Advanced Insights into David RNA Sequencing Analysis
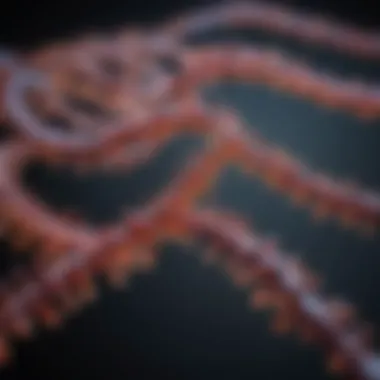
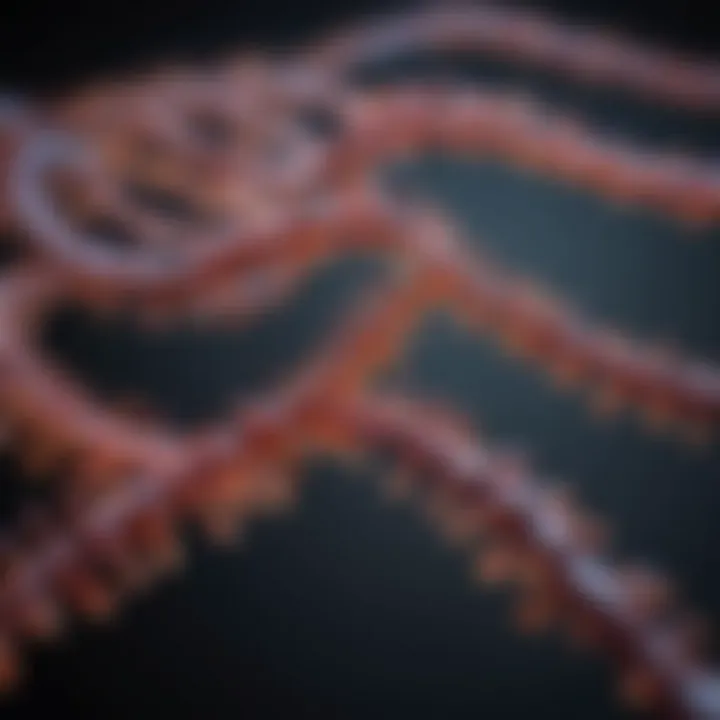
Intro
David RNA sequencing analysis stands as an integral part of genomics, emphasizing the need for deep insight into complex biological systems. This method allows researchers to comprehensively interpret gene expression data, providing clues on cellular functions and disease mechanisms. The power of RNA-seq is immense; it does not only profile ongoing transcriptional activity but also provides a pathway for innovative approaches in various fields like transcriptomics, disease research, and personalized medicine.
Research Overview
Summary of Key Findings
Recent advancements in David RNA sequencing reveal remarkable insights into gene expression patterns. Key findings highlight how this methodology can uncover the regulatory mechanisms that govern gene activities. For instance:
- Enhanced Resolution: RNA-seq has improved resolution compared to previous methods, enabling the detection of low-abundance transcripts.
- Alternative Splicing Events: The ability to identify and quantify different splice variants of genes is significantly enhanced.
- Functional Annotation: David tools efficiently annotate the genes, linking expression data to biological functions and pathways.
"Understanding gene expression through David RNA sequencing not only enhances biological comprehension but also drives forward the developments in personalized medicine."
Methodologies Employed
Several methodologies have been key to advancing David RNA sequencing analysis, including:
- Sample Preparation: High-quality RNA isolation and the use of libraries for sequencing are fundamental. Proper sample handling ensures reliable results.
- Sequencing Platforms: Utilization of platforms such as Illumina and Oxford Nanopore technology has expanded the capabilities of RNA-seq, allowing for large datasets to be generated swiftly.
- Bioinformatic Tools: The integration of David bioinformatics tools facilitates the analysis of sequencing data. Steps include normalization, differential expression analysis, and functional enrichment.
In-Depth Analysis
Detailed Examination of Results
The results obtained from David RNA sequencing often require meticulous examination. Significant shifts in expression levels between different conditions can be indicative of underlying biological processes. By employing statistical models like DESeq2 or edgeR, researchers can derive meaningful conclusions about the gene functions that may be altered in disease states.
