Understanding Protein Databases: Structure and Function
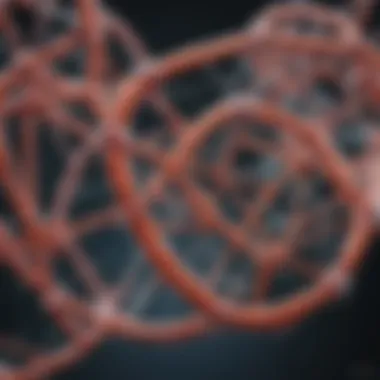
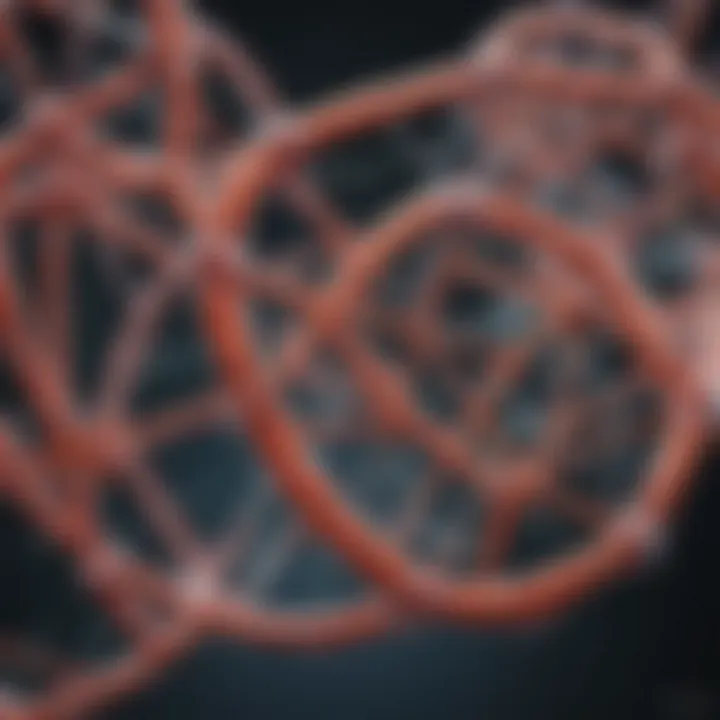
Intro
Protein databases serve as invaluable resources in the field of biological research. They hold a wealth of information on various proteins, including their structure, function, and potential applications in scientific inquiry. This article aims to provide a detailed exploration of protein databases, emphasizing their significance in modern biology. Understanding the structure and function of these databases will offer insights into how they contribute to various research domains, such as drug discovery and genomics.
Research Overview
Summary of Key Findings
Protein databases can be classified based on their focus. Some databases are dedicated to sequence data, like UniProt, which holds extensive protein sequences and functional information. Others, such as the Protein Data Bank (PDB), focus on 3D structures of proteins. The interplay between these databases enables researchers to glean a comprehensive understanding of proteins from different angles. This synthesis of structural, functional, and sequence data forms the backbone of many bioinformatics studies, allowing for more informed hypotheses and experimental designs.
Methodologies Employed
The methodologies used in the collection and storage of protein data vary. Many databases utilize high-throughput techniques to gather data efficiently. For example, mass spectrometry is commonly employed for proteomics studies to identify and quantify proteins in complex mixtures. Additionally, X-ray crystallography and nuclear magnetic resonance are often used to determine protein structures, which are then deposited in databases like PDB. The rigorous processes in data validation ensure that the information remains reliable for researchers.
In-Depth Analysis
Detailed Examination of Results
Dividing protein databases into distinct categories allows researchers to choose the right resource according to their needs. UniProt, as mentioned earlier, primarily focuses on sequences. Its annotations help highlight the biological roles of various proteins, which is crucial for functional genomics studies. Meanwhile, the PDB provides detailed structural data that can inform drug design and protein engineering projects. By analyzing the structural patterns, scientists can make predictions about how a protein interacts with other molecules, thus guiding experimental approaches in a laboratory setting.
Comparison with Previous Studies
Comparative studies shed light on the evolution of protein databases. For instance, earlier databases were often siloed, housing limited types of information. The advent of integrated databases has changed this landscape, facilitating cross-referencing among data types. This improvement enhances the user experience by providing comprehensive tools for researchers. The impact of these advancements is reflected in the increasing number of publications relying on data from these protein databases, showcasing their growing importance in scientific research.
