ForData: A Comprehensive Analysis of Data Implications
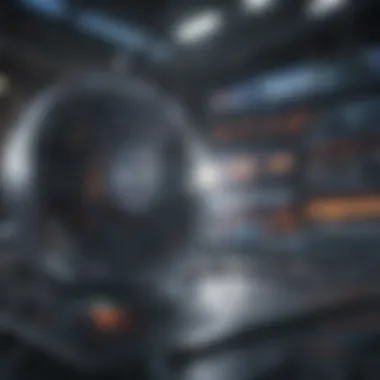
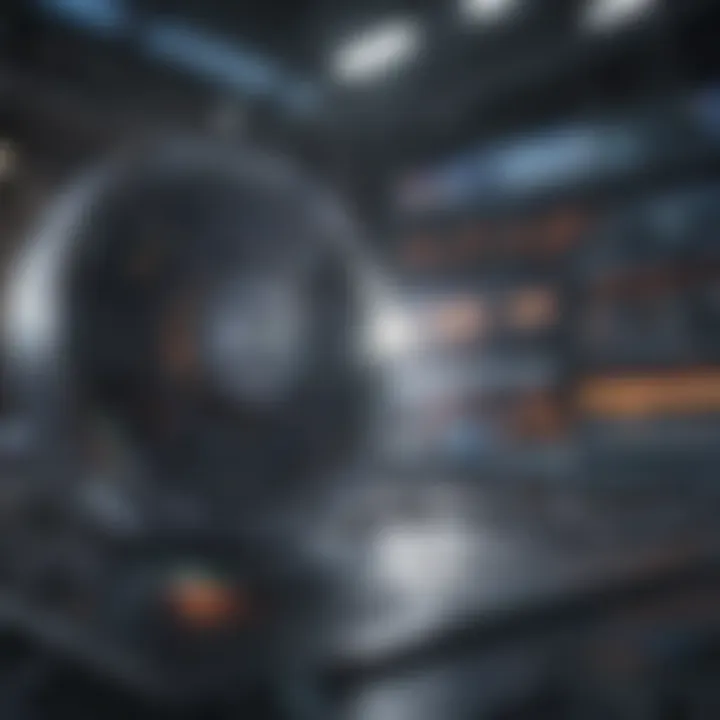
Intro
In recent years, the field of data management has gained significant attention, especially with the rise of technology and the increasing value of data in scientific research. βForDataβ serves as a notable concept that helps in understanding the broader implications of data within various disciplines. This article aims to unpack the intricate relationship between data, methodology, and ethics in research, providing valuable insights for students, educators, and professionals alike.
Research Overview
The foundation of this exploration lies in the understanding of data-centric approaches within research paradigms.
Summary of Key Findings
The analysis reveals several critical aspects:
- Data is not just a byproduct of research; it is a key asset that drives scientific inquiry.
- Ethical considerations are paramount as data utilization raises questions concerning privacy, representation, and bias.
- Methodological advancements are ongoing, reshaping how data is gathered, analyzed, and interpreted.
These findings indicate that researchers must not only be aware of the data they use but also the ethical implications of their methodologies.
Methodologies Employed
To conduct this in-depth analysis, various methodologies have been employed, including:
- Literature review of existing studies on data management.
- Case studies showcasing practical applications in different scientific fields.
- Interviews with researchers to gain firsthand insights into challenges and best practices.
Through a synthesis of these methods, the article presents a thorough overview of how data practices evolve and adapt to the changing landscape of research.
In-Depth Analysis
Delving deeper into the practical aspects of data usage, this section examines the results achieved through meticulous data management in various disciplines.
Detailed Examination of Results
The analysis highlights substantial progress in areas such as:
- Enhanced data visualization techniques that facilitate better comprehension of complex datasets.
- Improved data collection processes that emphasize accuracy and reliability.
- Innovative analytical tools that allow for faster processing and interpretation of data.