Comparison with Previous Studies
Comparative studies shed light on the advancements in RNA-seq approaches. Earlier methodologies such as microarray analysis had limitations in sensitivity and specificity. The rise of RNA-seq, particularly with David tools, has shown a dramatic improvement in the ability to:
- Detect novel transcripts
- Analyze non-coding RNAs
- Uncover gene fusions related to certain cancers
Prelims to RNA Sequencing Analysis
RNA sequencing analysis serves as a cornerstone of modern genomics, vital for comprehending the complexity of gene expression and its implications in various biological processes. By examining RNA molecules, researchers can gain insights into cell function, differentiation, and response to environmental factors. The importance of RNA sequencing is underscored by its role in identifying novel transcripts, alternative splicing events, and gene regulation mechanisms.
The benefits of conducting RNA sequencing analysis are manifold. Firstly, it provides a comprehensive snapshot of the transcriptome in specific cellular contexts. This allows for the identification of differentially expressed genes under various conditions, contributing to our understanding of disease mechanisms and therapeutic targets.
Considerations in RNA sequencing analysis include technical aspects such as sample preparation, sequencing depth, and quality control measures. Proper handling and attention to detail in these areas are essential to ensure reliable and reproducible results. Moreover, ensuring correct data interpretation is crucial, as misinterpretations can lead to misguided conclusions in research projects.
Understanding RNA sequencing analysis is not only academically significant but also pivotal for driving innovations in personalized medicine, oncology, and infectious disease research.
In summary, the necessity of mastering RNA sequencing analysis cannot be overstated. It enables researchers and professionals to exploit genomic data effectively, leading to breakthroughs in science and medicine.
Understanding RNA Sequencing
RNA sequencing, commonly referred to as RNA-seq, involves the process of sequencing the complete RNA content of a cell. This sophisticated method allows scientists to quantify gene expression levels, identify splice variants, and discover novel transcripts. Factors such as the choice of library preparation methods, sequencing platforms, and computational techniques directly influence the quality and efficacy of RNA-seq studies.
The fundamental principle of RNA sequencing hinges on converting RNA into complementary DNA (cDNA), followed by sequencing the cDNA fragments. This procedure enables the elucidation of both coding and non-coding RNA profiles, providing a fuller picture of the transcriptomic landscape. As a result, RNA-seq offers a highly sensitive approach to measure gene expression, surpassing traditional techniques like microarrays.
Historical Context of RNA Sequencing
The evolution of RNA sequencing can be traced back to the advancements in next-generation sequencing technology, which revolutionized genomics. The first RNA-seq experiments emerged around 2008, introducing a paradigm shift in genomics research. Before this, methods for analyzing gene expression were limited, often constrained by the throughput, sensitivity, and resolution.
Early studies highlighted the robustness of RNA-seq, leading to a rapid increase in its adoption across various domains of research. Breakthroughs in sequencing chemistry and bioinformatics have continuously enhanced the capabilities of RNA-seq technologies. The historical journey has paved the way for extensive genomic analyses, moving from single-gene studies to comprehensive transcriptomic studies.
The historical lens through which RNA sequencing is viewed reveals not only its scientific significance but also its growing impact on personalized medicine and therapy design, influencing treatment plans based on unique genomic profiles.
Overview of the David Bioinformatics Tool
David is a robust bioinformatics tool designed for the analysis of large-scale genomic data. Its primary aim is to provide comprehensive insights into gene function and how genes interact within biological systems. The advent of RNA sequencing has made vast amounts of data available to researchers, increasing the need for efficient analysis tools that can offer clarity amidst the complexity.
Understanding the David tool's role in RNA sequencing analysis is crucial because it bridges the gap between raw data and meaningful biological interpretation. With the increasing focus on personalized medicine and targeted therapies, the ability to decipher gene expression patterns has never been more significant.
David integrates multiple analytical approaches, allowing researchers to carry out functional annotation, enrichment analysis, and graphical visualization of results. This versatility enables scientists to tackle a variety of biological questions and challenges using RNA-seq data.
Prelims to the David Tool
The Database for Annotation, Visualization, and Integrated Discovery, commonly referred to as David, is an online bioinformatics resource. It assembles numerous tools that assist in interpreting large volumes of gene expression data resulting from RNA sequencing. This tool is tailored for researchers who require more than mere lists of genes; it provides biological context and function to the data.
Using David, one can upload gene lists and employ various analyses that pinpoint key biological insights. The tool employs an intuitive interface, helping to facilitate ease of use for those who may not be experts in bioinformatics. The interdisciplinary design ensures that it can be beneficial for students, educators, and researchers alike.