"The role of protein databases in understanding complex biological systems cannot be overstated. They serve as fundamental resources in accelerating discoveries in life sciences."
Through this overview and analysis, it becomes evident that protein databases are not merely collections of data, but rather dynamic resources that drive research forward. Their structural and functional insights empower scientific advancements across multiple disciplines.
Prologue to Protein Databases
Protein databases play a crucial role in current biological research. Understanding these databases allows researchers to utilize a wealth of information on protein structure and function. As protein databases evolve, their importance in fields like genomics, drug development, and biotechnology becomes even more evident. This exploration into the intricacies of protein databases will reveal their fundamental aspects, benefits, and considerations.
Defining Protein Databases
A protein database is a collection of protein-related data that is organized for easy access and analysis. These databases typically include information such as amino acid sequences, structural data, and functional annotations. They serve as a resource for scientists, allowing quick retrieval of critical information for research and development purposes.
Protein databases can be categorized into primary and secondary databases. Primary databases are those that directly collect raw data, such as UniProt, which compiles protein sequences and functional annotations. In contrast, secondary databases analyze and provide information derived from the primary sources. The distinction is important for identifying the quality and types of information available.
Moreover, specific datasets in protein databases can be utilized to address various scientific questions. This highlights the versatility and necessity of having a well-structured protein database, particularly as biological research pivots towards a more data-driven approach.
History and Evolution of Protein Databases
The concept of protein databases began to take shape in the 1970s when researchers recognized the need for accessible data on protein sequences. Early efforts, such as the Amino Acid Sequence Database established in 1986, marked the beginning of a systematic approach to storing protein information. Following this, the creation of databases like the Protein Data Bank (PDB) in 1971, revolutionized how structural data was shared among scientists.
Over the decades, protein databases have exploded in volume and complexity. The rapid advancement in genome sequencing technologies and computational methods facilitated the expansion and refinement of these databases. Today, protein databases are integral to bioinformatics, providing tools and infrastructure necessary for analysis and interpretation of vast biological data sets.
The transition from simple sequence listings to comprehensive databases that include structural information and functional annotations has been significant. This evolution reflects the increasing understanding of the protein world and the necessity for detailed, organized biological data. As a result, modern databases are now essential for research and clinical applications.
"The evolution of protein databases marks a pivotal moment in the life sciences, transforming how researchers access and utilize biological data."
As we continue through this article, the focus will shift to the types of protein databases, their key components, and their applications in research.
Types of Protein Databases
Understanding the types of protein databases is fundamental to appreciating their role in biological research. Protein databases serve a variety of functions, from storing sequences to providing structural information. The classification into primary and secondary databases, alongside domain-specific resources, aids researchers in choosing the right tool for their specific needs.
Primary vs. Secondary Databases
Primary databases collect and organize raw data derived from direct experimental sources. These include sequence data, structure coordinates, and functional annotations. An example of a primary database is UniProt, which provides a centralized resource of protein sequences and their functional information.
The main characteristics of primary databases include:
- Direct data input: Data is collected from experimental results by researchers, ensuring accuracy.
- Data variety: Sequence, domain, or structure data can be stored.
- Current updates: These databases continuously receive data, contributing to their evolving nature.
In contrast, secondary databases rely on data that has been derived from primary databases. These resources often analyze, summarize, or otherwise interpret primary data for specialized uses. For example, the Protein Data Bank (PDB) primarily holds 3D structural data and is dependent on primary sequence databases like UniProt for its functionality.
Features of secondary databases include:
- Data interpretation: They focus on interpretation of available data, enhancing user understanding.
- Specialized focus: They often target specific areas, such as structure or function.
- Less frequent updates: Changes in these databases are usually dependent on the primary data being updated.
