AI and Protein Folding: A Revolutionary Approach
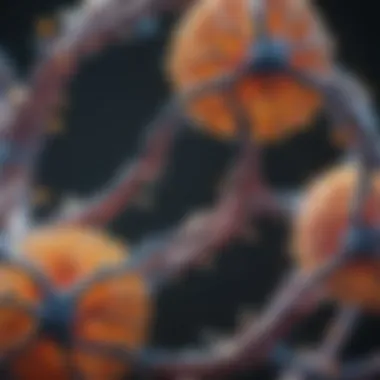
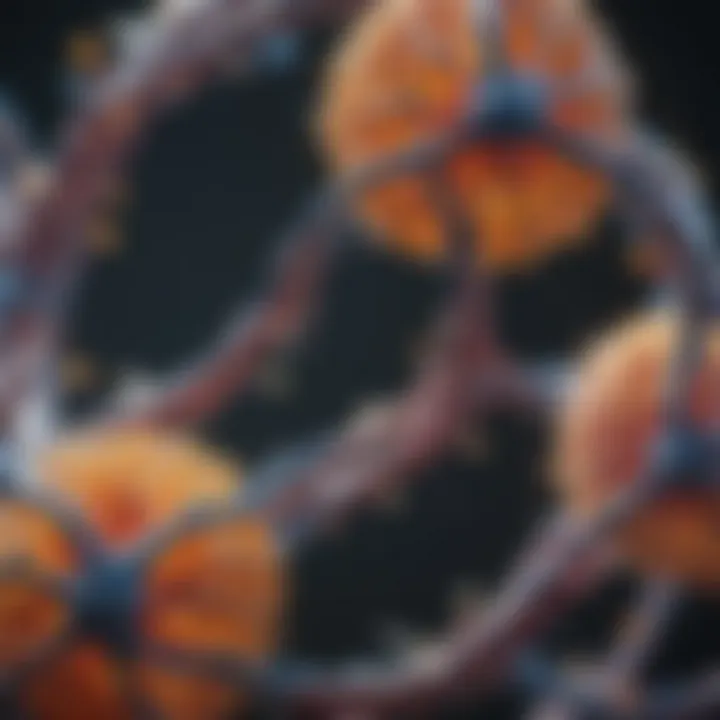
Intro
In recent years, the relationship between artificial intelligence and protein folding has captured the attention of researchers, educators, and professionals alike. Protein folding is a process that is critical to the function of proteins, which are essential to every cell in the body. By understanding how these molecules attain their three-dimensional shape, scientists can unlock vital information about biological processes.
Artificial intelligence, on the other hand, provides sophisticated tools that can analyze vast amounts of data much faster and more accurately than traditional methods. This intersection of AI and protein folding has the potential to accelerate drug discovery, enhance our understanding of diseases, and ultimately, improve medical treatment.
As we explore this topic, we will delve into the foundational elements of protein folding, the technological advancements in AI that aid in this area, and the implications for research and clinical applications.
Research Overview
Summary of Key Findings
The integration of AI in protein folding research has led to several key findings:
- Predictive Accuracy: AI algorithms now demonstrate increased accuracy in predicting protein structures, often outperforming conventional methods.
- Speed of Analysis: AI can process and evaluate data at a pace that significantly reduces the time needed to derive meaningful insights from protein folding studies.
- Diverse Applications: Results from these advancements are seen across various fields, including drug development, vaccine design, and understanding genetic disorders.
The prominence of AI-driven platforms, such as AlphaFold, has transformed how scientists predict protein structures.
Methodologies Employed
Various methodologies are employed in this research area, including:
- Machine Learning: Algorithms used for pattern recognition in vast biological datasets.
- Deep Learning: Neural network models that learn complex representations for better accuracy.
- Generative Models: These simulate processes to predict folding patterns based on existing data.
With these methodologies, researchers can glean insights that were previously unattainable.
In-Depth Analysis
Detailed Examination of Results
Recent studies illustrate that AI can minimize errors in protein structure prediction. A significant example of this is seen in the application of deep learning models, which have shown improved precision in folding predictions compared to traditional approaches. Researchers now seek to validate these findings further in biological systems.