Key Features of David
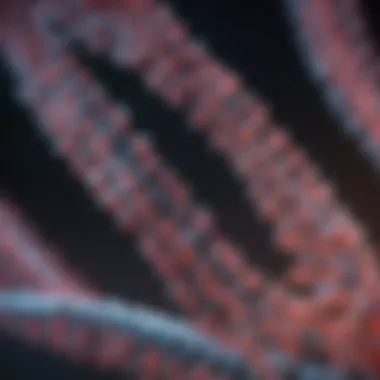
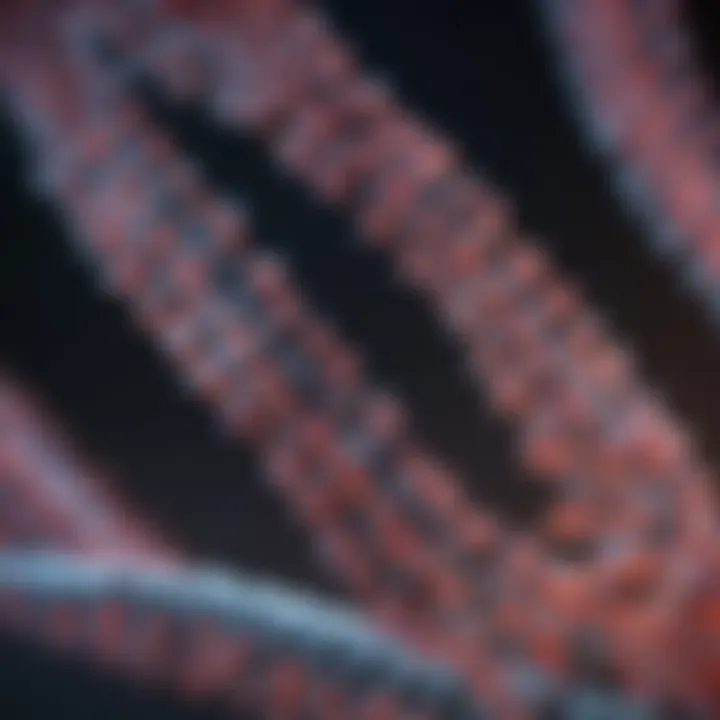
David boasts several features that underpin its utility in RNA sequencing analysis:
- Functional Annotation: Users can receive detailed information about gene function, including gene ontology and biochemical pathway involvement.
- Enrichment Analysis: This allows researchers to determine if certain biological functions are overrepresented in their gene list, which can reveal significant trends in gene expression.
- Visualization Tools: Graphical outputs such as charts and graphs assist in interpreting and presenting data clearly.
- Customizable Options: The platform enables users to customize their analysis based on their specific research needs, enhancing its applicability across various studies.
"With David, researchers can turn extensive RNA-seq data into structured, interpretable insights that illuminate gene function and interactions."
These key features make David an invaluable resource in genomic research, particularly when it comes to unraveling the complexities of RNA sequencing data.
RNA Sequencing Methodologies
RNA sequencing methodologies serve a crucial role in the field of genomics by providing a pathway to understanding gene expression and functional genomics. These methodologies enable researchers to analyze complex RNA data, unveiling intricate biological processes. Key elements include effective library preparation, sequencing platforms, and data analysis protocols. Each component is essential to ensure accurate results and enhance the reliability of findings derived from RNA sequencing.
Library Preparation Techniques
Library preparation is a foundational step in RNA sequencing. It involves converting RNA into complementary DNA, allowing for the sequencing of genetic information. This process is vital because it influences the quality and accuracy of sequencing results.
Protocols for cDNA Synthesis
Protocols for cDNA synthesis cover various techniques used to convert RNA into complementary DNA. These protocols typically involve reverse transcription, where the enzyme reverse transcriptase synthesizes cDNA from RNA templates. The key characteristic of these protocols is simplicity, making them accessible for both small-scale research and large-scale genomics.
An important aspect of cDNA synthesis is the choice of primers, which can be random hexamers or oligo(dT). Random hexamers provide a more unbiased representation of the RNA population, while oligo(dT) primers focus on polyadenylated mRNAs.
Advantages of these protocols include high sensitivity for detecting low-abundance transcripts and compatibility with various RNA types. However, they may also come with some disadvantages, such as potential biases in transcript representation depending on the chosen primers.
Amplification Strategies
After cDNA synthesis, amplification strategies are necessary to increase the quantity of cDNA for sequencing. These strategies often employ polymerase chain reaction (PCR) techniques. The key characteristic of amplification strategies is their ability to produce sufficient quantities of genetic material for downstream applications.
One commonly used amplification method is PCR, which selectively amplifies specific cDNA regions. This method is beneficial as it can greatly enhance the amount of synthesized product, enabling detection of rare transcripts. However, the main disadvantage is that it may introduce biases, especially if amplification is not carefully controlled. Over-amplification can skew the representation of specific transcripts in the resulting library.