Thus, understanding these advancements is essential for any researcher looking to harness the full potential of data.
Comparison with Previous Studies
Comparatively, contemporary research has evolved significantly from earlier work, such as:
- The simple statistical methods previously employed now give way to sophisticated algorithms and machine learning.
- Ethical considerations are now integrated into the research design phase, contrasting with past practices where they were often an afterthought.
This comparison illustrates a shift towards a more holistic approach in both data handling and ethical responsibility, reinforcing the idea that data is integral to sound scientific inquiry.
Understanding ForData
When exploring the world of data, the concept of ForData emerges as crucial. It involves examining not only the data itself but also the processes behind its collection, analysis, and interpretation. Understanding ForData allows individuals and organizations to grasp how data informs research and decision-making. With an increasing reliance on data across various fields, gaining insights into its management becomes imperative.
Definition and Context
ForData is an interdisciplinary framework focused on data handling within scientific research. It encompasses all aspects of data, from its generation to its final use. At its core, ForData emphasizes the need for structured methodologies and ethical practices in handling data. The context of ForData aligns with the growing significance of accurate data in ever-evolving research environments. In short, understanding ForData helps stakeholders appreciate the complexities behind data-generated conclusions.
Historical Background
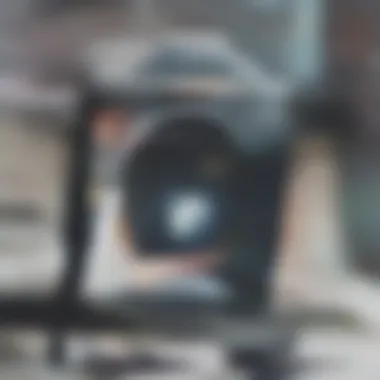
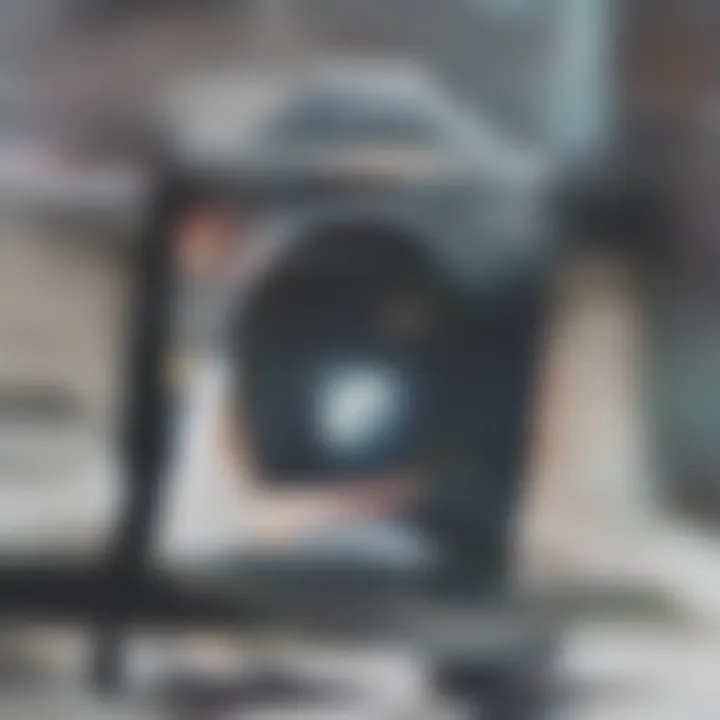
Historically, the evolution of data management reflects advancements in technology and methodology. In the early days, data collection was often manual and prone to errors. The rise of computers in the late 20th century marked a pivotal change. Researchers began to utilize advanced software for data storage and analysis, leading to more reliable outcomes. Over time, the availability of vast datasets due to the internet has transformed the landscape. Today, organizations leverage data not only for research but also for strategic initiatives. Recognizing this historical trajectory underlines the importance of ForData in contemporary research practices.