Comparison with Previous Studies
Looking back at earlier studies in protein folding reveals a stark contrast to current methodologies. Techniques relied heavily on experimental data, often resulting in lengthy processes that offered limited scope. However, the innovations brought by AI indicate a paradigm shift in how this research is conducted, yielding faster and more reliable outcomes.
In summary, the advent of AI technologies heralds a new era in the domain of protein folding, unlocking opportunities for significant advancements in biological research.
Intro to Protein Folding
Protein folding is a critical process that dictates the three-dimensional shape of proteins, which in turn influences their function in biological organisms. The folding process is essential; a properly folded protein can perform its specific tasks, while misfolded proteins can lead to various diseases. Understanding this process is crucial for fields such as biochemistry, molecular biology, and pharmacology.
Definition of Protein Folding
Protein folding refers to the physical process by which a protein chain acquires its native 3D structure from a randomly coiled polypeptide chain. This process involves a complex series of interactions, including hydrogen bonding, ionic interactions, and hydrophobic effects among the amino acids in the protein. The final structure is usually unique to a particular protein and enables it to perform its biological role effectively.
Importance in Biological Systems
Protein folding is paramount in biological systems. Each protein's specific structure determines its function within the cell. For example, enzymes rely on their unique shapes to catalyze biochemical reactions efficiently. Misfolding can lead to loss of function or toxic gain of function, contributing to illnesses such as Alzheimer's or cystic fibrosis. Therefore, understanding protein folding can provide insights into normal biological processes and potential therapeutic interventions.
Challenges in Protein Folding Studies
There are several challenges in studying protein folding. First, the sheer number of possible configurations for a protein makes it difficult to predict which conformation it will adopt. This is known as the folding problem. Furthermore, many proteins do not fold in isolation but interact with other molecules, complicating the predictions. Finally, experimental methods to determine structures, like X-ray crystallography and NMR spectroscopy, can be time-consuming and costly, creating a barrier to understanding protein folding on a broader scale.
"Understanding protein folding can unveil crucial therapeutic targets in disease treatment and prevention."

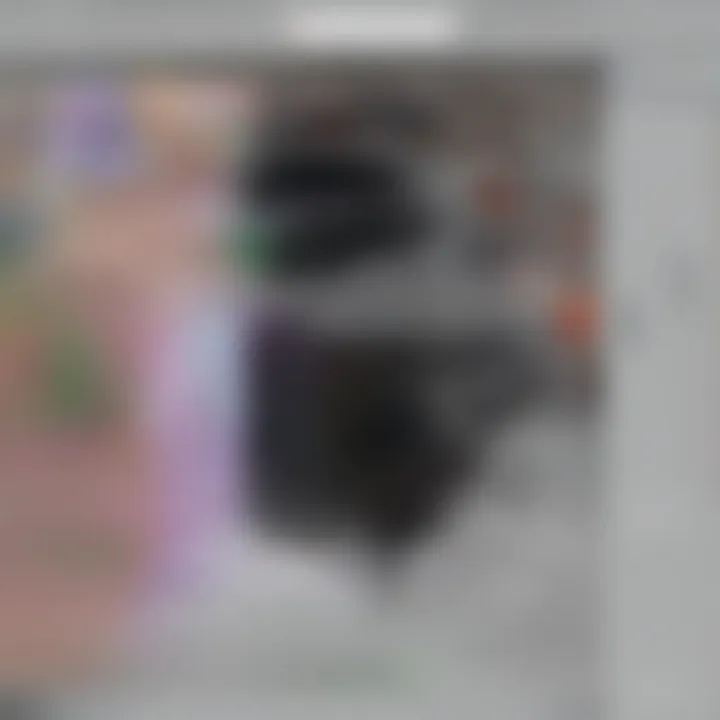
In summary, protein folding is an intersection of chemistry, biology, and physics. The intricacies involved in this process are essential for maintaining life. With the advent of technologies like artificial intelligence, researchers are beginning to unravel these complexities, paving the way for breakthroughs in drug discovery and medical research.