Sequencing Platforms
RNA sequencing methodologies strictly depend on the sequencing platforms employed. Different platforms can yield varying read lengths, throughput, and error rates. Understanding these distinctions is important when selecting a suitable platform for a given project.
Next-Generation Sequencing
Next-generation sequencing (NGS) revolutionized the field of genomics by allowing high-throughput sequencing. NGS technologies enable researchers to sequence millions of fragments simultaneously, drastically reducing the time and cost associated with sequencing.
The key characteristics of NGS techniques include their speed and scalability. This technology allows for vast quantities of data to be generated from a single run. In RNA sequencing, NGS can provide deeper insight into transcriptomic landscapes, uncovering nuances in gene expression.
However, while NGS is a popular choice, it also presents challenges, such as the requirement for extensive bioinformatics expertise to analyze the data produced. Moreover, the initial setup cost may deter smaller laboratories.
Comparative Analysis of Platforms
Conducting a comparative analysis of sequencing platforms is essential for making informed choices. Factors to consider include accuracy, read length, throughput, and cost-effectiveness. By evaluating these factors, researchers can better align their platform choice with their specific project needs and available resources.
The key aspect of comparative analysis is its capacity to highlight the strengths and weaknesses of each platform. For example, while Illumina sequencing offers high throughput and accuracy, technologies like Oxford Nanopore provide long-read capabilities, which can be beneficial for resolving complex genomic regions.
Ultimately, the decision on which platform to use hinges on the scientific objectives and resources available. Understanding the unique features, advantages, and limitations of each technology allows researchers to optimize their RNA sequencing studies, ensuring reliable results that drive biological insight.
"Proper methodology is the cornerstone of successful RNA sequencing analysis, allowing researchers to decode the complexities of gene expression."
Adhering to well-established methodologies in RNA sequencing is critical for producing reproducible and meaningful results in biological research.
Data Processing in RNA Sequencing
Data processing in RNA sequencing is critical for obtaining reliable and meaningful insights from sequencing data. This stage includes various steps that ensure the quality of the data before further analysis. It not only improves the accuracy of results but also helps in identifying biological significance in the raw data. Given the complexity of RNA-seq datasets, robust data processing techniques are essential. Each step in this process must be performed with careful consideration to reduce noise and enhance signal detection.
Quality Control Measures
Quality control measures are the backbone of RNA-seq analysis. They consist of a series of checks aimed at assessing the quality of sequencing reads. Without proper quality assessment, subsequent analysis may lead to misleading conclusions.
Assessing Read Quality
Assessing read quality entails evaluating the raw sequencing data to determine overall accuracy. Tools like FastQC are commonly used to visualize key metrics such as the distribution of base qualities across reads. A primary characteristic of read quality assessment is its ability to identify sequencer errors that can impact downstream analysis. High-quality reads with minimal errors are essential for accurate gene expression measurement and biological interpretation. This practice is beneficial because it helps researchers make informed decisions when filtering out low-quality reads. However, relying solely on numerical metrics can be limiting, as it may not convey biological relevance effectively.
Trimming Techniques
Trimming techniques aim to remove low-quality bases from the ends of sequencing reads. This step is crucial for enhancing the integrity of the remaining data. Trimming improves overall data quality by ensuring that only high-quality bases are considered in analysis. A popular choice for trimming is the Trimmomatic software, which provides flexible trimming options based on quality scores. The unique feature of trimming techniques lies in their ability to optimize read length and quality, significantly benefiting downstream applications like alignment. However, if trimming is excessively aggressive, it can lead to loss of valuable information.
Alignment Algorithms
Alignment algorithms are fundamental in mapping sequencing reads to a reference genome. This step is integral as it determines how reads correlate with known genes or transcripts. Effective alignment is crucial for making sense of gene expression data.