The Role of Data in Scientific Research
Data holds a fundamental role in scientific research, acting as the backbone of methodologies and conclusions. Without data, researchers are left with unvalidated assumptions rather than concrete findings. The reliability of research outcomes hinges on the quality and integrity of the data collected. This section explores how data influences research across various scientific domains, emphasizing the need for robust data practices and innovative applications.
Types of Data in Research
Qualitative Data
Qualitative data encompasses non-numerical information that seeks to capture subjective qualities or experiences. Its value lies in understanding complex phenomena through detailed descriptions. Commonly used in fields such as psychology and anthropology, qualitative data provides depth and context. It might come from interviews, focus groups, or open-ended survey responses.
One key characteristic of qualitative data is its ability to explore the motivations behind behaviors. This makes it a popular choice for researchers wanting a nuanced understanding of human experiences. However, the analysis of qualitative data can be time-consuming and subject to researcher bias, posing challenges to its reliability across broader samples.
Quantitative Data
Quantitative data, on the other hand, is structured and numerical. It allows for statistical analysis and the formulation of hypotheses. Researchers depend on quantitative data to provide clear evidence in studies, commonly utilizing measurements and measurements to represent variables. This type of data is essential in fields such as medicine and economics.
A distinct feature of quantitative data is its ability to yield results that can easily be generalized to larger populations. This strengthens its role in validating research claims. Nevertheless, while it offers precision, quantitative data may overlook deeper insights into human behavior that require qualitative understanding.
Data Collection Methods
Surveys
Surveys are a prevalent method for data collection, enabling researchers to gather information from large groups efficiently. They typically consist of structured questionnaires. This method facilitates the collection of both qualitative and quantitative data, depending on the design. Surveys can reach diverse populations through various mediums, such as online platforms or paper forms.
One key advantage is the anonymity they provide, which may lead to more honest responses. However, surveys may suffer from issues like survey fatigue or non-response bias, affecting the validity of the data gathered.
Experiments
Experiments allow researchers to test hypotheses under controlled environments. This method provides a systematic way to ascertain cause-and-effect relationships between variables. Researchers manipulate one or more independent variables while keeping other factors constant. This controlled setting ensures that results are attributable to the variable being tested.
A significant benefit of experiments is their ability to establish causality rather than mere correlation. Yet, they can be resource-intensive, and ethical considerations must be addressed, particularly involving human subjects.
Field Studies
Field studies involve observing and collecting data in real-world settings rather than controlled environments. This approach offers researchers insights into behaviors as they occur naturally, providing an authentic context. Field studies can incorporate a variety of methods, from direct observation to immersive participation, allowing for rich data collection.
Their major advantage is the ecological validity they lend to findings. Nevertheless, they can encounter obstacles, such as uncontrolled variables, which may confound the results.
"Data's role in research is not merely supportive; it is foundational, turning theories into evidence and allowing for advancements across disciplines."
Understanding these methods and types of data is crucial for advancing scientific research. Each offers unique insights and presents its own set of advantages and disadvantages. The choice of method often determines the scope and impact of the research findings.
Data Management Practices
Data management practices are essential in modern research settings. They encompass a range of processes that ensure data integrity, accessibility, and usability throughout the data lifecycle. Effective data management not only enhances the quality of scientific research but also addresses legal and ethical considerations regarding data handling. Understanding these practices is crucial for students, researchers, educators, and professionals who rely on data-driven insights.
Good data management practices have several benefits. First, they promote efficiency by facilitating easy data access. When researchers adhere to structured data management, they can save time during data retrieval and analysis, which enhances overall productivity. Second, robust data management contributes to data quality. Proper organization, documentation, and maintenance reduce the risk of data loss and inaccuracies. Lastly, these practices are crucial for compliance with regulatory policies, ensuring that research adheres to ethical standards.
