Understanding Western Quantification: Historical Insights
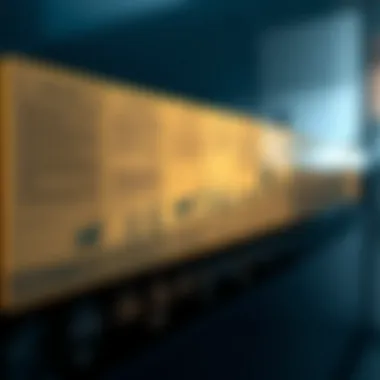
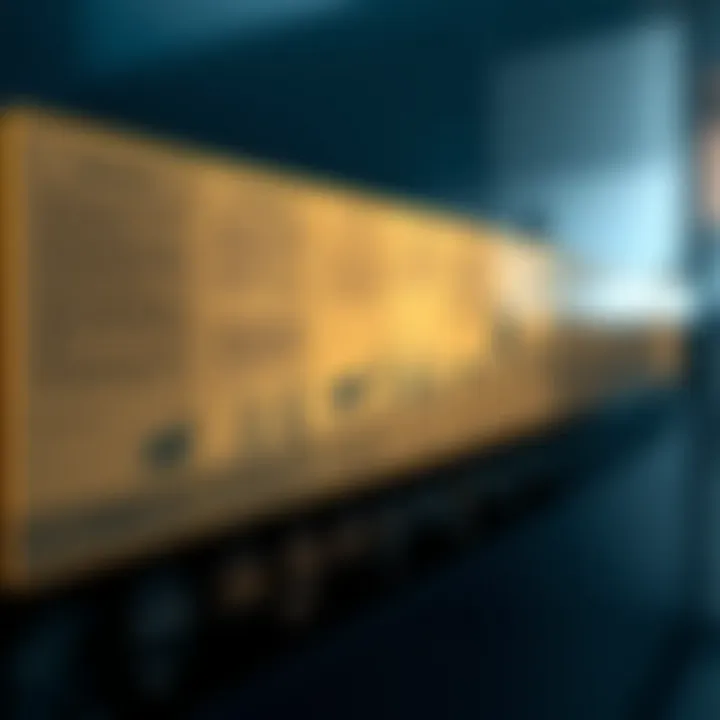
Intro
In an age where numbers reign supreme, the discourse surrounding western quantification becomes ever more critical. Quantification, as a foundational element in the scientific endeavor, has evolved significantly over time. This article aims to peel back the layers of this multifaceted concept, often taken for granted in various fields—the natural sciences, social studies, and even in humanities.
From its historical roots to contemporary methodologies, we will journey through how this practice shapes our understanding of reality. Not merely a matter of arithmetic or statistics, quantification affects how data is interpreted, how knowledge is constructed, and ultimately, how we perceive our world.
As we delve into these aspects, it’s essential to elucidate the implications that arise from an over-reliance on quantitative metrics. Especially in today’s data-driven landscape, the call for a critical re-examination of these paradigms is louder than ever. Understanding these elements thoroughly provides students, researchers, educators, and professionals with the necessary tools to navigate the nuances of scientific inquiry in our quantification-centric era.
The foundation we are building will be structured as follows:
Research Overview
Summary of Key Findings
The exploration into the realm of western quantification uncovers various pivotal conclusions that highlight its significance across disciplines. Through a detailed investigation, we find that quantification is not just a method but a lens through which knowledge is constructed, often dictating which aspects of phenomena are deemed worthy of study and which are overlooked.
Some of the key findings include:
- The historical context of quantification has heavily influenced how contemporary methodologies are structured.
- Various scientific fields exhibit a diverse reliance on quantification, showcasing the need for field-specific appraisal of data.
- A noticeable trend is observed where qualitative insights are sidelined in favor of numerical data, leading to potential gaps in understanding complex issues.
Methodologies Employed
In scrutinizing quantification methods, researchers have employed diverse strategies that vary among disciplines. Some noteworthy methodologies include:
- Statistical Analysis: Widely used across fields, this approach allows for meaningful conclusions based on numerical data.
- Surveys and Questionnaires: A technique that captures subjective experiences but often translates them into quantifiable data.