Preamble to Alignment Metrics
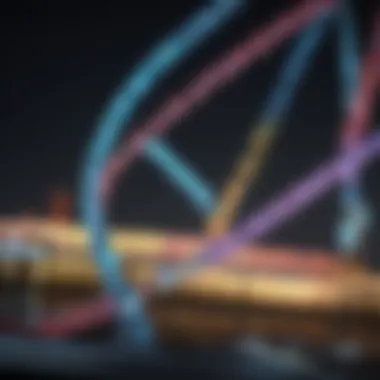
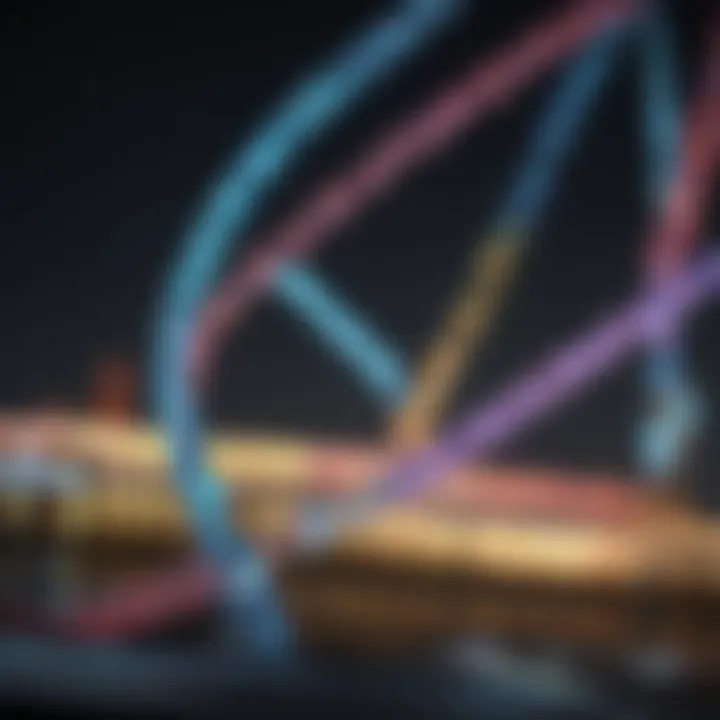
Introduction to alignment metrics covers how the performance of alignment algorithms is evaluated using various statistical measures. Metrics like alignment rate and error rate guide researchers in assessing the reliability of the alignment. A crucial characteristic of alignment metrics is their role in identifying how well reads match to a reference sequence, which significantly influences downstream analysis. These metrics are beneficial as they provide quantitative assessments that help refine alignment strategies. However, they can sometimes lead to false confidence in results if the underlying assumptions do not hold for the data being processed.
Challenges in Alignment
Challenges in alignment often arise from biological variability, sequencing errors, and limitations of the algorithms themselves. Variability in gene expression can complicate the mapping of reads, particularly in complex regions of the genome. A key characteristic of these challenges is their contribution to systematic biases that can affect data integrity. Addressing these issues is vital for achieving accurate alignment and, therefore, meaningful insights. While thorough alignment strategies can mitigate some challenges, it is essential to recognize that no algorithm is flawless. This reality requires continuous refinement of methods to adapt to emerging complexities in RNA-seq analysis.
Key Takeaway: Effective data processing through quality control measures and robust alignment algorithms is indispensable in ensuring the reliability of RNA sequencing data, ultimately facilitating accurate biological interpretation.
Analysis of RNA-Seq Data
The analysis of RNA-Seq data is a cornerstone of modern genomic studies. This process allows researchers to delve into complex gene expression patterns, providing insights that can translate into significant biological discoveries. By implementing rigorous methods and utilizing specialized tools like the David Bioinformatics tool, scientists can effectively harness the wealth of information generated through RNA sequencing.
Differential Gene Expression Analysis
Statistical Methods Used
Statistical methods play a crucial role in differential gene expression analysis. They help identify genes that show significant changes in expression between different conditions, such as healthy and diseased states. Popular methods include DESeq2 and edgeR, known for their robustness and sensitivity in handling RNA-Seq data.
The key characteristic of these statistical methods is their ability to account for various sources of variability, including differences in sequencing depth and biological variance among samples. This makes them valuable for accurately determining which genes are truly differentially expressed, as opposed to those with fluctuating signals due to noise.
A unique feature of these methods is their reliance on negative binomial distributions, which effectively model count data inherent in RNA-Seq. This approach offers a distinct advantage in producing reliable results, although it can become complex in datasets with low counts or high variability among samples.
Interpreting Results
Interpreting results from differential gene expression analysis offers insights into biological processes. By examining the list of differentially expressed genes, researchers can draw conclusions about underlying biological mechanisms or disease processes. The identification of key genes and their functional roles can lead to targeted research for therapeutic interventions.
The key characteristic of result interpretation lies in the integration of gene expression data with available biological knowledge. This integration allows for mapping gene expression to pathways and cellular functions, making the analysis highly relevant. However, a notable challenge is the risk of overinterpretation, where researchers may make claims based on statistically significant findings without robust biological validation.
Functional Annotation of Genes
Using David for Functional Insights
The David Bioinformatics tool provides essential support for understanding gene functions and their biological implications. By linking genes to known functions, David facilitates deeper insights into the roles of differentially expressed genes. This aspect is critical for discerning the biological relevance of gene expression changes observed in RNA-Seq data.