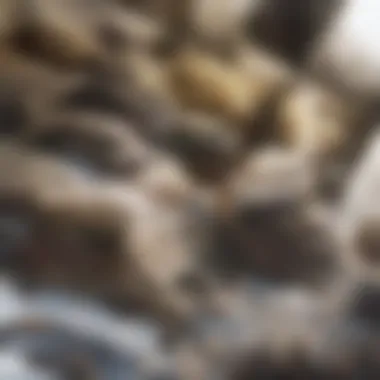
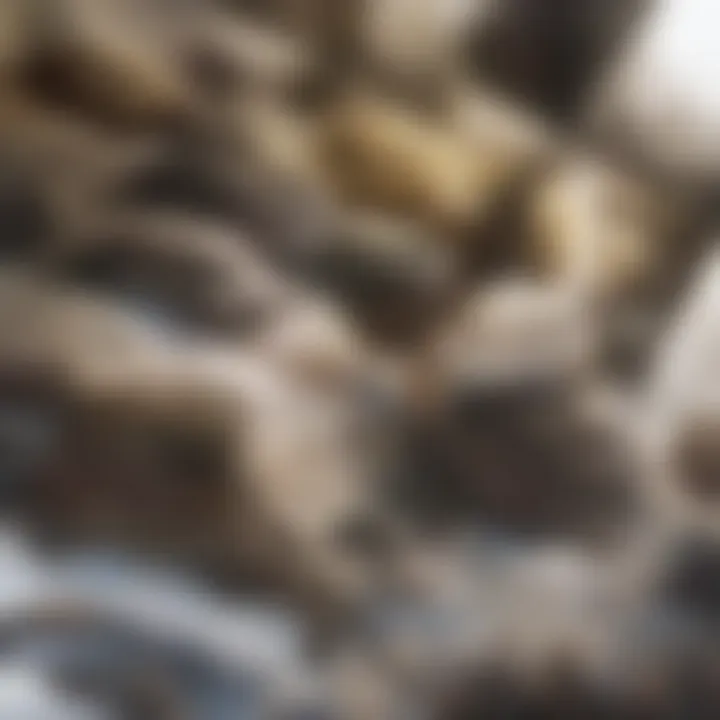
Overall, the distinction between primary and secondary databases highlights the critical pathways through which data is processed and disseminated across the scientific community.
Domain-Specific Databases
Domain-specific databases cater to particular fields or types of analyses within biological research. They are designed to house specialized data sets relevant to a specific area of study. An example is BRENDA, which focuses on enzyme data.
These databases provide several benefits:
- Tailored information: They offer specialized data that may not be found in general databases, enhancing the depth of information available to researchers.
- Focused search capabilities: Users can perform queries to retrieve data pertinent to their specific area of study, rather than sifting through irrelevant information.
- Community-driven resources: Many of these databases are developed with input from domain experts, ensuring content relevancy and accuracy.
In sum, understanding the myriad types of protein databases, from primary to domain-specific, equips researchers with the necessary knowledge to effectively navigate the vast landscape of protein data. The selection of the appropriate database can greatly enhance the quality of scientific research and data analysis.
Key Components of Protein Databases
Protein databases are integral to the field of bioinformatics, acting as repositories for data that facilitate research in molecular biology, genetics, and biochemistry. Understanding the key components of these databases provides valuable insights into how they operate, the benefits they offer, and the considerations researchers must observe when utilizing them.
The significance of these components lies in their roles in not only storing but also managing and retrieving vast amounts of information about proteins. Here, we examine two crucial elements: data collection methods and data storage along with access protocols.
Data Collection Methods
The process of gathering protein data is systematic and multifaceted. Various methodologies are adopted to ensure high-quality, reliable, and comprehensive data entry into protein databases. Common data collection methods include:
- Experimental Techniques: This includes high-throughput methods such as mass spectrometry and X-ray crystallography, which generate primary data directly relating to protein structure and function.
- Computational Predictions: With the growth of algorithms and software tools, predictions about protein structure or functions can be derived from sequence data, expanding the scope of database information.
- Publications: Many databases curate data from existing literature. This involves manually extracting relevant data from scientific papers or utilizing automated text-mining techniques to identify new protein sequences and functional annotations.
Data collection must adhere to stringent guidelines to ensure uniformity and reliability. Incorporating standardized formats and protocols across data collection methods enhances the consistency of the information, making it more usable for researchers.
Data Storage and Access Protocols
Efficient data storage and retrieval are central to the functionality of protein databases. Key aspects include:
- Database Management Systems: Most protein databases employ relational database management systems (RDBMS) or NoSQL solutions to store structured and unstructured data. This adaptability is essential given the large volumes of protein data and the diverse data types involved.