- Experimental Designs: Providing controlled environments where variables can be measured precisely, revealing insights that pure observation might miss.
Intro to Western Quantification
Understanding the concept of Western quantification is essential as it underpins much of modern scientific inquiry and discourse. This section sets the stage for exploring how quantification has evolved, the methodologies involved, and its impact on knowledge production. A deep dive into quantification reveals not only its strengths but also its limitations and nuances, urging scholars and practitioners alike to reassess its role in shaping contemporary understandings of the natural world.
Definition and Scope
At its core, Western quantification refers to the systematic approach of measuring phenomena, often employing numerical data and statistical methods. It is indispensable in various fields, including the social sciences, natural sciences, and even in the humanities. The scope expands as we look at how this measuring process influences theoretical frameworks, research methodologies, and the general interpretations of data. Understanding definition and scope helps clarify what we mean by quantification and why it matters.
Historical Context
Exploring historical context provides a lens through which we can trace the philosophical and practical development of quantification through time.
Roots in Western Philosophy
The roots of Western quantification can be traced back to ancient philosophers like Aristotle and Plato, who laid the groundwork for rational inquiry and empirical observation. This aspect of philosophical thought emphasizes logic and systematic categorization, leading to a more structured way of understanding the surrounding world. Such early philosophical roots are a beneficial inclusion as they illustrate the longstanding tradition of quantifying knowledge that informs current methodologies. A unique feature here is the juxtaposition of qualitative observation against emerging quantitative methods, which has led to rich debates in epistemology. However, it also raises questions about the limitations of purely numerical representations in capturing the full essence of human experience.
Early Scientific Endeavors
As we moved into the scientific revolution, figures like Galileo and Newton began employing quantification not merely as a tool but as a cornerstone of scientific validation. One key characteristic of these early scientific endeavors is the rigorous application of mathematics to natural phenomena. This practical aspect dramatically solidified quantification's position in science, making it a popular choice for the foundations of contemporary research. It's important to note that while the use of quantification propelled scientific advancement, it came with challenges. One such challenge was the struggle to translate human and environmental complexity into mere numerical values, inevitably leading to oversimplification.
Transition to Modern Quantification
With the advent of the 19th and 20th centuries, methodologies further evolved, refining how quantification is practiced in various fields. This transition to modern quantification is characterized by the development of statistical theories and computational techniques that enabled researchers to handle large datasets and complex variables efficiently. The benefits of this shift cannot be overstated; it opened doors to new forms of inquiry and laid the groundwork for advancements in data analysis. However, the rise of big data also introduced significant ethical concerns and issues surrounding data interpretation, impacting our understanding within scientific communities.
"Quantification, while powerful, carries the weight of representation, demanding rigorous scrutiny at every step of the research process."
This narrative illustrates that although Western quantification offers valuable insights and systematic methodologies, its historical journey is fraught with complexities that continue to shape contemporary practices.
In the sections to come, we will further dissect methodologies, critiques, and the impacts of quantification on scientific discourse, allowing us to paint a comprehensive portrait of its role in science.
Methodologies of Quantification
The methodologies used for quantification play a pivotal role in shaping modern scientific inquiry. They are the backbone that provides consistency, accountability, and a framework for analyzing complex phenomena. Understanding these methodologies allows researchers and practitioners to select appropriate methods for gathering and interpreting data.
In this section, we will delve into various approaches, exploring the strengths and weaknesses of quantitative and qualitative methods, the statistical techniques that underlie data analysis, and the frameworks that guide experimental designs.
Quantitative vs. Qualitative Approaches
Defining Quantitative Research
Quantitative research is grounded in the collection and analysis of numerical data. It often utilizes statistical techniques to generate results that can be extrapolated beyond the sample studied. Importantly, this method allows for a structured and systematic exploration of hypotheses, providing clear and objective measurements. One key characteristic is its focus on numbers; the data tells the story through metrics and charts rather than narratives.