A key characteristic of using David is its comprehensive databases, including gene ontology and pathway information. This resource is favored because it pools vast amounts of data, enabling quick access to functional annotations across many organisms. A unique feature of David is its ability to generate functional clustering results, summarizing information and highlighting important biological themes.
However, there can be limitations, such as dependency on existing knowledge bases. If a gene is poorly characterized or absent from databases, functional insights may be limited.
Pathway Enrichment Analysis
Pathway enrichment analysis assesses whether differentially expressed genes are significantly represented in particular biological pathways. This process is critical for comprehending the broader implications of gene expression changes and linking these alterations to specific cellular processes.
The key characteristic of pathway enrichment analysis is the statistical methods it employs to evaluate the significance of observed overlaps between gene lists and predefined pathways. This aspect renders the approach popular, as it provides contextual meanings to gene expression data. A unique feature is the enrichment score, which quantifies the degree of association between gene sets and pathways, offering insight into their biological importance.
Despite its advantages, pathway enrichment analysis can present challenges. Results are heavily influenced by the choice of gene ontology categories or pathways considered, which may introduce biases. Thus, careful selection and interpretation of results are paramount to avoid misleading conclusions.
Applications of RNA Sequencing
RNA sequencing has transformed the fields of genomics and molecular biology. Its capacity to provide insights into gene expression patterns has profound implications for diverse research areas, making its applications far-reaching and impactful. This section will explore notable areas where RNA sequencing has made significant contributions, including transcriptomic studies, clinical applications in disease research, and the development of personalized medicine approaches.
Transcriptomic Studies
Transcriptomic studies rely heavily on RNA sequencing to provide an extensive view of the transcriptome. By analyzing the complete set of RNA molecules present in a biological sample, researchers can identify gene expression levels and discover novel transcripts. This method offers clarity into cellular activities, revealing how genes are regulated in different conditions.
Key benefits of RNA-seq in transcriptomic studies include:
- High sensitivity, allowing the detection of low-abundance transcripts.
- The ability to quantify RNA levels across a wide dynamic range.
- Capability to discover novel non-coding RNAs and alternative splicing events.
These advantages make RNA sequencing an essential tool for researchers seeking to understand gene regulation and function in various biological contexts.
Clinical Applications in Disease Research
RNA sequencing applications extend into clinical research, particularly in understanding disease mechanisms. Through precise gene expression analysis, scientists and clinicians can uncover molecular changes associated with various diseases. This insights can lead to new diagnostics and targeted therapies.
Oncology Research Applications
In oncology, RNA sequencing is invaluable for identifying biomarkers associated with tumor types. By comparing the expression profiles of cancerous and normal tissues, researchers can uncover unique signatures that facilitate more accurate diagnoses and treatment plans. One main characteristic of RNA-seq in oncology is its ability to capture the heterogeneity of tumors, which is crucial since cancers can vary significantly even among individuals with the same diagnosis.
The unique feature of this application is its potential for personalized treatment strategies. For instance, if a tumor expresses a particular gene that responds to a specific drug, clinicians can tailor therapy to that unique profile, improving outcomes. However, challenges exist, such as the need for comprehensive datasets and complex bioinformatics analyses to interpret results accurately.
Research in Genetic Disorders
In the realm of genetic disorders, RNA sequencing allows researchers to identify mutations that disrupt normal gene expression patterns. This application has proven beneficial in understanding rare diseases. One highlight of RNA-seq in genetic research is its ability to elucidate the functional consequences of genetic variants.