Overview of Artificial Intelligence
Artificial Intelligence is a critical topic within the context of this article. The relevance of AI in protein folding stems from its capacity to enhance predictions related to protein structure and dynamics. The juxtaposition of AI technologies and biological studies offers a transformative lens through which research can be conducted, leading to more accurate models and insights. This section serves to build a foundation for understanding how AI contributes to significant advancements in the field of molecular biology.
AI Defined
Artificial Intelligence refers to the capability of machines to mimic cognitive functions typically associated with human minds, such as learning, reasoning, problem-solving, and understanding language. In the context of protein folding, AI utilizes algorithms to analyze vast amounts of biological data. These algorithms can identify patterns within protein structures that would be difficult for researchers to discern manually. A crucial aspect to note is that AI does not replace human expertise; rather, it acts as an amplifying tool that drives innovative discoveries.
Historical Context of AI
The history of AI traces back to the mid-20th century, with early explorations in computational models and symbolic reasoning. Initially, AI focused on rule-based systems, which limited its effectiveness. However, significant breakthroughs emerged in the 1980s with the development of machine learning techniques. These approaches allow programs to learn from data inputs and improve their predictions over time. By the 2010s, the rise of deep learning redefined AI, enabling it to handle increasingly complex datasets, particularly in research like protein folding where massive amounts of data exist. Understanding this evolution is key to appreciating the current state and potential of AI in biological research.
Key Terminologies in AI
Grasping the core terminologies associated with AI is essential for any meaningful discussion in this field. Here are some key terms:
- Machine Learning: A subset of AI that allows systems to learn from data and improve without being explicitly programmed.
- Deep Learning: A specialized method of machine learning that uses neural networks with many layers, helping to uncover intricate structures in data.
- Neural Networks: Algorithms inspired by the human brain that are used to recognize patterns and make decisions based on data input.
AI also involves terms like "natural language processing" and "computer vision," each playing unique roles within different branches of AI research. Familiarity with these concepts will aid in understanding AIโs application in protein folding studies and how it carries significant implications for the future of biological sciences.
"Artificial Intelligence is a reflection of human intellect, structured to extend our capabilities in processing biological complexities."
This foundational overview of AI sets the stage for delving into its specific roles in protein folding. It equips the reader with the necessary vocabulary and historical understanding to appreciate the implications that AI holds in this field.
The Role of AI in Protein Folding
Artificial Intelligence is becoming a pivotal force in the field of protein folding. Proteins are essential macromolecules that perform a myriad of functions in biological systems. Understanding their structure and folding mechanisms is critical for comprehending how they operate. AI is enhancing our ability to predict and analyze protein structures, making it easier to explore complex biological processes.
By utilizing AI, researchers gain systematic tools that assist in understanding and simulating the intricate pathways of protein folding. This involvement of AI leads to more accurate predictions, which can, in turn, aid various branches of biological research. AI brings consistency, speed, and precision to protein analysis that manual methods simply cannot match.
Mechanisms of Protein Folding Assistance
AI contributes to protein folding assistance in several ways. Firstly, machine learning algorithms allow for the analysis of vast datasets generated by biological experiments. These algorithms can identify patterns that might remain hidden in manual analysis. For instance, AI can be trained on known protein structures to develop models that predict how new proteins will fold based on their amino acid sequences.
Furthermore, advances like AlphaFold have showcased AIโs capability to predict protein structures with remarkable accuracy. By using deep learning techniques, AlphaFold demonstrates the power of AI to tackle classic biological challenges. This mapping of sequence to structure is invaluable, especially for proteins that are difficult to study experimentally.
AI in Predictive Modeling
Predictive modeling is another crucial area where AI plays a significant role in protein folding. With tools like neural networks, researchers can simulate various folding conditions and predict outcomes effectively. The models help understand not just the static structures of proteins, but also their dynamic states during folding.