- Indexing: Proper indexing allows for quick access to data. This enables users to efficiently search and retrieve specific information without directly scanning through the entire dataset.
- Access Protocols: Application Programming Interfaces (APIs) and web interfaces are commonly used to facilitate usersβ interaction with the database. Protocols such as REST are standard, allowing users to programmatically query databases and obtain data in real-time.
"The efficiency of data access is as crucial as the data itself; it determines the effectiveness of research outcomes."
In addition to structure and access, maintaining data integrity and security is vital. Regular updates, backups, and quality checks contribute significantly to the reliability of the database.
Overall, mastering the components of protein databases equips researchers with a thorough understanding of how best to leverage these tools for scientific inquiry.
Major Protein Databases
The topic of Major Protein Databases is vital in understanding the framework within which biological data is gathered, stored, and utilized. These databases are critical for researchers and scientists in fields like genomics, drug discovery, and structural biology. They provide centralized resources for accessing a plethora of protein-related information, which can be essential for both basic research and practical applications. Without these databases, the information regarding proteins would be fragmented, leading to inefficiencies in research and development.
UniProt: Universal Protein Resource
UniProt is a comprehensive protein sequence and functional information database. UniProt stands out because it offers a unified and multi-faceted view of protein sequences from different organisms. One key benefit of UniProt is its diverse annotations, which include functional information, post-translational modifications, and interactive browsing options.
- Accessibility: Researchers can easily access curated data, making it a go-to resource in many studies.
- Integration: The database integrates data from various sources, offering a holistic view of protein functions.
- UniRef and UniParc: These sub-databases allow users to explore redundant sequences and maintain a comprehensive dataset, which is valuable for comparative studies.
UniProtβs user-friendly interfaces and robust API make it suitable for both seasoned researchers and new students in the field.
Protein Data Bank (PDB)
The Protein Data Bank (PDB) is a crucial repository for 3D structural data of proteins. It provides invaluable information about how proteins function in a three-dimensional context. Understanding a protein's structure is essential for insights into its function, interactions, and potential as a drug target.
- Structure Analysis: PDB allows scientists to visualize protein structures, which can be critical when determining how proteins interact with ligands or other proteins.
- Resource for Drug Design: Many drug design efforts rely on PDB data to model how new compounds might fit into target proteins, aiding in the rational design of pharmaceuticals.
The seamless usability of PDB's search functionalities enables researchers to annotate and retrieve protein structures efficiently, fostering innovation across various biomedical fields.
BRENDA: The Comprehensive Enzyme Database
BRENDA focuses specifically on enzyme functionality and is among the most extensive databases in this niche. Enzymes play critical roles in biochemical pathways, and the detailed information in BRENDA is vital for understanding enzyme kinetics, structure, and function.
- Detailed Enzyme Data: BRENDA contains data on enzyme function, catalyzed reactions, and specificity, providing insights that are essential for both basic and applied research.
- Integration with Other Databases: BRENDA is connected with other databases, allowing researchers to cross-reference information about enzymes and their interactions with other biomolecules.
In summary, BRENDA offers a rich repository that serves as a starting point for anyone looking to explore enzymatic functions within biological systems.
"The structured information provided by BRENDA is crucial for advancing our understanding of metabolic pathways and enzyme function in a cellular context."