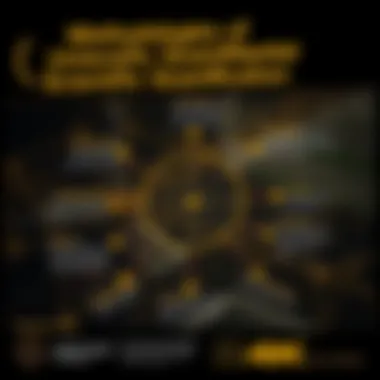
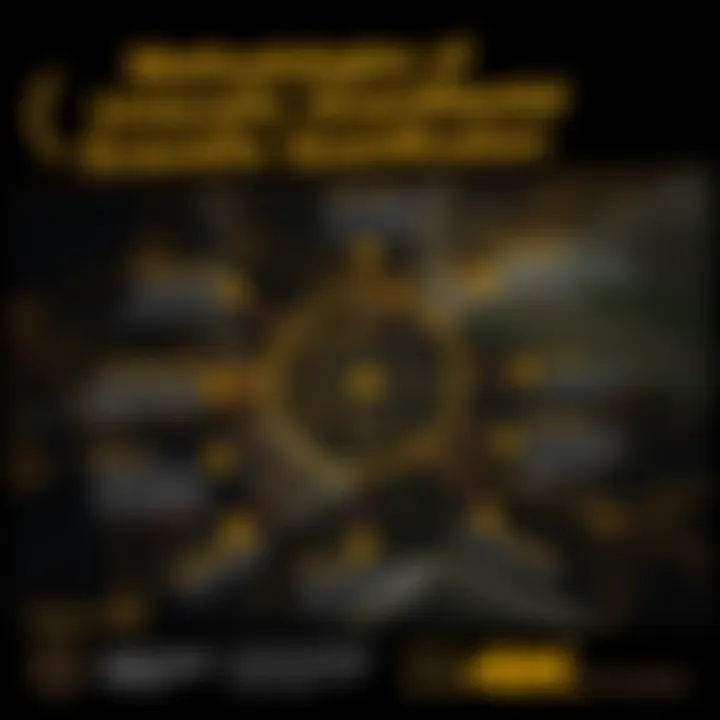
The benefits of quantitative research include its ability to handle large datasets effectively, yielding results that are easily interpretable and can facilitate comparisons across different contexts. However, this method is sometimes criticized for oversimplifying complex human experiences, reducing them to mere figures, which can lead to a loss of nuance.
Engaging with Qualitative Perspectives
Qualitative research delves into understanding human behavior, thoughts, and emotions through methods like interviews, focus groups, and content analysis. This approach prioritizes context, capturing rich, detailed narratives that quantitative methods might overlook. A crucial aspect of engaging with qualitative perspectives is its ability to provide depth. It’s not just about the ‘what’ but the ‘why’ behind phenomena.
Such insights can unveil unique viewpoints, allowing researchers to grasp social dynamics and cultural factors at play. However, qualitative methods can be seen as subjective. The findings might vary significantly depending on the researcher’s interpretation, which poses challenges for ensuring reliability.
Integrative Frameworks
Integrative frameworks seek to marry the strengths of both quantitative and qualitative research, creating a holistic view of the research problem. This approach acknowledges that certain research questions may necessitate both statistical analysis and narrative context. The noteworthy characteristic of these frameworks is their adaptability, allowing researchers to address complex issues through diverse methodologies.
The advantages here are substantial; by integrating different perspectives, researchers can enrich data interpretation and provide a more comprehensive understanding. Yet, this process can also be challenging due to the need for researchers to be proficient in both quantitative and qualitative techniques, often involving a steeper learning curve.
Statistical Techniques
Descriptive Statistics
Descriptive statistics form the groundwork for summarizing and interpreting data, offering a snapshot of the characteristics of a dataset. Key elements like mean, median, mode, and standard deviation come into play, providing useful insights into data distribution. This method is beneficial as it helps in translating massive volumes of raw data into comprehensible summaries.
The unique feature of descriptive statistics is its straightforwardness. It provides clear, concise information that is easily digestible. However, it’s often criticized for lacking depth, as it does not infer conclusions beyond the data at hand.
Inferential Methods
Inferential methods push the boundaries further, allowing researchers to make predictions or inferences about a larger population based on a sample. This is where concepts like hypothesis testing and confidence intervals come into focus. A central characteristic of these methods is their capacity to generalize findings, which is vital for establishing broader applicability.
The advantage of employing inferential methods lies in their ability to derive insights that extend beyond mere observations. Nevertheless, they come with inherent risks, particularly concerning sample bias, which can skew results and lead to erroneous conclusions.
