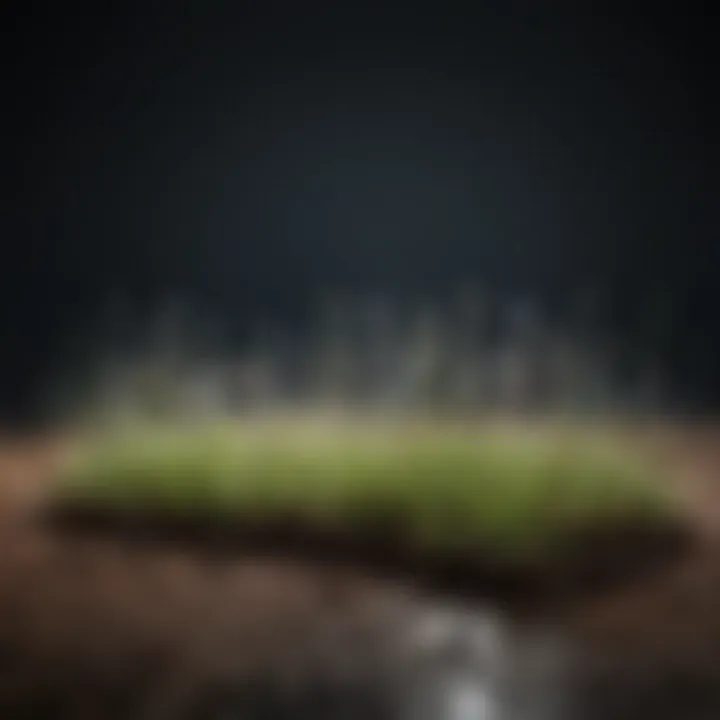
The seeding density in 384 well plates is not merely a technical specification; it is a crucial determinant of experimental success and cell behavior. A careful consideration of various factors is essential to optimize cell culture and achieve reliable results. These factors include cell type, medium composition, and environmental conditions. Understanding these elements is paramount for researchers as it directly impacts cell growth, function, and response to treatments. By evaluating these various influences, researchers can make informed decisions that enhance the accuracy and reproducibility of their experiments.
Cell Type Considerations
The biological characteristics of the cell type being used in an experiment can significantly dictate appropriate seeding density. Different cells have varied growth rates, sensitivities, and spatial requirements. For example, adherent cell lines such as HeLa or NIH 3T3 might require higher seeding densities compared to suspension cell lines like Jurkat cells.
It is also important to consider how cell types respond under crowded conditions. Certain cells may show altered behavior when they reach a specific density. In some cases, overcrowding can lead to contact inhibition, affecting cell proliferation and metabolism. Additionally, varying cell lines may necessitate tailored approaches, making it imperative to perform preliminary assessments to determine optimal density for each cell type. This facilitates clearer insights into their growth dynamics.
Medium Composition
The culture medium provides essential nutrients and support for cell survival and proliferation. Its composition can influence how cells interact with one another and their environment, which in turn affects seeding density. The choice of serum, growth factors, and supplements within the medium plays a critical role.
For instance, a medium enriched with high serum levels might allow for more rapid cell growth, which could necessitate lower starting densities to avoid overcrowding. Conversely, a simplified medium with limited nutrients might require a higher seeding density to ensure optimal growth conditions and prevent starvation effects. Researchers should evaluate medium attributes, including pH, osmolarity, and specific compositions, to find the best match for their experimental needs.
Environmental Conditions
Environmental conditions encompass factors such as temperature, CO2 concentration, humidity, and the presence of antibiotics or antifungals. These elements play a vital role in maintaining cell health and can substantially influence cell growth and behavior. For example, inadequate CO2 levels can impair pH balance in the medium, affecting nutrient absorption and cell welfare.
The incubation temperature is another crucial factor, as different cell lines have unique temperature preferences for optimal growth. Additionally, cells subjected to fluctuating environmental conditions may respond unpredictably, which can lead to inconsistencies in experimental outcomes. Therefore, a stable and controlled environment is necessary to ensure that selected seeding densities yield reproducible results.
Optimal seeding density is not just a number; it requires a comprehensive approach that takes into account these various influencing factors to achieve reliable cell culture results.