Functionality and Usability

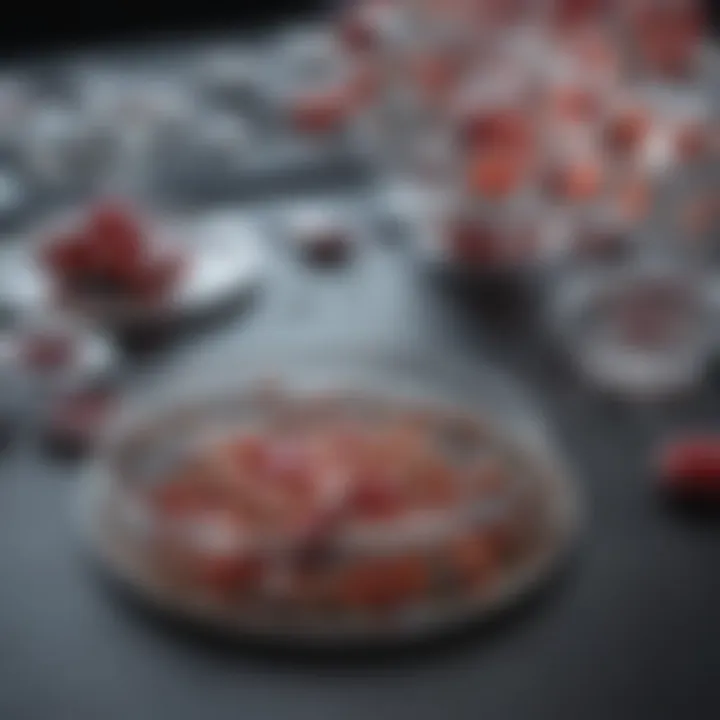
Functionality and usability are crucial aspects of protein databases. These elements determine how researchers interact with the data, the efficiency of their workflows, and the overall impact of the databases on scientific inquiry. A well-structured database can greatly enhance a userβs experience, leading to timely discoveries and fostering collaboration among scientists. In contrast, poor usability can hinder research efforts, making it difficult for users to obtain the information they need.
Search Tools and Query Mechanisms
Search tools and query mechanisms play a vital role in navigating protein databases. Researchers must quickly find relevant data to advance their studies. Databases provide various search options to facilitate this process.
- Keyword Search: This allows users to enter specific terms related to proteins, genes, or functions, yielding results based on matching records.
- Advanced Search: This offers filters and customizable criteria to refine searches based on specific needs, such as organism type, protein family, or structural details.
- Programmatic Interfaces: Application programming interfaces (APIs) allow automated data retrieval and integration within software, helping researchers efficiently update experiments or conduct complex analyses.
Properly designed search tools can significantly decrease the time spent on data collection. Users can focus on analysis and interpretation, where their expertise truly resides. Furthermore, the development of user-friendly interfaces facilitates access for non-specialists, expanding the potential user base of these databases.
Data Visualization and Analysis Tools
Visualizing data is critical for understanding complex biological information. Protein databases offer various data visualization tools that allow users to interpret and analyze protein information effectively.
- Graphical Representations: Graphical displays, such as heat maps or 3D protein structures, make it easier for users to comprehend relationships between proteins, their functions, and interactions.
- Interactive Tools: These tools enable users to manipulate data in real-time, creating a personalized experience. By adjusting parameters, researchers can better visualize how changes affect protein structure or function.
- Statistical Analysis Integration: Many databases incorporate statistical tools to analyze protein interactions or expression levels. This helps researchers identify significant patterns and correlations, supporting hypothesis generation and validation.
In summary, data visualization and analysis tools enhance usability by making complex information accessible and understandable. As protein databases continue to evolve, they must prioritize functionality, ensuring users can quickly search, analyze, and visualize protein data effectively.
"The usability of a protein database can significantly influence the speed and quality of research outcomes."
By addressing these key components, protein databases will remain relevant and continue to support advancements in biological research.
Applications of Protein Databases in Research
The role of protein databases in research cannot be overstated. These databases provide essential data that spans various disciplines such as drug discovery, genomics, and structural biology. They support scientists and researchers in their explorations by providing access to otherwise complex information. These applications showcase how protein databases enhance the quality of research and scientific inquiry.
Drug Discovery and Development
Drug discovery is a multi-faceted process, often requiring intricate knowledge of target proteins and their interactions. Protein databases play a critical role in this phase by housing vast amounts of data regarding protein structures, functions, and pathways.
- Target Identification: By accessing databases like UniProt and the Protein Data Bank, researchers can identify candidate proteins that could be targeted in drug design. This is fundamental in narrowing down potential options efficiently.
- Structure-Based Drug Design: Structural data allow scientists to visualize the three-dimensional arrangements of proteins. This is crucial for understanding how potential drug molecules might interact with the target proteins. The PDB, for instance, offers detailed structural datasets that assist in this endeavor.
- Analysing Protein-Drug Interactions: Protein databases often include information on known drug-protein interactions, which aids researchers in predicting the efficacy and safety of new compounds.
This integrative approach ensures a faster path from initial discovery to clinical trials while minimizing the risk of failure.
Genomics and Proteomics Integration
The integration of protein databases with genomic and proteomic data is vital in modern biological research. This convergence facilitates a comprehensive understanding of gene expression and the roles of proteins within cellular systems.