Emerging Statistical Models
As the landscape of research evolves, so do statistical models. Emerging statistical models often incorporate advanced methodologies such as machine learning and Bayesian statistics. These models can process vast datasets and uncover patterns that traditional techniques might miss. One significant characteristic is their ability to continuously adapt and refine as more information becomes available.
The benefits of these models are numerous, especially in handling complexity and uncertainty in data. However, they can also introduce challenges, particularly with interpretability and the need for sophisticated understanding to implement effectively.
Experimental Designs
Controlled Experiments
Controlled experiments are foundational in establishing causality, where variables are manipulated in a controlled environment to observe effects. A defining characteristic is the random assignment of participants, which helps eliminate bias. This design is valuable for its ability to isolate specific variables and directly measure outcomes.
The major advantage lies in its strong internal validity. Conclusions drawn from these experiments are often regarded as robust. However, the limits of generalizability to real-world settings can be a downside, as controlled settings may not always replicate everyday conditions.
Field Studies
Field studies take research outside the lab into natural settings, offering a more contextual understanding of phenomena. This approach captures behaviors and interactions in real-world environments. One key feature is its authenticity; the data reflects actual practices rather than artificially constructed scenarios.
Such studies typically provide a rich context that enhances relatability for findings. However, the challenge lies in controlling external variables, which can introduce confounding factors into the research.
Longitudinal Approaches
Longitudinal approaches study subjects over an extended period, enabling exploration of changes and developments over time. A pivotal characteristic is the ability to track the evolution of phenomena, offering insights into causation rather than mere correlation.
This method’s strength is in its depth, allowing researchers to observe long-term impacts and trends. Yet, the disadvantages include resource intensity and the potential for participant attrition, which may limit the study’s validity if the dropout rates are high.
Critiques of Western Quantification
The discussions around western quantification often prompt a vital critique that speaks to its limitations and ethical considerations. Understanding these critiques is key to engaging deeply with the topic. These critiques raise red flags regarding the methodologies employed and how they can often oversimplify complex natural phenomena or distort data to serve certain narratives.
Limitations in Scope
Reductionism
Reductionism stands out as a significant aspect within the critique of western quantification. At its core, reductionism seeks to understand systems by breaking them down into their simpler parts. This approach often gains traction due to its straightforward and methodical nature. It provides a clear pathway to approach complex issues by dissecting them piece by piece. However, this method can lead to the myopic view of phenomena. For instance, when studying human behavior, focusing solely on neurotransmitter levels might ignore the broader social context or historical influences that shape actions.
The major characteristic of reductionism is its emphasis on quantifiable aspects, making it appear benficial for various scientific inquiries. Yet this oversimplification stifles the nuances inherent in the subjects under study. Consequently, while it offers clarity, reductionism detracts from the rich, layered understanding necessary for holistic analyses.
Unique Features and Disadvantages
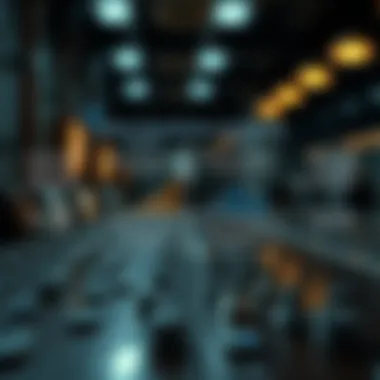
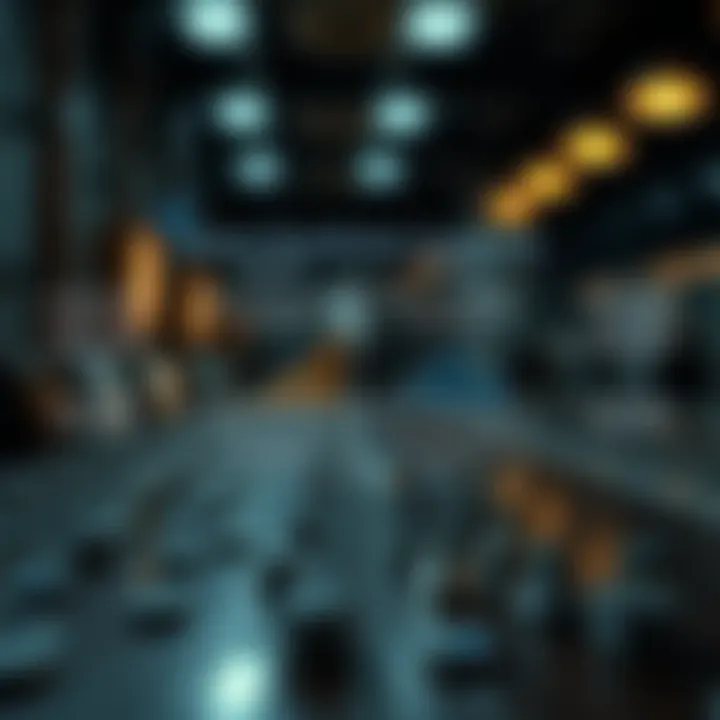
- Simplification: Reductionism often glosses over complexities.