Optimal Seeding Densities for Various Applications
Understanding optimal seeding densities is crucial in a range of cellular applications. Proper seeding density influences not just cell growth but also experimental outcomes. Each application has unique requirements that must be addressed in order to achieve reliable and reproducible results.
In high-throughput screening, for example, accurate seeding densities allow researchers to identify potential drug candidates efficiently and effectively. Cell viability assays likewise rely on optimal densities to gain insights into cell health and function. Similarly, in drug response studies, the manner by which cells are seeded can greatly affect how they respond to therapeutic agents. Therefore, adjustments to seeding density could lead to significant shifts in data interpretation.
High-Throughput Screening
High-throughput screening (HTS) is a method that allows for rapid testing of large numbers of compounds or conditions in parallel. In this high-stakes environment, the seeding density must be meticulously calculated to ensure consistent cell behavior across the well plate.
The density of cells can affect the metabolic activity and responsiveness, which is fundamental for screening assays. If cell density is too high, it may lead to overcrowding, causing competition for nutrients and space, thereby compromising cell health. Conversely, a low density can yield insufficient cellular interactions that are essential for effective screening. High-throughput systems often incorporate robotics and automation to achieve precise control over seeding density, reducing human error and enhancing reproducibility.
Cell Viability Assays
Cell viability assays are vital in determining the health of cells under various conditions. Here, choosing the right seeding density is essential for obtaining accurate and reliable results. If the seeding density is optimized, the assay can reflect the true state of the cells. For example, an appropriate density facilitates better detection of toxic effects or the benefits of treatments.
Typically, cells should be seeded at a specific density that allows for optimal growth without leading to excessive confluence during the duration of the assay. If the cellular environment is overcrowded, it can mask the effects of treatments, leading to misleading conclusions. Thus, calibrating seeding density is paramount to ensure that the cell behavior being measured is both genuine and interpretable.
Drug Response Studies
In drug response studies, the relationship between seeding density and drug efficacy becomes particularly apparent. Proper seeding densities ensure that there is a representative cell population that can respond predictably to drugs. Depending on the drug and the expected cellular targets, the optimal density can vary significantly.
For instance, certain drugs may exert effects only at specific cellular densities; too few cells may lead to an inadequate response, while too many can inhibit cellular activities that are essential for drug action. Therefore, conducting preliminary experiments to quantify the optimal seeding density is a critical step for ensuring accurate interpretation of drug responses.
Optimal seeding densities can significantly impact data quality and reproducibility in research applications. Small adjustments in density can yield large differences in experimental outcomes across various fields.
In summary, understanding and implementing optimal seeding densities is integral to maximizing the efficiency of various applications involving 384 well plates. This affects not just growth conditions but also the reliability of experimental data, which is crucial for advancing scientific research.
Techniques for Calculating Seeding Density
Understanding seeding density in 384 well plates is crucial for ensuring optimal growth conditions for cells in various experiments. Numerous techniques exist to accurately measure and calculate the seeding density, impacting the outcome and reliability of research findings. This section will cover three main techniques: direct cell counting, automated systems, and mathematical formulations. Each method has its advantages and unique considerations that researchers must evaluate to select the most appropriate for their specific applications.
Direct Cell Counting
Direct cell counting is a fundamental technique used to determine the number of cells in a given volume. This method involves using a hemocytometer or similar counting chamber, where a small sample of the cell suspension is placed under a microscope. Researchers can then manually count the cells in a defined area, allowing for accurate determination of cell concentration.
Benefits of Direct Cell Counting:
- Provides immediate results.
- Allows for visual confirmation of cell morphology and viability.
- It can be modified for various cell types.
Considerations:
- Prone to human error and subjectivity in counting.
- Requires proper training and attention to technique for accuracy.
- Time-consuming compared to automated methods.