Data Storage Solutions
Data storage solutions refer to the methods used to store data securely. These solutions can vary from physical storage devices to cloud-based systems. Choosing the right data storage method is significant because it impacts data accessibility and security.
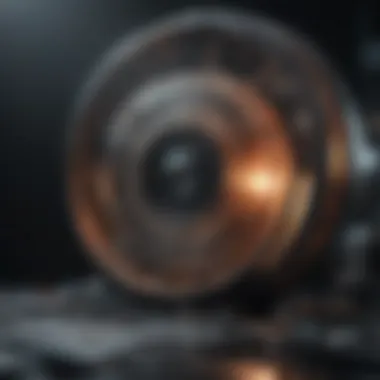
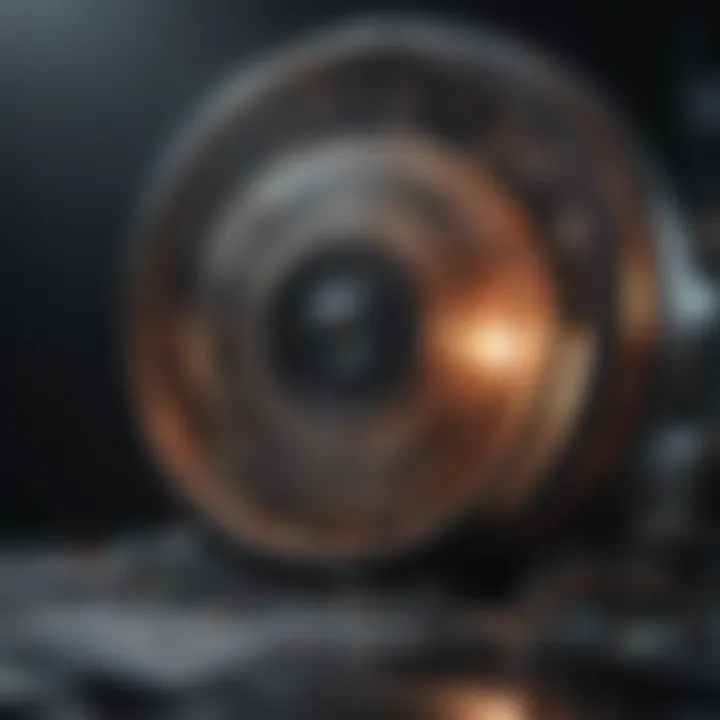
Physical storage options include hard drives, external drives, and network-attached storage. These systems are traditionally used but come with risks, such as data deterioration over time and potential for physical damage.
On the other hand, cloud storage solutions like Google Drive, Dropbox, or Amazon S3 offer versatility and ease of use. Data can be accessed remotely, and scalability becomes easier as data volume increases. However, the security of cloud storage must be managed actively, as sensitive data may be vulnerable to breaches if not properly protected.
Key Considerations in Data Storage:
- Accessibility: Choose a solution that allows easy access to authorized users.
- Scalability: Ensure the solution can grow alongside your data needs.
- Cost-effectiveness: Assess the budget implications of different storage options.
- Security: Implement encryption and access controls to protect stored data.
Data Security and Privacy
Data security and privacy are critical components of data management practices. Given the increasing concerns over data breaches and the misuse of sensitive information, researchers must prioritize these aspects.
Adhering to data protection regulations, such as GDPR or HIPAA, enhances trust among research participants and stakeholders. Implementing comprehensive security measures is imperative. Start with encrypting data at rest and in transit to protect against unauthorized access.
Regular audits of data access and usage can further safeguard sensitive information, ensuring only authorized individuals have access to critical datasets. Moreover, data anonymization techniques can mitigate privacy risks by dissociating personal identifiers from the collected data. This practice enables researchers to utilize datasets without infringing on individual privacy rights.
"Effective data management ensures not only the integrity of research outcomes but also the ethical treatment of data throughout its lifecycle."