- Scientific Popularity: Its clear-cut nature makes it popular among researchers.
However, the drawback here is substantial: relying too heavily on this approach limits insight into how interconnected systems work. In the context of this article, reductionism serves as a cautionary tale of how oversimplification can mislead research.
Neglect of Contextual Factors
Neglect of contextual factors is yet another critical concern when discussing western quantification. This aspect often reveals how a purely quantitative lens can overlook important dimensions that contribute to understanding a phenomenon. For example, a study examining educational achievement solely through grades might omit factors such as socioeconomic background, access to resources, or cultural influences that significantlly impact outcomes.
The failure to consider context raises the question of validity. It could lead to misleading conclusions about what influences certain results. This aspect is relevant, as it highlights how crucial it is to incorporate qualitative insights into quantification methodologies. Keeping context front and center allows for a more comprehensive analysis.
Unique Features and Advantages/Disadvantages
- Multi-layered Understandings: Acknowledging context enriches interpretations.
- Validity: Incorporating diverse factors enhances research accuracy.
Despite its advantages, ignoring contextual factors often skews the results. Within this article, examining this neglect unearths a vital lens through which to critically navigate the quantification landscape.
Ethical Considerations
Data Misrepresentation
Data misrepresentation can severely taint the integrity of research. This phenomenon occurs when data is presented in a way that is misleading or deceptive. It can manifest in various forms, including cherry-picking data points or distorting visual representations in graphs. For instance, a researcher might selectively report data that supports a hypothesis while omitting contradictory evidence. This manipulation isn't just a technical error; it's an ethical blunder that compromises the research's credibility.
Highlighting this issue invites scrutiny into the trustworthiness of data sources and the motivation behind research conclusions. In the realm of academic discourse, where data is often considered holy grail of truth, navigating this ethical minefield becomes critical.
Unique Features and Advantages/Disadvantages
- Trust Issues: Misrepresentation erodes public trust in data-driven fields.
- Scientific Integrity: Upholding rigorous standards ensures robust findings.
Implications for Subjectivity
The implications of subjectivity in research cannot be ignored. After all, quantification does not exist in a vacuum; it is influenced by human biases and perspectives. The way questions are framed or how variables are measured can reflect the researcher’s assumptions and beliefs. For example, employing a particular metric can segregate data in ways that reinforce existing power dynamics or social constructs.
This recognition of subjectivity is vital, urging a more nuanced understanding of how research can shape and sometimes distort realities. It provides compelling reasons for adopting mixed-methods approaches, combining qualitative and quantitative insights to counterbalance inherent biases.
Unique Features and Advantages/Disadvantages
- Nuanced Perspectives: Acknowledging subjectivity enriches research depth.
- Biases: Subjectivity can taint outcomes and interpretations.
Addressing these ethical considerations reveals the multifaceted dimensions in the landscape of quantification. By disaggregating these elements, we illuminate not just the critiques of the methodologies but their profound implications for scientific discourse itself.
The Impact of Quantification on Scientific Discourse
Quantification has become a cornerstone in scientific fields, affecting how knowledge is generated, communicated, and engaged with. The ability to assign numerical values to natural phenomena not only aids researchers in drawing conclusions from their work but also plays a critical role in shaping ongoing debates and inquiries. As such, understanding the implications of quantification is vital for academics, policymakers, and the general public alike.