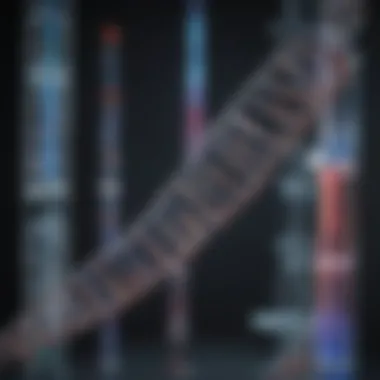
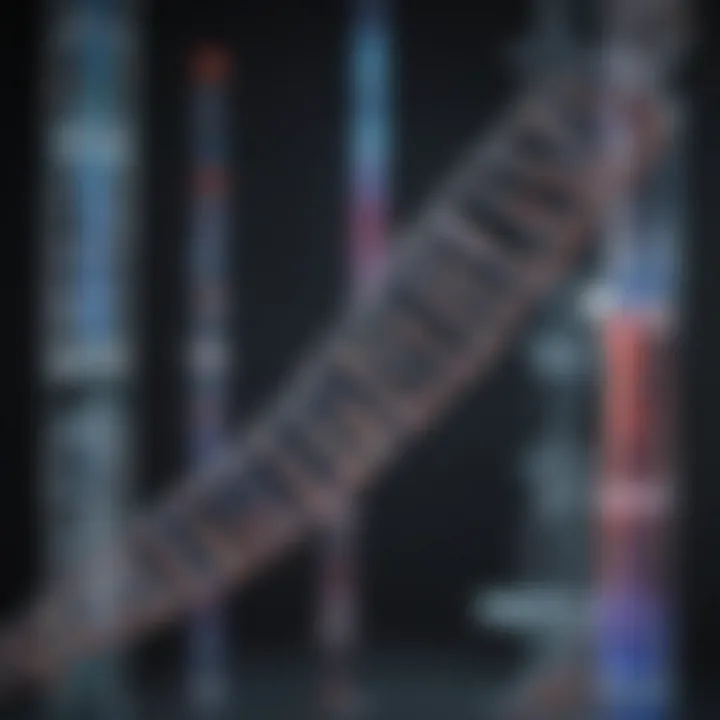
The unique feature of utilizing RNA sequencing here can uncover insights into gene functions and pathways affected by genetic mutations. Nevertheless, the high costs and technical expertise required for data interpretation might present challenges for broader adoption in routine clinical practice.
Personalized Medicine Approaches
The approach of personalized medicine has been revolutionized by RNA sequencing. By tailoring healthcare based on individual genetic profiles, clinicians can devise specific treatment plans that enhance efficacy and reduce side effects. RNA-seq plays a crucial role in identifying potential therapeutic targets that are unique to each patient.
Implementing RNA-Seq in Clinical Settings
When implementing RNA sequencing in clinical settings, the focus is on determining actionable data from genetic information. This implementation aims to integrate RNA-seq findings into standard care practices. One of its key attributes is the facilitation of understanding complex diseases by providing a comprehensive view of gene expression changes.
A notable advantage of this implementation is that it allows real-time adjustments to treatment based on a patient's ongoing response. It can, however, be limited by the lack of standardized protocols and variations in sequencing technologies, which may complicate cross-institution comparisons.
Evaluating Patient-Specific Therapies
Evaluating patient-specific therapies is another pivotal aspect of RNA sequencing applications. This entails analyzing an individual’s RNA profile to inform treatment choices uniquely suited for them. The main characteristic of this application is its potential to enhance therapeutic effectiveness by focusing on biomarkers unique to the patient’s tumor. This is particularly beneficial for oncology, where understanding a patient’s unique cancer profile can guide drug selection.
On the downside, not all therapeutic responses can be predicted accurately by RNA profiling alone. There are limitations, including varying responses among patients and the complex biological interactions influencing these outcomes.
In summary, the applications of RNA sequencing are extensive and impactful across various fields. From gaining insights into transcriptomics to supporting clinical applications in disease understanding and personalized medicine, RNA-seq continues to play a critical role in shaping modern genomics.
Challenges in RNA Sequencing Data Analysis
The process of RNA sequencing presents significant opportunities for researchers. However, alongside these opportunities come various challenges that need to be addressed to ensure reliable and valid results. Understanding these challenges is crucial for improving methodologies, developing better bioinformatic tools, and ultimately enhancing the interpretation of complex biological data. This section discusses the major challenges in RNA sequencing: technical limitations and biological variability.
Technical Limitations
Technical limitations are a prominent concern in RNA sequencing analysis. These limitations can arise from various stages of the sequencing workflow, including sample preparation, sequencing platform choice, and data processing methods. The following points are vital to consider:
- Sample Quality: RNA quality can greatly affect sequencing outcomes. Degraded RNA leads to poor libraries and subsequently confounding results.
- Library Preparation: Different protocols can introduce biases, especially when amplifying cDNA. Consistency in library preparation minimizes variability in results.
- Sequencing Depth: Insufficient read depth can limit the ability to detect lowly expressed genes, while excessive depth may increase costs without significant gains in data quality.
- Platform-Dependent Variability: Different sequencing platforms, such as Illumina and Oxford Nanopore, may yield variable accuracy and performance characteristics.
Addressing these technical limitations requires careful experimental design and selection of appropriate technologies. Researchers must prioritize quality control throughout the process to mitigate potential issues that could affect downstream analyses.