In summary, integrating sound data management practices into research enhances efficiency, data quality, and compliance with ethical standards. As the landscape of research continues to evolve, adopting innovative storage solutions and prioritizing security will be key to fostering sustainable data use and management.
Ethics in Data Usage
Data ethics plays a vital role in ensuring that research practices uphold fundamental principles of integrity and fairness. In the rapidly evolving landscape of data collection and analysis, it is crucial to establish guidelines that govern the conduct of researchers, organizations, and institutions in their data-related activities. This section will examine the importance of ethical standards and explore several case studies illustrating the consequences of ethical breaches.
Importance of Ethical Standards
Ethical standards serve as a foundation for trust in data usage. They guide researchers in making informed decisions about how to handle data, ensuring fairness, accountability, and transparency. Several key elements underscore the importance of these standards:
- Privacy Protection: Safeguarding personal information is a requisite for ethical research. Researchers must ensure informed consent, allowing participants to understand how their data will be used.
- Data Integrity: Ethical standards mandate accuracy in data representation. Misleading data can skew results, impacting scientific progress and public perception of research.
- Responsible Sharing: Ethical guidelines inform how and when data should be shared. Researchers must balance openness with the need to protect sensitive information.
- Bias Prevention: Recognizing and mitigating biases in data collection and analysis is crucial. Researchers must strive for inclusivity and representativeness in their work, thereby enhancing public trust.
"Ethics in data usage is not just a guideline, but a necessity to protect the dignity and rights of individuals involved in research."
Incorporating these ethical considerations not only improves the quality of research outcomes but also fosters a culture of respect for social responsibility among researchers.
Case Studies on Ethical Breaches
Understanding the implications of ethical breaches is essential to promoting better practices in research. Here are notable examples that illustrate the consequences of abandoning ethical standards:
- The Volkswagen Emissions Scandal: This case revealed how data manipulation can lead to widespread deception. Volkswagen used software that altered emissions data, leading to legal repercussions and a damaged public trust. It serves as a reminder that ethical lapses can have far-reaching consequences beyond immediate results.
- Duke University and the STAP Cells Controversy: Researchers at Duke published findings on stem cells, later found to be fraudulent. The ethical breach of misrepresenting data not only damaged reputations but also stunted scientific progress in that area. Subsequently, it highlighted the need for rigorous peer review and ethical scrutiny in publishing.
- Facebook and Cambridge Analytica: This incident showcased extensive misuse of user data without consent, sparking global outrage and significant regulatory changes. It emphasizes the critical importance of transparency and user rights in data use.
In summary, the exploration of ethical standards and breaches in data usage reveals the complexities involved in conducting responsible research. By adhering to established guidelines, researchers can protect their integrity while also ensuring that the advancement of knowledge is rooted in respect for individuals and communities.
Interpretation and Analysis of Data
Interpretation and analysis of data are crucial steps in the research process. These stages convert raw data into meaningful information. For researchers, uncovering trends, drawing conclusions, and making informed decisions hinges on how they interpret and analyze data. Understanding the myriad ways in which data can be analyzed enables more robust research outcomes.
Effective analysis often employs statistical methods to summarize and interpret data correctly. These methods help researchers identify patterns, correlations, and significant differences between groups. Statistically sound analysis minimizes bias and enhances reliability, thereby supporting the validity of conclusions drawn from the data. Moreover, applying the correct statistical techniques is vital. Each method requires a context where it can shine, be it descriptive statistics to summarize data, inferential statistics to draw conclusions, or multivariate analysis to understand complex relationships.
Another important aspect of data interpretation is the ability to effectively visualize the findings. Data visualization techniques transform complex datasets into graphical representations, making them easier to comprehend. Graphs, charts, and diagrams can communicate trends and relationships more clearly than numbers alone. This clarity is incredibly beneficial when presenting findings to diverse audiences, as effective visualizations engage readers while providing insights at a glance.