- Gene Annotation: Protein databases serve as repositories for functional annotations of genes. Researchers can correlate protein data with genomic sequences, thereby enhancing our understanding of gene function within biological pathways.
- Systems Biology: By linking proteomic data with protein databases, scientists can study the interactions among proteins in various cellular contexts. This approach is pivotal in identifying how proteins collaborate to execute complex biological functions.
- Data Mining: The ability to mine data from multiple databases allows researchers to draw meaningful conclusions about proteins involved in particular diseases based on genomic variations.
Integrating these fields leads to enriched findings, resulting in advancements in diagnostics and personalized medicine.
Structural Biology Applications
The significance of protein databases in structural biology is profound. They provide essential resources for researchers studying the architecture and dynamics of proteins.
- Protein Structure Analysis: Understanding a proteinβs structure is essential for grasping its function. Databases like the Protein Data Bank provide detailed structural information that serves as a basis for further research and understanding of biological mechanisms.
- Comparative Modeling: Researchers use protein databases to develop comparative models, enabling them to predict unknown protein structures based on known structures. This is particularly valuable when experimental determination of structure is challenging.
- Dynamic Studies: Some databases also include data on protein dynamics and conformational changes. These studies are crucial for understanding the functional implications of structural flexibility in proteins.
Overall, the applications of protein databases span far and wide, impacting drug development, genomic studies, and the field of structural biology. With continual advancements in data collection and analysis technologies, the relevance of protein databases will only increase in the future.
Challenges in Protein Database Management
The management of protein databases presents various challenges that can affect their functionality and the overall quality of scientific research. Understanding these challenges is crucial for researchers who rely on such databases for accurate and reliable information. Key aspects include data quality, consistency, and the integration of diverse data types, all of which play an important role in maintaining the integrity and usability of protein databases.
Data Quality and Consistency Issues
Data quality is one of the primary challenges in protein database management. It refers to the accuracy, completeness, and reliability of the data stored within these databases. Inconsistent data can lead to erroneous conclusions in research. Several factors contribute to data quality issues, including:
- Source Variation: Protein data is often obtained from different sources, and these sources may use varying methodologies for data generation. This can result in inconsistencies in the way proteins are annotated and classified.
- Errors in Data Entry: Manual data entry is susceptible to human error, which can further compromise data integrity.
- Outdated Information: As scientific understanding evolves, outdated data may persist in the databases, leading to misinformation.
To tackle such data quality issues, it is essential to implement regular updates and revisions. This might involve setting stringent guidelines for data collection and encouraging community participation in the validation of data.
Integrating Diverse Data Types
The second significant challenge encompasses the integration of diverse data types from various sources. Protein databases must handle different kinds of data, such as sequence data, structural data, and functional annotations. Each type may come from distinct disciplines within biology, making the integration complex. Key factors involved in this challenge include:
- Heterogeneity of Data Formats: Different databases may use varied formats, hindering the seamless integration of data across platforms.
- Annotation Overlap: Proteins may have multiple functional annotations that need to be reconciled. High levels of redundancy can complicate data interpretation.
- Interoperability: Linking databases so that they can communicate effectively is a critical concern.
Effective solutions to these challenges involve adopting standardized data formats and employing sophisticated data management systems. Increasing interoperability among databases is vital for providing richer and more accessible data to the scientific community.