Influence on Knowledge Production
Shaping Hypotheses
When we talk about shaping hypotheses, it’s clear that quantification adds a layer of precision to scientific reasoning. Researchers often draft their initial hypotheses based on observable trends, but numerical data helps to sharpen these ideas into testable constructs. This characteristic of quantification is both powerful and beneficial. It allows scientists to formulate hypotheses that not only reflect the subtleties of their observations but also hold up against statistical scrutiny. In other words, they are able to create predictions grounded in empirical evidence, which enhances the credibility of their work.
One unique feature of this approach is its emphasis on falsifiability. Using quantification, researchers can establish benchmarks that clearly indicate whether an outcome supports or refutes a hypothesis. This rigorous structure can lead to significant advances in a given field, but it also has its downsides. There can be a temptation to oversimplify complex phenomena into numerical data, risking a loss of depth in understanding.
Directing Research Funding
Directing research funding based on quantification has become a prevalent practice in academia and governmental institutions. The focus on metrics allows funding bodies to allocate resources effectively, ensuring that research that demonstrates strong quantitative findings receives attention. This aspect not only highlights the utility of quantification in driving knowledge production but also emphasizes its role in prioritizing particular research areas, shaping the scientific landscape.
A key characteristic here is the ability to measure outcomes against set performance indicators. By relying on quantifiable data, funding agencies can justify their decisions, thus promoting transparency. However, this strong reliance on numbers can lead to unintended consequences, such as underfunding vital but less quantifiable areas of research, which can stabilize certain paradigms while neglecting emerging or interdisciplinary fields.
Communication and Public Understanding
Translating Data for Lay Audiences
Communicating scientific findings to the public is fraught with challenges, especially when it comes to translating complex data into relatable insights. Quantification plays a crucial role here, allowing scientists to summarize their findings in a way that is digestible for lay audiences. This characteristic is essential in bridging the gap between research and public understanding.
The unique feature of this translation lies in its adaptability. Researchers can transform technical jargon into accessible language using quantifiable data, helping non-experts appreciate the value of scientific research. However, oversimplification can occur, risking the potential for misinterpretation. Without careful handling, important nuances may be lost.
Role of Visual Data Representation
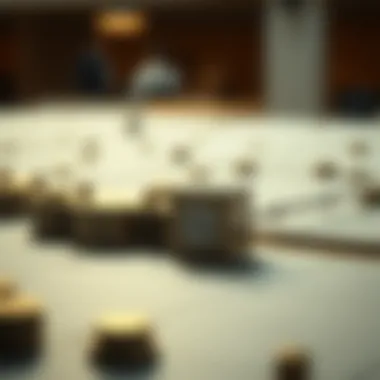
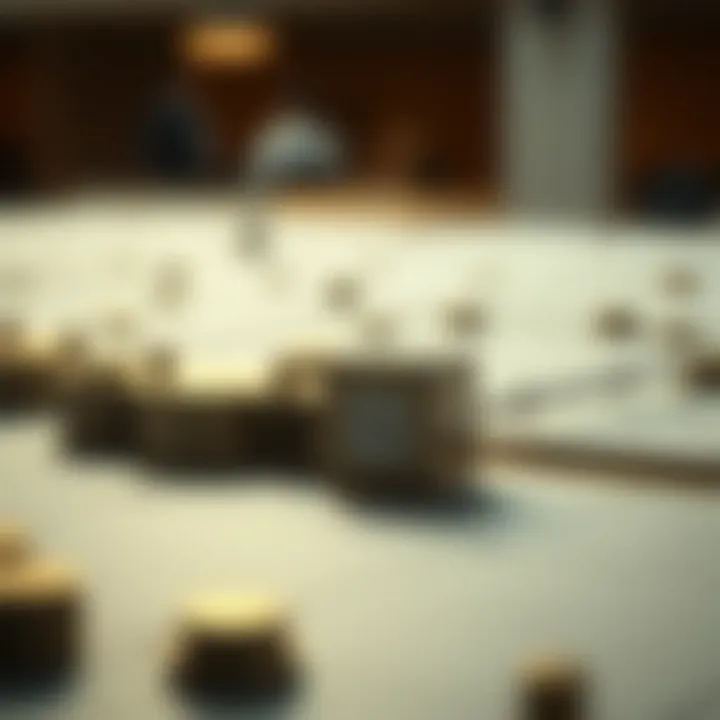
Visual data representation is another significant aspect that underscores the impact of quantification on communication. Effective visualizations—like charts, graphs, and infographics—can immediateley illustrate trends and relationships within data, making complex ideas more comprehensible to a broader audience. This is undoubtedly a powerful tool in conveying scientific concepts effectively.