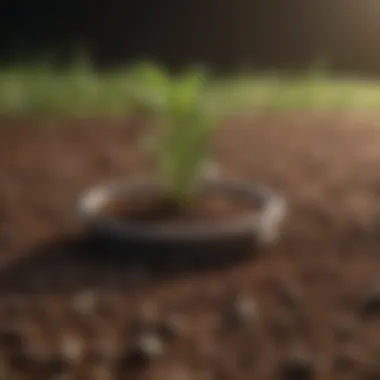
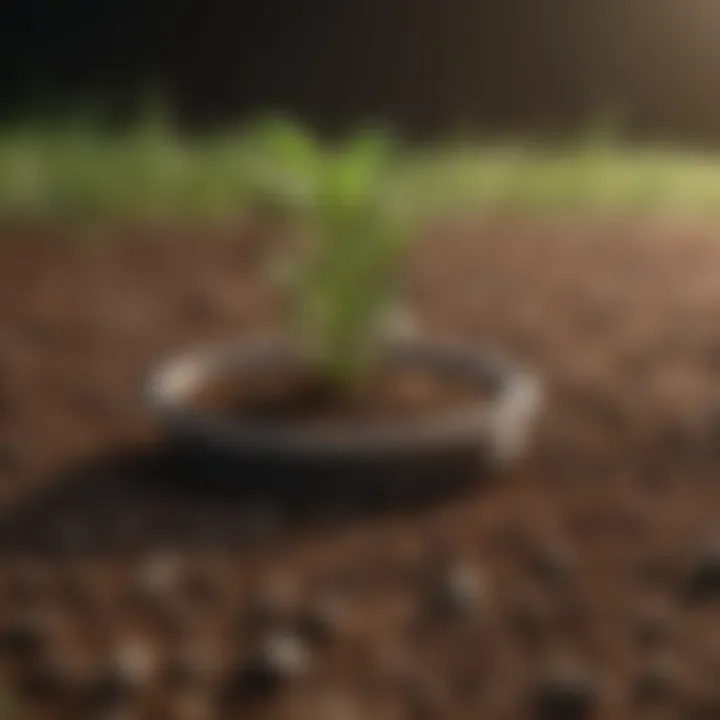
Automated Systems
Automated systems for calculating seeding density have gained popularity due to their efficiency and reduced manual effort. Machines like automated cell counters employ advanced technology to analyze cell samples quickly. These systems often utilize imaging, laser-based detection, or fluorescence to distinguish viable cells from debris.
Benefits of Automated Systems:
- Significantly speeds up the counting process, suitable for high-throughput applications.
- Reduces human error associated with manual counting.
- Provides consistent results across multiple samples.
Considerations:
- Initial investment in equipment can be high.
- Calibration and maintenance of equipment are essential for accurate results.
- Some systems may not work effectively with certain cell types or densities.
Mathematical Formulations
Mathematical formulations are essential for calculating seeding density based on total cell counts and the volume of the dilution or plating medium. Commonly used equations, like the dilution factor and final concentration calculations, enable researchers to plan and adjust their experiments based on desired cell density.
Benefits of Mathematical Formulations:
- Offers a theoretical framework to predict cell behavior in various environments.
- Facilitates quick adjustments to seeding protocols based on initial observations.
- Can be applied across various applications in cell culture.
Considerations:
- Requires accurate initial data (total cell count, volumes used) for reliability.
- Assumes homogeneity in cell suspension, which may not always be the case.
Effective calculation of seeding density ensures consistency and reproducibility, critical in high-throughput screening and other experimental contexts.
By employing one or more of these techniques, researchers can achieve precise control over cell seeding density, ultimately leading to more successful experimental outcomes in their studies.
Common Pitfalls in Seeding Density
In this section, we explore Common Pitfalls in Seeding Density when working with 384 well plates. Understanding these pitfalls is vital for achieving reliable results and optimizing experimental conditions. Amid the complexities of cell culture, specific missteps in seeding density can lead to significant consequences, altering interpretability of results and even threatening the integrity of entire studies. Recognizing these pitfalls is the first step to preventing them, thereby improving experimental robustness and reproducibility.
Overcrowding Effects
Seeding cells too densely can lead to overcrowding in well plates. High-density cultures often face nutrient depletion and waste accumulation. Here's how overcrowding impacts cell growth and experimental outcomes:
- Nutrient Competition: As cell numbers increase, individual cells compete for essential nutrients in the medium. This competition can stunt growth, leading to skewed results in assays targeting cell proliferation or viability.
- Altered Cell Behavior: Overcrowding can induce stress responses in cells. These responses vary by cell type but generally include reduced differentiation and altered gene expression, which can confound experimental results.