Biological Variability
Biological variability poses another layer of complexity in RNA sequencing analysis. Variability arises from numerous sources in biological systems, necessitating thorough consideration during data interpretation:
- Intrinisic Biological Differences: Variability in gene expression can stem from natural differences between individuals, tissues, or even within a single tissue over time. Understanding these differences is key for accurate comparison and analysis.
- Environmental Influence: Factors such as diet, temperature, and stress can impact gene expression levels. These external influences can result in variations that complicate the analysis and interpretation phases.
- Experimental Conditions: Differences in handling, processing, or storage conditions can further introduce biological variability, which needs to be accounted for in experimental designs.
While technical and biological variability pose challenges, embracing these obstacles can lead to enhanced methodologies and more robust results. Recognizing sources of variability allows researchers to interpret their findings with greater accuracy and can inform the development of more sophisticated analytical approaches.
Future Perspectives in RNA Sequencing
The future of RNA sequencing analysis is promising, with many potential advancements on the horizon. This section will cover significant trends shaping the field. Understanding these developments is crucial for researchers and professionals investing in RNA-seq technologies.
Advancements in Sequencing Technologies
The progress in sequencing technologies plays a pivotal role in enhancing the capabilities of RNA sequencing.
- Single-Cell RNA Sequencing: Recent methods have focused on the analysis of RNA from individual cells. This technique allows researchers to investigate heterogeneity within tissues, providing clearer insights into cellular functions and gene expression patterns.
- Long-Read Sequencing: Traditional short-read sequencing often presents challenges in resolving complex genomic regions. New approaches, such as those used by Pacific Biosciences and Oxford Nanopore Technologies, offer the ability to generate longer reads. This advancement facilitates better assembly of transcripts and enhances the understanding of transcript variants.
- Integration with Other Omics Technologies: Combining RNA sequencing with proteomics and metabolomics is becoming more common. This multi-omics approach enables a more holistic view of biological processes, promoting a deeper comprehension of cellular responses.
As technology evolves, these advancements will likely transform future research methodologies, allowing for greater precision and depth of understanding in RNA-seq.
Evolving Approaches in Analysis Methods
In addition to sequencing advancements, the methods for analyzing RNA-seq data continue to evolve.
- Machine Learning Applications: The use of machine learning in RNA data interpretation is gaining traction. Leveraging algorithms helps decode patterns in large datasets, leading to more refined predictions and insights into gene functionality.
- Improved Statistical Frameworks: New statistical models are being developed to address complexities in RNA-seq data. These models enhance the reliability of differential expression analysis and reduce false discovery rates.
- User-Friendly Bioinformatics Tools: As the demand for RNA analysis rises, user-friendly platforms are becoming increasingly important. Tools that simplify the analysis process and provide intuitive interfaces are essential for facilitating widespread adoption.
"The convergence of advanced technologies and sophisticated analysis methods will define the next era of RNA sequencing research."
These evolving methodologies represent a critical shift toward more sophisticated analyses, ultimately enabling a better understanding of the biological implications of gene expression.
The End
The conclusion section of any article is essential as it brings together all the key themes discussed throughout the text. In the case of this article on RNA sequencing analysis via the David tool, it serves to reinforce the significance of the techniques, methodologies, and applications explored.
Summarizing Key Findings
The findings discussed underscore the importance of RNA sequencing in elucidating gene expression patterns. This is paramount in contemporary biology, as it not only helps in understanding normal physiological processes but also sheds light on pathological states. Through tools like David, researchers have improved capabilities in interpreting vast datasets. The integration of advanced analytical methods allows for more precise functional annotation. Key aspects to remember include:
- The role of library preparation in ensuring data quality.
- Advanced statistical methods that enhance the robustness of differential expression analyses.
- Functional analysis offering insights into biological pathways impacted by gene expression changes.
Collectively, these elements reflect how David RNA sequencing analysis enhances our understanding of genomics.
The Ongoing Importance of RNA-Seq
As the landscape of genomics continues to evolve, RNA sequencing maintains a crucial position. Its applicability extends far beyond basic research, now embracing clinical and translational research as well. With the advent of personalized medicine, RNA-Seq is positioned to play an integral role in developing tailor-made therapies, particularly in oncology and genetic disorders. Researchers must consider:
- The continuous advancement of sequencing technologies, which leads to higher throughput and reduced costs.
- The integration of RNA-Seq data with other omics data for a more comprehensive understanding of biological systems.
- Ethical considerations surrounding genomic data usage and patient confidentiality.
Ultimately, the ongoing importance of RNA-Seq lies in its potential to transform not just our understanding of biology but also its applications in medicine and therapeutics, making it a focal point in genomics research.