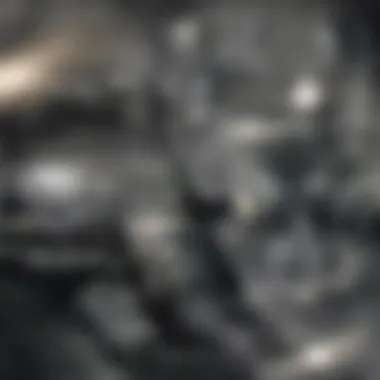
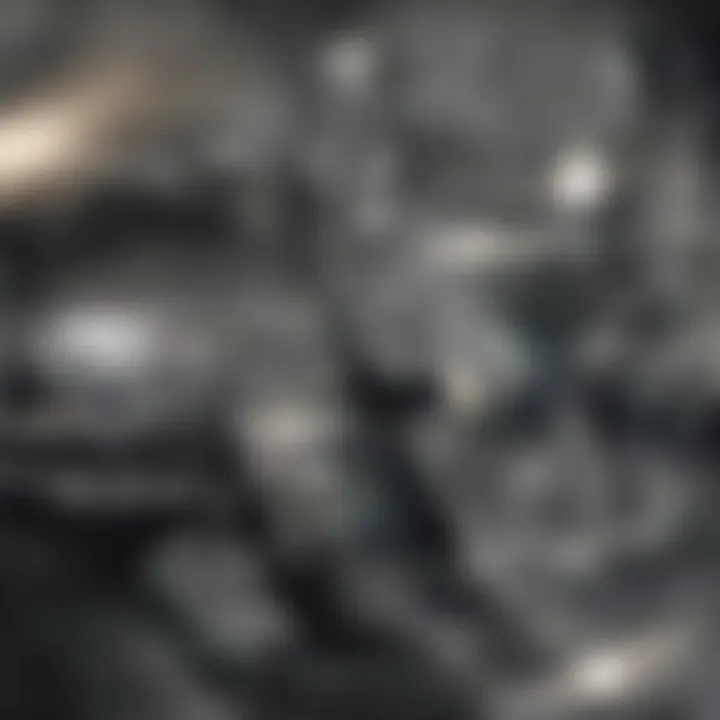
Challenges in managing protein databases are substantial but not insurmountable. By addressing data quality and integration issues, we can enhance the value of these resources in advancing biological research.
Future Directions in Protein Databases
The evolution of protein databases is an ongoing journey shaped by advancements in technology, data management, and the growing demands of the biological research community. Future directions in protein databases are crucial to enhance the scope and efficiency of these vital assets. This section highlights emerging technologies and user-centric approaches that promise to further refine the utility and accessibility of protein databases.
Emerging Technologies in Data Collection
Emerging technologies are revolutionizing how data is collected, processed, and disseminated within protein databases. Innovations, such as artificial intelligence and machine learning, are enhancing data acquisition processes, allowing for more accurate predictions of protein structures and functions.
Some of the most significant advancements include:
- Automated Data Gathering: Advances in robotics and biosensors are enabling real-time data capture from experiments. These tools can systematically generate large datasets that can be instantly integrated into databases.
- Next-Generation Sequencing: This technology has transformed genomics and proteomics by allowing rapid sequencing of DNA and RNA. As a result, more detailed and extensive protein data can be stored and accessed.
- Cloud Computing: This facilitates broader and more flexible data storage solutions, enabling collaboration and easier access for researchers worldwide. Researchers can retrieve large datasets without the traditional limitations of local storage.
- APIs and Data Interoperability: Application Programming Interfaces (APIs) enable seamless interaction between different data sources. This fosters integration across various protein databases, enhancing the holistic understanding of protein functions and relationships.
These technologies promise significant improvements in the efficiency of data collection, allowing for robust, high-quality datasets that are essential for advancing biological research.
Enhancing User Accessibility and Collaboration
Accessibility is a key factor in ensuring that protein databases serve their intended purpose effectively. As the research community continues to expand, the need for easier access to data is paramount. Enhancing user accessibility can significantly improve collaboration among researchers, educators, and students.
Key strategies for improving accessibility include:
- User-Friendly Interfaces: Simplifying interfaces can help non-expert users navigate complex databases with ease. Intuitive design promotes broader participation from various disciplines.
- Open Data Initiatives: Advocating for open access to protein data can facilitate collaboration and innovation. This encourages researchers to share their findings and insights without restrictions, fostering a conducive environment for discoveries.
- Training and Support Resources: Providing tutorials, workshops, and online resources will enable users to better utilize protein databases. Effective training can demystify complex tools and empower users to extract meaningful insights from the data.
- Community Engagement Platforms: Platforms that foster engagement between users can lead to collaborative projects that leverage shared knowledge. Discussions on platforms like Reddit can facilitate exchanges of ideas and best practices.
By making protein databases more accessible and promoting collaboration, the future landscape of biological research can be characterized by inclusivity and shared progress.
"Enhancing user accessibility and collaboration is essential for democratizing scientific research. Every researcher, regardless of expertise, should have the ability to access and contribute to protein databases."
In summary, the future directions of protein databases revolve around integrating novel technologies for efficient data collection and enhancing user accessibility. These developments not only promise to improve the quality of data but also foster collaborative research efforts that can lead to groundbreaking discoveries.