- Increased Cell Death: High cell densities often result in hyperoxia or hypoxia conditions, depending on cellular respiration rates, which might contribute to increased rates of apoptosis. Such effects can heavily distort data, particularly in drug efficacy studies.
It is crucial to establish optimal seeding densities before beginning experiments. Avoiding overcrowding requires continual assessment of cell growth rates and adjustments to initial seeding plans.
Inadequate Seeding
On the other end of the spectrum, inadequate seeding is another critical pitfall. Insufficient cell numbers may lead to several disadvantages:
- Poor Experimental Control: A low density can lead to a lack of statistical power in assays, making it challenging to draw significant conclusions from results. Cells may not adequately represent the biological model intended.
- Unreliable Data: Low seeding density may result in variability without a defined pattern, undermining the reliability of data and increasing experimental noise. This noise can obscure important signals, making it difficult to identify meaningful trends.
- Inefficient Use of Resources: Using expensive reagents, like specialized media or compounds, on inadequate cell plts may lead to wasted resources. Poorly planned experiments that utilize too few cells can lead to repeated trials and inefficient research timelines.
In practice, ensuring appropriate and reproducible seeding density is vital. Optimal densities will differ based on the cell type and the specific aim of the experiment, requiring researchers to tailor their approaches accordingly.
Technological Advancements
Technological advancements play a pivotal role in the study of seeding density within 384 well plates. As scientific inquiries become more complex, researchers require precise methods for cell seeding to ensure accurate and reproducible results. Innovations in technology provide significant benefits that address the challenges associated with cell culture. This section will outline key advancements in robotics and artificial intelligence, which streamline processes related to seeding density.
Robotics in Cell Seeding
Robotic systems have emerged as vital tools in laboratory environments, particularly in seeding cells in 384 well plates. The automation of cell seeding minimizes human error and enhances consistency in experiments. Robotic arms equipped with precise pipetting systems allow for rapid and accurate cell placement. This capability supports high-throughput screening efforts, as numerous plates can be processed simultaneously.
The advantages of robotic systems include:
- Increased Throughput: Robots can seed multiple plates within a short time, considerably improving overall productivity.
- Reproducibility: Consistent robotic techniques lead to less variance in seeding density, which is crucial for experiments reliant on uniformity.
- Flexibility: Modern systems can be programmed to accommodate various protocols, making it possible to adapt to different experimental needs.
However, there are considerations when implementing robotics:
- Cost: Initial investment in robotic systems can be high, which may not be feasible for all labs.
- Technical Skill: Personnel must be trained to operate and maintain these systems effectively.
In summary, robotics in cell seeding offer essential efficiency and reliability that contribute significantly to the overall accuracy of experiments involving seeding density.
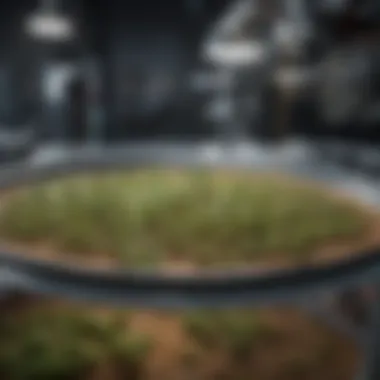
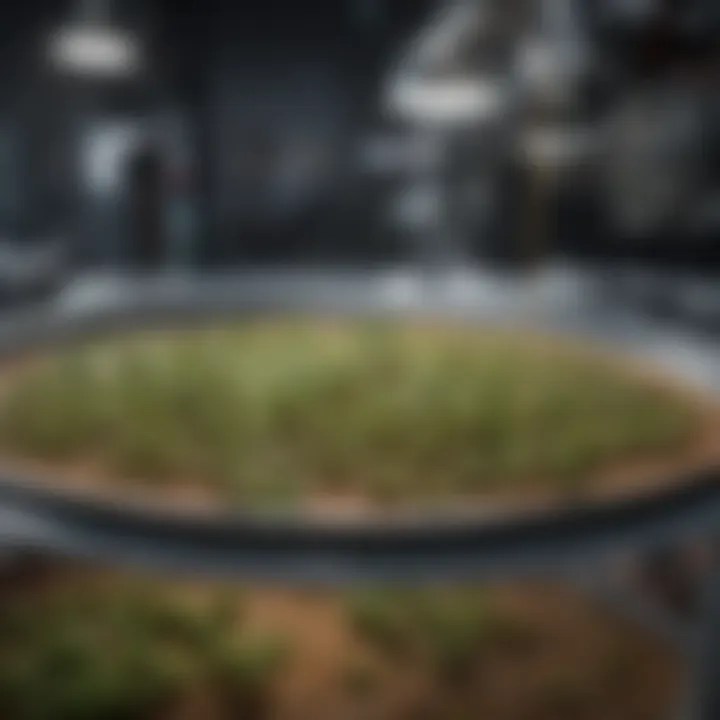
AI and Machine Learning Applications
Artificial intelligence and machine learning technologies are revolutionizing how researchers approach seeding density in high-throughput applications. AI algorithms can analyze vast datasets quickly, providing insights that were not possible with traditional methodologies. These technologies can predict optimal seeding densities based on historical data and experimental outcomes.