One of the best aspects of visual data representation is that it capitalizes on our innate ability to recognize patterns quickly. When people see a visual representation of data, they can often grasp the essence of findings much more rapidly than if they were presented with raw numbers or dense texts. However, it's important to meticulously design these visuals. Misleading graphs or poorly constructed visuals can lead to significant misunderstandings, which may undermine trust in scientific findings.
"Quantification's role stretches beyond mere data collection; it fundamentally alters the fabric of scientific discussions, influencing everything from hypotheses to how the public engages with research outcomes."
In summary, the impact of quantification on scientific discourse is profound and multi-faceted. By shaping hypotheses, directing funding, and enhancing communication, quantification has transformed how we understand and contextualize scientific knowledge. Yet, with these advantages come pitfalls that require prudent consideration. The ability to balance the merits of quantification with the nuances of scientific inquiry will ultimately determine how effectively knowledge is produced and shared in future endeavors.
Alternative Approaches to Understanding Natural Phenomena
In diving into the subject of Western quantification, it is crucial to acknowledge alternative methodologies that provide a broader perspective when examining natural phenomena. These approaches often serve as counterpoints to traditional Western methods, emphasizing the richness of understanding that can emerge from integrating diverse epistemologies. Understanding these alternative paradigms not only challenges the dominance of quantitative analyses but also enriches our comprehension of complex systems and respects the validity of various sources of knowledge.
Indigenous Knowledge Systems
Multi-faceted Understandings
Multi-faceted understandings are paramount in today's discourse about knowledge acquisition. They emphasize that the world is not merely a collection of quantifiable data points, but a tapestry of interconnected elements, each with its own significance. This viewpoint brings forth a critical characteristic—depth of context. These understandings allow for a richer, more textured insight into phenomena that statistical measures may overlook, making them a compelling choice for examination in this article.
One unique feature of multi-faceted understandings is their adaptability across cultures and environments, offering fresh perspectives on sustainability and ecological balance. While they shine in contextual richness, a potential disadvantage lies in their subjective nature. Critics might argue that these approaches lack the rigour often associated with quantitative methods. Yet, their strength lies in their ability to capture the nuances of experience that numbers alone cannot convey.
Integrating Oral Traditions
Integrating oral traditions into scientific discourse adds a layer of historical continuity and cultural relevance. Oral traditions are passed down through generations and often encapsulate deep understandings of land, resources, and ecological interactions. This characteristic of longevity makes these traditions crucial for holistic environmental knowledge, positioning them as a beneficial choice for this article's exploration.
The key aspect of integrating oral traditions is their inherent reflection of collective experiences and wisdom. In many Indigenous communities, these narratives inform issues of land stewardship and natural resource management. They offer unique insights into climate phenomena and biodiversity protection. Nonetheless, one must recognize the challenge of translating these oral accounts into contemporary scientific frameworks without losing their essence.
Interdisciplinary Perspectives
Collaborative Scientific Models
Collaborative scientific models advocate for an integrative approach that brings together experts from varied fields to tackle complex problems. This paradigm champions the merging of diverse viewpoints and methodologies, helping create a more comprehensive understanding. The key characteristic here is the synergistic potential of interdisciplinary work, making it a popular theme in this article.
A standout feature of collaborative models is their pragmatic approach to problem-solving. By pooling resources and expertise, these models foster innovation. They encourage solutions that might remain hidden in siloed environments. However, while collaboration often leads to more robust findings, managing differing methodologies and terminologies can become challenging. Thus, navigating these complexities is vital.