AI-driven predictive modeling can generate hypotheses for experimental validation. This process streamlines research efforts since focused searches can be made based on AI predictions. The implications for drug discovery are profound, as models can anticipate the interactions of potential drug molecules with protein targets precisely. The insights gained from predictive modeling enhance our understanding of protein behavior in various biological contexts.
Limitations of Current AI Tools
Despite the promise of AI in protein folding, several limitations exist that warrant discussion. Most AI tools require substantial amounts of data to train effectively, and not all proteins have sufficient data for accurate predictions. Additionally, while algorithms can provide predictions, they often lack transparency. The 'black box' nature of some AI models makes it challenging for researchers to understand the underlying reasoning for predictions.
Moreover, AI models must be continuously validated against experimental data. This validation process is crucial, as inaccuracies can lead to misguided research directions. Researchers should be cautious not to over-rely on AI predictions without corroborating evidence from laboratory studies. Addressing these limitations will help solidify the role of AI in protein folding research.
In summary, AI's role in protein folding represents a transformation in biological research methods. The ongoing advancements will likely refine our understanding of protein structures and their functionalities, thus advancing fields like drug discovery and disease treatment.
Deep Learning Techniques in Protein Folding
Deep learning is a pivotal factor in advancing the study of protein folding. This compound area combines elements of artificial intelligence and systems biology. Deep learning leverages large datasets to discern intricate patterns that may escape conventional analytical approaches. In the realm of protein folding, where predicting structure is vital, deep learning is revolutionizing methods for model building and understanding biological function.
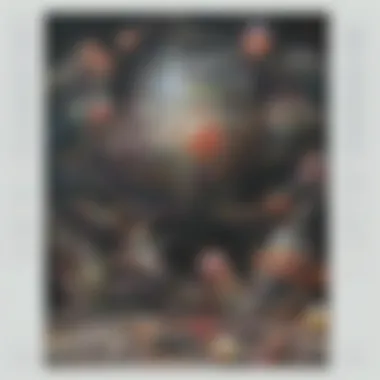
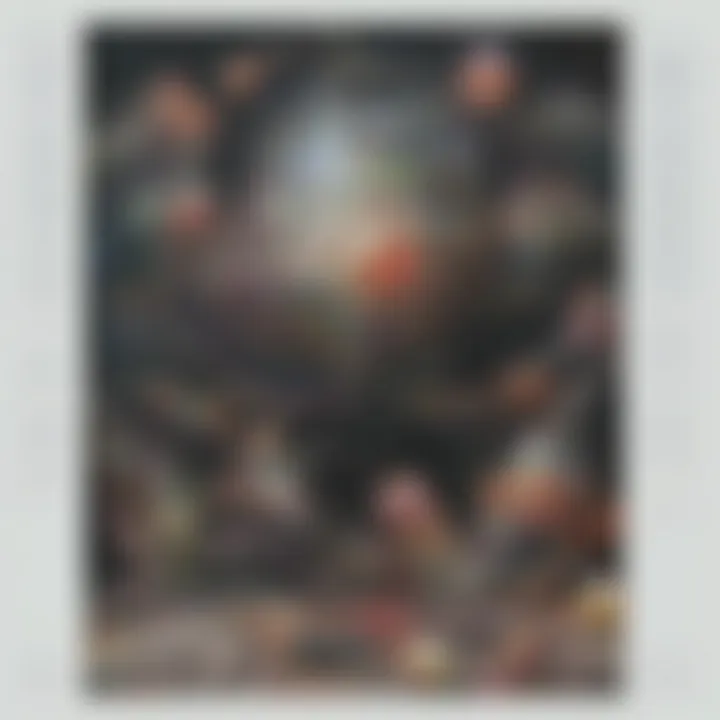
Overview of Deep Learning
Deep learning refers to a subfield of machine learning characterized by algorithms based on neural networks. These networks consist of layers of interconnected nodes that process data. Each layer extracts features, and subsequent layers refine these features to enable the model to learn complex representations. This structured approach has proven effective in many domains, including image recognition, natural language processing, and, importantly, protein structure prediction.