Key applications of AI in this field include:
- Predictive Modeling: Machine learning can forecast the ideal seeding density for specific cell types under varying conditions, reducing trial and error.
- Anomaly Detection: AI systems are capable of identifying outliers in data, which can indicate issues with seeding or environmental factors, alerting researchers to potential problems early on.
- Optimization: Continuous learning algorithms can refine seeding conditions iteratively, leading to improved experimental designs over time.
The integration of AI carries several advantages:
- Data-Driven Decisions: Utilizing AI for making informed decisions helps to enhance the reliability of results.
- Adaptability: AI systems can adjust protocols based on real-time data, leading to more responsive experimental strategies.
Nonetheless, challenges remain in implementing these technologies, such as:
- Data Quality: The efficacy of machine learning models heavily depends on the quality and volume of input data.
- Interpretability: Understanding the rationale behind AI recommendations can be complex, which may hinder trust in these systems.
Thus, AI and machine learning applications hold great promise for optimizing seeding density, potentially leading to breakthroughs in various fields of research.
Case Studies and Practical Examples
Understanding seeding density is not just theoretical; it has practical applications that can significantly impact research outcomes. Case studies serve as important references, illustrating how variations in seeding density lead to different results in specific contexts. They provide valuable insight into optimizing conditions for various cell types and experiments. The real-world applications of seeding density can spotlight how researchers have navigated challenges and improved processes. This pragmatic approach underlines the necessity of tailored strategies and methodological rigor, ensuring the validity of experimental data.
Seeding Density in Cancer Research
In cancer research, adjusting seeding density is critical. Cancer cells often exhibit unique growth patterns that may not align with traditional cell lines. For instance, when investigating the efficacy of novel cancer therapies, researchers have found that high seeding densities can mask therapeutic effects. A study demonstrated that when cancer cell lines were seeded at densities exceeding optimal levels, cell-cell interactions changed significantly, leading to altered responses to drug treatments. Thus, determining the right seeding density can enhance experimental reliability.
Additionally, the consistency of results across multiple experiments is crucial in cancer studies. One practical example is how varying the seeding density can influence apoptosis assays. An experiment showed that a density too low resulted in inaccurate depictions of drug efficacy, while too high led to suppression of cell death signals. The careful balancing of seeding density thus ensures that researchers gain a true reflection of therapeutic effectiveness.
Applications in Stem Cell Biology
In stem cell biology, seeding density plays a pivotal role. The behavior of stem cells greatly depends on their environment, including how densely they are packed. An application of this principle was evident in a study focusing on the differentiation of mesenchymal stem cells. Researchers observed that increasing seeding density promoted cellular interactions that facilitated differentiation into osteoblasts.
Moreover, the maintenance of pluripotency in embryonic stem cells is sensitive to seeding density. A too-dense environment can induce unwanted differentiation while a sparse one can hinder growth. By examining various seeding densities, researchers documented optimal conditions that promoted self-renewal while minimizing spontaneous differentiation.
In summary, these examples illustrate the profound effects of seeding density in both cancer research and stem cell biology. Effectively managing this variable can lead to significant improvements in experimental outcomes and broaden the understanding of cellular behaviors, ensuring the results are both reproducible and relevant.