Bridging Gaps Between Disciplines
Bridging gaps between disciplines is an essential effort in addressing multifaceted issues in contemporary science. This concept underlines the importance of fostering communication across specialized fields, allowing for a more cohesive narrative in understanding natural phenomena. Its key characteristic lies in its inclusivity, encapsulating various paradigms that range from the empirical to the anecdotal, making it a valuable aspect to discuss in this article.
What distinguishes this approach is its potential to uncover insights at the intersections of knowledge. For instance, environmental science and traditional ecological knowledge might not always intersect, yet bridging these gaps can yield transformative insights. However, there’s a challenge here; reconciling differing cultural perspectives and scientific methodologies can be intricate, as tension might arise from misunderstandings. Educating stakeholders on the value of interdisciplinary dialogue becomes indispensable to making these connections fruitful.
In embracing the diversity of knowledge systems, we can foster a deeper, more resonant understanding of the world around us.
Future Directions in Quantification
As we navigate through the complexities of Western quantification, it’s imperative to look forward, reflecting on how the practices and ideologies of today can shape our understanding tomorrow. The significance of scrutinizing future directions in quantification lies not only in the potential for augmenting our existing methodologies but also in addressing the limitations previously encountered. By engaging with cutting-edge elements like technological advancements and the evolution of scientific paradigms, one can anticipate a more nuanced approach to measuring and interpreting the myriad phenomena that frame our scientific discourse.
Technological Innovations
Big Data Analytics
Big data analytics stands out as a transformative force in modern quantification practices. With the explosion of data generated in areas like social media, healthcare, and environmental science, the ability to process and derive insights from this vast information pool has become essential. The most compelling characteristic of big data analytics is its capacity to uncover patterns and correlations that were previously obscured. This adaptability makes it a favorite in many research disciplines.
A unique feature of big data analytics is its performance through machine learning algorithms. These algorithms sift through enormous datasets, extracting valuable insights that can guide scientific investigations. However, despite its strengths, big data faces scrutiny concerning data privacy issues and the potential for misinterpretation of results. It is crucial that researchers balance the pursuit of knowledge with ethical use of the data collected.
Artificial Intelligence in Research
Artificial Intelligence (AI) emerges as another pivotal contributor in the evolving landscape of quantification. A notable aspect of AI in research is its predictive modeling capabilities. Algorithms designed with AI can simulate complex scenarios, providing researchers with forecasts that aid decision-making processes. The automation of data processing and analysis is one key characteristic that enhances efficiency, making AI an attractive option for academics and industry experts alike.
Among its distinct advantages, AI simplifies the handling of large datasets, allowing for rapid analysis and yielding results almost in real-time. On the downside, there’s a growing apprehension regarding the biases that may creep into AI algorithms based on the data they are trained on. This dilemma underscores the importance of scrutinizing both the methodologies and the data sources used in AI-driven research.
Evolving Scientific Paradigms
Emphasis on Interdisciplinarity
The current scientific landscape is increasingly characterized by an emphasis on interdisciplinarity. This perspective fosters collaboration between varying fields, enabling a more holistic approach to addressing complex issues. One key characteristic of this trend is the breakdown of traditional silos. By integrating insights from disciplines like biology, sociology, and computer science, researchers can craft more comprehensive frameworks for understanding the world around them.
A unique feature of this interdisciplinary focus is the heightened capacity for problem-solving. Complex global challenges, from climate change to public health crises, require collaborative input. However, one must remain cautious of the potential difficulties in communication and methodology standardization that can arise from such collaborations. Ensuring a clear dialogue among diverse fields is essential to reap the benefits of this approach.
Shifting Ethical Standards
Lastly, the concept of shifting ethical standards cannot be overlooked when examining future directions. Ethical considerations are becoming increasingly vital as scientific methods evolve. A key characteristic of this shift is the growing public demand for transparency and accountability in research. With rising awareness around data usage—especially in sensitive fields such as genetics and social sciences—questions about consent and data integrity take center stage.
This evolution in ethical standards presents a unique opportunity for researchers to engage in more responsible practices. On the flip side, it introduces challenges, notably in navigating the complexities of ethical guidelines that can differ across cultures and jurisdictions. As we embrace these new standards, a unified approach to ethics can help cultivate trust among researchers and the communities they serve.
"The future of quantification is not merely a matter of refining our tools but also about reconciling the ethical implications they carry."