The advantages of deep learning largely stem from its ability to process massive arrays of data, enabling it to extrapolate insights from nuanced biological interactions that human researchers might overlook. Moreover, deep learning models continue to improve their performance with exposure to more data, shifting the paradigms of predictive modeling in biological research.
Neural Networks and Protein Structures
Neural networks are designed to mimic human cognitive processes, which makes them suitable for tackling the complex challenges of protein structure. Every protein's functionality is intricately tied to its three-dimensional configuration. This configuration arises from interactions among amino acids during folding.
Using deep learning, researchers can train neural networks to predict protein structures from amino acid sequences. Notable models like AlphaFold have shown remarkable success. This particular model uses advanced neural network architectures to simulate the folding processes, providing predictions that match experimental data.
The implications of these neural networks extend beyond mere predictions. They facilitate deeper understanding by uncovering relationships between sequence and structure, advancing personalized medicine and therapeutic strategies.
Case Studies in AI-Driven Research
Certain case studies illustrate the impact of AI in protein folding. One significant example is the use of deep learning to predict the SARS-CoV-2 spike protein structure. Researchers utilized networks that analyzed sequence data to ascertain structural parameters.
Additionally, a group at the University of Washington developed a model that could predict protein interfaces. This tool enabled researchers to not only predict folding but also assess how proteins might interact. Such case studies exemplify the capacity of deep learning frameworks to address problems that have long challenged biological science.
In summary, deep learning techniques are reshaping the field of protein folding. With ongoing advancements, the intersection of AI and protein structure prediction continues to promise transformative potential for scientific research and applications.
Implications of AI in Biological Research
Artificial intelligence has seeped into various scientific fields, and its implications within biological research are profound and expansive. The intersections of AI and biology have the potential to not only advance our understanding of protein folding but also propel various applications that can lead to notable advancements in health and medicine. Understanding these implications is crucial for a comprehensive view of how AI is reshaping the biological landscape.
Enhancements in Drug Discovery
The integration of AI technologies in drug discovery stands as a monumental benefit of merging artificial intelligence with biological research. AI can sift through massive datasets, identifying patterns that are often invisible to human researchers. This capability expedites the identification of potential drug candidates by predicting how different molecular structures interact with biological targets.
For instance, companies like Atomwise utilize AI algorithms to screen countless compounds for their effectiveness in treating specific diseases. Their deep learning models analyze existing database of compounds and match them against known biological targets. This process not only reduces the time required for drug discovery but also minimizes costs significantly. The result is a more efficient pathway to developing innovative therapies that can be advanced into clinical trials.
"AI can dramatically reduce the time and cost involved in discovering new drugs and understanding interactions at a molecular level."
Predicting Protein-Protein Interactions
AI's role doesnโt just stop at drug discovery; it extends into understanding how proteins interact within the human body. Predicting protein-protein interactions is fundamental in cellular biology. AI systems, particularly those leveraging deep learning, can give insights into these complex interactions. This predictive capability is critical for deciphering pathways that are essential in metabolic processes and disease mechanisms.
Machine learning models that have been trained with known protein structures and interactions can provide probabilities of interactions between novel protein pairs. Such predictions help categorize proteins into functional groups, offering researchers a clearer road map to study specific biological functions. This information can be pivotal in developing interventions that target these interactions in diseases where they become dysregulated.
Applications in Disease Research
The implications of AI in disease research have become increasingly clear. AI algorithms can analyze genetic data, environmental factors, and clinical histories to identify risk factors for diseases. Moreover, by correlating these factors with protein folding processes, researchers can uncover insights into diseases like Alzheimer's and Parkinson's, where protein misfolding plays a critical role.
AI applications in this realm include:
- Diagnostic Tools: Machine learning models can assist in diagnosing diseases by analyzing symptoms and biological data, improving accuracy and speed.
- Prognostic Models: AI helps predict patient outcomes based on various genetic markers and protein interactions, thus enabling personalized medicine approaches.
- Treatment Strategies: AI can provide insights into effective treatment combinations by analyzing the responses of different patient cohorts to various therapies.