Future Directions in Seeding Density Research
The exploration of seeding density in 384 well plates is not just a current focal point but also a gateway to future advances in biological research. As scientists strive to refine their experimental designs, understanding the future trajectory of seeding density research becomes essential, particularly given its implications on cell behavior and experimental reproducibility. The evolving landscape of biotechnology and cell culture presents opportunities to adapt and integrate novel techniques that enhance both efficacy and innovation. As research progresses, several specific elements warrant attention in the domain of seeding density.
Emerging Trends
Emerging trends in seeding density are reflective of both technological advancements and shifts in research focus. The use of microfluidics has gained traction, allowing for precise control over cell distribution, enhancing reproducibility in experiments. This technology facilitates the manipulation of small volumes of liquids, ensuring that seeding density can be optimized for various applications. Furthermore, high-throughput technologies continue to influence seeding practices, frequently allowing researchers to conduct multiple assays simultaneously in larger populations of cells. This is critical for fields such as drug discovery, where variations in cell density can greatly affect results.
Additionally, an increased emphasis on personalized medicine drives a demand for novel cell-based models. Researchers are exploring how seeding density can affect the phenotypic expression of cultured cells, especially in the context of patient-derived cells. These real-world implications lend significant value to refined seeding practices and methodologies.
Potential Innovations
Potential innovations in seeding density research are vast, ranging from automation to artificial intelligence. Robotics, already instrumental in cell seeding, continues to advance, promising to reduce human error and increase throughput. Furthermore, AI and machine learning are beginning to play a role in predicting optimal seeding densities based on experimental parameters. By analyzing large datasets, these systems can provide insights that may not be immediately obvious, paving the way for tailored protocols that enhance experimental outcomes.
"The intersection of technology and biology holds the promise of revolutionizing our understanding of seeding densityβs role in cell behavior."
Moreover, enhancements in cell culture media composition and scaffolding materials may also reshape how researchers approach seeding density. Customizing the environment in which cells are seeded could lead to more predictable behaviors and improved viability. Innovations in bioprinting and scaffolds can help mimic in vivo conditions, allowing for further exploration of seeding density impacts in complex tissue models.
Overall, the future of seeding density research is poised for transformation. By embracing technological advances, adapting to emerging trends, and integrating potential innovations, researchers can enhance the reliability and applicability of their work in high-throughput formats. The implications are profound, with possibilities that extend across various scientific disciplines.
Epilogue
In this article, the exploration of seeding density in 384 well plates has highlighted several crucial aspects that impact experimental outcomes. Understanding the importance of proper seeding density is not merely an academic exercise; it is essential for achieving reliable and reproducible results in a variety of research applications. Adhering to optimized seeding practices ensures that cells exhibit expected behaviors, whether in high-throughput screenings, drug response studies, or viability assays.
Summary of Key Points
- Definition and Importance: Seeding density is the number of cells placed in a well. This parameter significantly influences cellular interactions and experimental results.
- Factors Influencing Density: Various elements such as cell type, medium composition, and environmental conditions require careful consideration when determining appropriate densities.
- Optimal Practices: Recommended densities vary based on specific applications. Understanding these nuances can lead to improved experimentation.
- Calculation Techniques: Direct cell counting, automated systems, and mathematical formulations are methods available to determine appropriate densities accurately.
- Common Issues: Recognizing pitfalls like overcrowding and inadequate seeding helps avoid problems that can compromise research integrity.
- Technological Advancements: Innovations in robotics and AI have the potential to enhance the precision and efficiency of cell seeding practices.
- Future Directions: Emerging trends in seeding density research pave the way for further advancements and a deeper understanding of cellular behavior.
Implications for Future Research
The studies and insights provided on seeding density can lead to several implications for future research. Firstly, as technology continues to evolve, integrating more sophisticated methods for calculating and implementing seeding densities will enhance the accuracy of experimental design. Additionally, investigating the effects of varying densities on different cell types could yield new insights into cellular behavior and treatment efficacy.
Encouraging interdisciplinary approaches may also foster innovation in this sphere, merging biology with computational analysis, for instance. Researchers should prioritize collaborations that utilize these advanced methodologies for even more refined outcomes.
Ultimately, as scientists delve deeper into these practices, the potential for breakthroughs in drug discovery, chronic disease treatment, and regenerative medicine increases significantly. By embracing a systematic approach to seeding density, the scientific community can unlock new levels of understanding and applications within the realms of cellular biology and biomedicine.