Case Studies
Case studies play a vital role in demonstrating the real-world significance of protein databases. They provide concrete examples of how these databases assist researchers in uncovering biological insights. By examining specific instances where protein databases have been utilized, we better understand their contributions to scientific knowledge and advancements.
Impact of Protein Databases on Specific Research
The impact of protein databases on specific research can be profound. For instance, researchers investigating a disease might use the Protein Data Bank (PDB) to explore the three-dimensional structures of proteins implicated in that disease. This access to structural data can lead to breakthroughs in understanding how these proteins function or malfunction.
Key benefits include:
- Accelerated Research: Researchers can quickly access comprehensive data, leading to faster experimentation and validation of hypotheses.
- Enhanced Collaboration: Data shared across various institutions fosters cross-disciplinary collaboration. This sharing enables teams to build upon one another's findings without redundancy.
- Innovative Approaches: The availability of detailed protein information inspires novel research strategies, such as targeted drug design.
Furthermore, by closely analyzing case studies, the maverage researcher can appreciate the multifaceted roles of these databases in accelerating discoveries. One example can be found in cancer research. Researchers often rely on databases like UniProt to discover potential biomarkers by identifying mutations in specific proteins linked to tumor formation.
Collaboration among Leading Research Institutions
Collaboration among leading research institutions has been critical in enhancing the functionality of protein databases. Institutions often share data, methodologies, and findings to push the boundaries of protein science.
Important considerations include:
- Resource Optimization: Sharing data prevents the duplication of efforts. Thus, institutions can focus on different aspects of research, improving overall progress.
- Standardization of Data: Collaborative efforts necessitate a standardization of data formats and protocols. This step ensures compatibility and enhances data reliability, increasing the efficacy of further research.
- Building Networks: Institutions that collaborate form extensive networks. These networks can lead to future partnerships and collective advancements in scientific goals.
A prime example can be found in the Europe PMC collaboration, where multiple research organizations contribute to a collective database. This collective effort has resulted in a richer, more diverse dataset, ultimately benefiting global research initiatives.
"Collaboration and data sharing are paramount to advancing knowledge in protein science and biotechnology."
The case studies presented show that the integration of protein databases into ongoing research not only enhances the quality of outputs but also opens avenues for new discoveries. The fusion of efforts across institutions sets a precedent for future advancements in protein research.
The End
Protein databases play a crucial role in modern biological research and applications. They serve as centralized repositories of information, providing the foundational knowledge necessary for understanding protein structure and function. The accessibility of this data is vital for researchers across various fields, including drug discovery, genomics, and proteomics. The significance of maintaining high-quality and consistent data cannot be overstated, as inaccuracies can mislead research efforts and waste valuable resources.
The Ongoing Relevance of Protein Databases
The relevance of protein databases persists as scientific inquiries evolve. Each year, new findings in molecular biology prompt updates and additions to existing databases. These updates reflect not only new protein sequences but also novel structural insights and advances in methodologies for studying protein function. As a result, databases like UniProt and PDB remain integral for researchers who rely on accurate and consistent data.
Moreover, these databases facilitate collaboration among scientists worldwide. By providing a common platform, protein databases encourage joint efforts in sharing findings and accelerating discovery. For example, researchers can freely access structural data from PDB to model interactions or predict the functions of unknown proteins.
"Protein databases are not just collections of data; they are evolving ecosystems that support scientific discovery."
Encouragement for Continued Research and Development
Continued research and development in the realm of protein databases is paramount. As our understanding of proteins deepens, so too does the complexity of the data we need to manage. This includes integrating diverse data types such as genomic, proteomic, and structural information into cohesive frameworks. With advancements in technology and bioinformatics, there offers significant potential for enhancing data collection methods and visualization tools.
Investments in machine learning could lead to improved predictive tools for protein functions, which would dramatically enhance the usability of these databases. Furthermore, as new methodologies emerge, the shift towards more user-friendly interfaces could democratize access to data, encouraging a wider range of scientists and educators to engage with protein databases.
In summary, the ongoing development of protein databases is vital for sustaining progress in biological research. Emphasizing the significance of these resources will motivate the next generation of scientists to not only utilize this information but also contribute to its continual advancement.