The crossroad of AI and biological research promises to illuminate areas that were once obscured by data complexity and sheer volume. Ultimately, the implications extend beyond theoretical exploration, translating into tangible benefits in medical advancements, personalized treatments, and enhanced understanding of lifeโs molecular intricacies.
The Future of AI and Protein Folding
The fusion of artificial intelligence and protein folding presents promising potential for advancing biological sciences. As researchers understand more about proteins, the urgency for accurate predictive models intensifies. With AI, there are innovative prospects for breakthroughs in various aspects of protein science.
Emerging Technologies
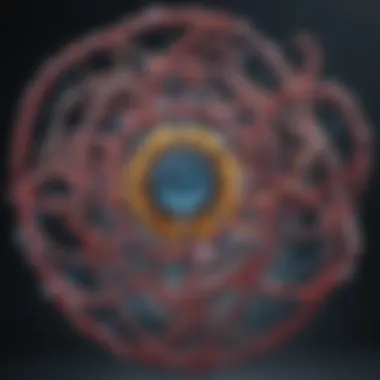
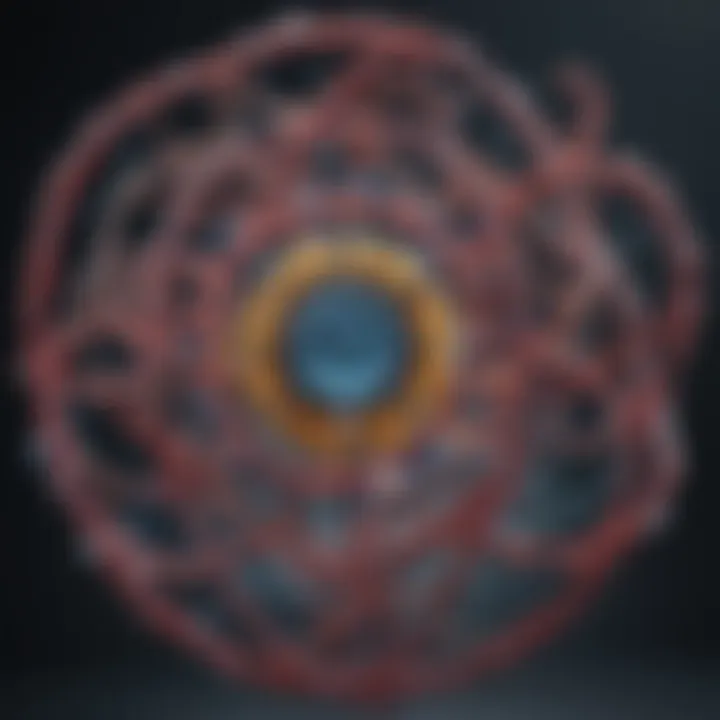
The rapid expansion in AI capabilities is fostering the emergence of novel technologies that change the landscape of protein folding research. Among these, techniques such as generative adversarial networks (GANs) and reinforcement learning are gaining traction.
- Generative Adversarial Networks (GANs): These networks help in creating realistic protein structures by learning from existing data. They can generate new protein configurations based on established principles, optimizing structure prediction.
- Reinforcement Learning: This technique teaches AI to make decisions by rewarding successful predictions. Such methods allow for adaptive learning, where AI continually improves its performance in predicting folding outcomes.
- Integration of Quantum Computing: This is an emerging frontier. Quantum computing has the potential to handle complex calculations much faster than traditional methods. This could prove useful in simulating protein interactions under various conditions.
As these technologies become more refined, they will likely enhance the accuracy of simulations, leading to more reliable protein models.
Ethical Considerations
With the advancement of AI in protein science, ethical considerations become paramount. As AI systems evolve to provide deeper insights, the responsibility associated with these advancements must be addressed. Several points warrant attention:
- Data Privacy: Protecting sensitive genetic data used in AI training is crucial. Researchers must ensure compliance with regulations to safeguard individual privacy.
- Bias in AI Algorithms: AI systems can inherit biases present in training data. It is essential to continually assess and mitigate these biases to ensure fair and equitable scientific inquiries.