"The purpose of data visualization is to communicate information clearly and efficiently to users. A well-chosen visual can help uncover trends and insights that may not be immediately obvious in raw data."
Successful interpretation and analysis hinge on a few essential considerations:
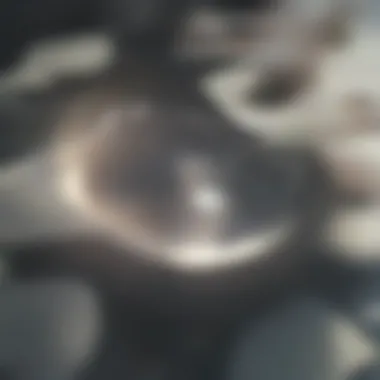
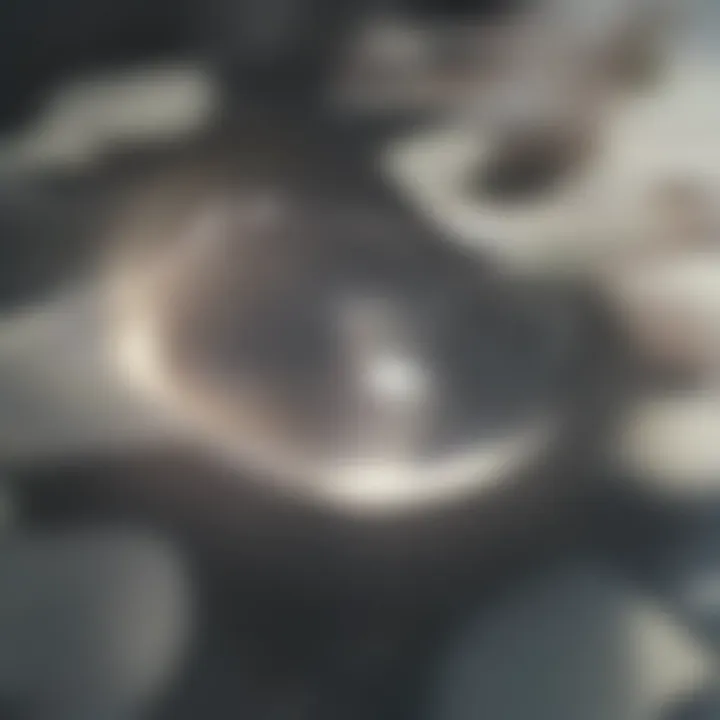
- Understanding the context of the data collected.
- Choosing appropriate analytical methods.
- Presenting results in a clear, concise format.
- Being aware of ethical implications in the conclusions drawn.
Researchers must continuously refine their analytical skills and draw from new techniques in statistics and visualization. By doing so, they can better address complex questions in their fields. This continual learning is not just theoretical; it necessitates practical application within various disciplines to maintain relevance in a rapidly evolving landscape.
Impact of Data on Scientific Advancements
Data has transformed scientific research in many ways. It acts as the backbone for hypothesis testing and validation. In scientific advancements, data helps to establish patterns, predict outcomes, and inform decisions. Innovation relies on accurate, timely data, making it crucial in developing new technologies and understanding complex systems.
Case Studies in Various Disciplines
Biology
In biology, data significantly impacts fields such as genomics and ecology. The use of large datasets has led to breakthroughs in understanding genetic diseases. One key characteristic of biology is its focus on living organisms, making it a dynamic choice for this article. The unique feature of biological data is its variability, which can be both an advantage and a disadvantage. The variability provides a rich source for analysis, but it also poses challenges in standardization and comparison across studies.
Chemistry
Chemistry utilizes data to reveal chemical behaviors and relationships. The contribution of data in chemistry is seen in areas such as drug discovery and material science. A key characteristic of chemistry is its quantitative nature. This numeric focus makes it a beneficial choice for this exploration. The unique aspect of chemistry is the detailed quantitative analysis, leading to precise predictions. This precision can enhance understanding but may also lead to over-reliance on quantification alone, neglecting qualitative insights.