- Responsible Use of AI Models: The potential for misuse in biotechnological research must not be overlooked. Ensuring that AI is used to benefit society in ethical ways is a concern that researchers must prioritize.
"As AI becomes integrated into biological research, itโs important to tread carefully, ensuring that ethical frameworks evolve alongside technological advancements."
Collaborations in Research
Collaboration is integral to harnessing the full power of AI in protein folding. By bringing together experts from diverse fields, such as computational biology, machine learning, and biochemistry, the intersection of these disciplines can lead to notable advancements. Key points to consider include:
- Interdisciplinary Teams: Working alongside statisticians, computer scientists, and biologists fosters a comprehensive approach. Different perspectives can improve the innovation rate and lead to breakthroughs.
- Public-Private Partnerships: Joint research ventures between academic institutions and private companies can accelerate the development of AI-driven protein research tools.
- Open Sharing of Data: Platforms that allow shared access to datasets can promote collaboration. This fosters innovation as researchers can apply AI methodologies to large datasets produced by others.
The future of AI and protein folding is bright, especially when the right collaborative strategies are in place. With technological innovations on the horizon, the effective integration of AI holds the potential to transform research and applications in biology in profound ways.
Epilogue
The conclusion of this article encapsulates the intricate relationship between artificial intelligence and protein folding, illustrating the potential and the challenges involved in this evolving field. First, it is essential to recapitulate the pivotal insights presented throughout the text. Understanding the synergy between AI methodologies and the complexities of protein structure prediction is vital for advancing biological research. These technologies not not only refine our approaches but also significantly impact drug discovery and disease understanding.
Recapitulation of Key Points
In summary, several key points have underscored the importance of AI in the context of protein folding. Those points include:
- The foundational role of protein folding in biological systems: Accurate protein structures are crucial for the proper functioning of organisms.
- AIโs transformative impact: AI significantly enhances our capacity to predict protein structures, overcoming challenges that have persisted in traditional methodologies.
- Real-world applications: AI facilitates advancements in drug discovery, aids in predicting protein-protein interactions, and contributes to our understanding of various diseases.
The integration of AI into protein folding processes not only improves speed and accuracy but also opens new avenues for research and medical applications. This can ultimately lead to more effective therapies and diagnostic tools.
Future Directions in Research
Looking forward, several areas present promising opportunities for further exploration within the intersection of AI and protein folding. These include:
- Optimizing AI algorithms: Ongoing improvements in machine learning models and neural networks may yield even more accurate protein structure predictions.
- Incorporating multi-scale modeling: Bridging atomic-level interactions with larger biological systems may enhance the contextual understanding of protein folding.
- Ethical considerations: As AI continues to advance, it is crucial to address ethical implications related to data privacy, accessibility, and the potential biases in training data.
Research collaborations between computational biologists and AI specialists can also lead to innovative methodologies.
Primary Literature
Primary literature refers to original research articles where data and observations are first published. This type of literature is critical in understanding the developments in AI applications for protein folding. Here are several notable benefits of incorporating primary literature:
- Novel Insights: Primary sources offer cutting-edge discoveries that can inform current understanding.
- Methodological Rigor: Researchers can scrutinize the methodologies used in these studies, evaluating the strength of the findings.
- Data Transparency: Original articles present data that allows for independent validation by other scientists.
Furthermore, key journals such as Nature, Science, and PLOS Biology frequently publish studies relating AI to molecular biology, providing a rich resource for this research area.
Online Resources and Databases
In addition to primary literature, online resources and databases play a pivotal role in facilitating research. Platforms like Protein Data Bank and UniProt provide extensive datasets crucial for understanding protein structures and folding mechanisms. The advantages of these resources include:
- Accessibility: They offer free access to structural data, making it available to a broad audience.
- Comprehensive Data: Researchers can access a range of proteins and their associated data points, enhancing predictive modeling efforts.
- Collaboration Opportunities: Online databases often encourage collaboration among researchers, fostering a community-driven approach to scientific exploration.
Important Resources to consider:
"Access to online resources has democratized information availability, impacting how rapidly science progresses."