Physics
Physics relies heavily on data to validate theories and models. It is essential in areas like particle physics and astrophysics. A significant feature of physics is its foundational principles, which guide experimental designs. This makes physics a popular choice for investigating data's impact. The rich data sets in physics can lead to groundbreaking discoveries. However, complexities in the data can lead to misinterpretation if not analyzed correctly.
Earth Sciences
Earth sciences draw on data to study climatic and geological systems. The contribution of data here is critical for understanding environmental changes. One key characteristic of earth sciences is the interdisciplinary approach, combining aspects of geography, geology, and meteorology. This broad scope makes it an important field for this article. A unique facet of earth sciences is the integration of historical data with current readings. This approach helps in predicting future trends but can face challenges in data reliability due to environmental variability.
Innovations Driven by Data
Data-driven innovations have reshaped numerous industries, particularly in health, technology, and energy sectors. The continuous collection and analysis of data lead to enhanced efficiency and effectiveness in systems. These innovations often result from collaborations between various scientific disciplines, fostering environments that prioritize problem-solving through data. For instance, in healthcare, data analytics have improved patient outcomes through personalized medicine. Similarly, in technology, machine learning depends heavily on data to enhance software performance.
"Data itself is a backbone, an infrastructure for knowledge development and innovation across many scientific fields."
In summary, data's impact on scientific advancements is profound and multifaceted. It drives innovation, informs practical applications, and enhances our understanding of the world around us. As the landscape of data continues to evolve, its role in advancing science becomes even more critical.
Future Trends in Data Management for Research
The evolving landscape of data management presents numerous opportunities. Researchers must adapt to these changes for effectiveness and innovation. This section outlines significant trends influencing data management in research, focusing on the integration of technology and broader accessibility.
Artificial Intelligence and Data
Artificial Intelligence, or AI, offers profound impacts on data management. The automation of data processing enhances efficiency. AI can analyze vast datasets swiftly, identifying patterns often missed by human analysts. This capability leads to more thorough insights. For example, machine learning algorithms can classify data more accurately. Researchers can also use AI tools to predict outcomes based on historical data.
However, the implementation of AI must be approached with caution. Data quality remains crucial. Poor quality datasets can lead to misleading conclusions. Therefore, valid and comprehensive data should be prioritized. As AI continues to evolve, redefining methodologies in data analysis is essential for valid scientific research.
"AI facilitates new dimensions of exploration in data, yet requires a basis of integrity in data collection."
Additionally, AI could enhance collaborative efforts by integrating disparate datasets. This function improves accessibility to information. Researchers from different disciplines can combine findings, advancing knowledge beyond traditional silos. One challenge is ensuring that these systems are transparent. Stakeholders must understand how AI-generated insights are derived to trust their validity.
Open Data Initiatives
Open Data Initiatives promote accessibility and transparency. Researchers share their datasets openly, allowing others to use and analyze them. This practice fosters innovation and reproducibility. When datasets are available, it enables collaborative research across borders and disciplines.
Such practices can lead to significant discoveries. For instance, researchers in health sciences can access datasets from various studies. This cross-pollination enhances understanding of complex issues, like disease outbreaks.
However, with open data comes ethical considerations. Data privacy must be maintained, even when sharing datasets. Researchers should anonymize data to protect individual identities. Thus, a balance between openness and ethical responsibility is vital.
Following these trends, institutions are encouraged to develop policies that support open data while ensuring protections are in place. Effective management of open data initiatives can lead to a culture of cooperation in the scientific community. Institutions like governments and universities can significantly contribute by establishing frameworks.
By aligning efforts with Open Data Initiatives, the future of research will likely see increased collaboration, innovation, and advancement across multiple disciplines, enhancing the overall impact of scientific inquiry.