Exploring the Fusion of Biotechnology and AI
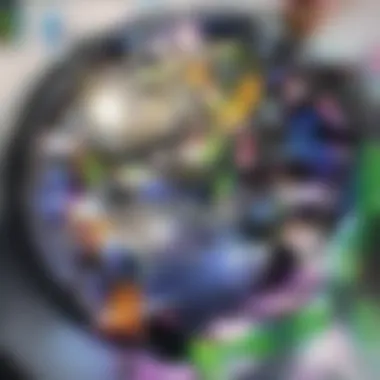
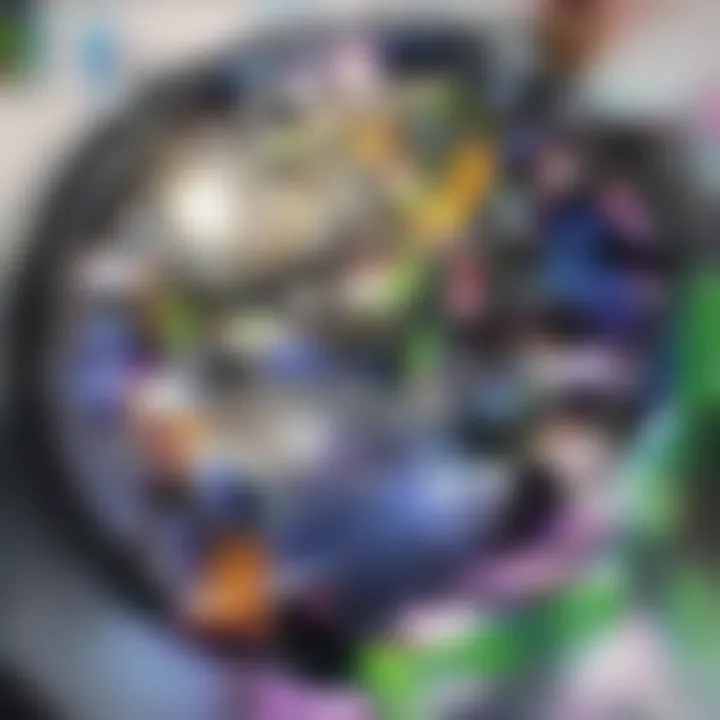
Intro
The convergence of biotechnology and artificial intelligence is an exciting and rapidly evolving field. This interaction fundamentally reshapes how researchers approach problems in both domains. Biotechnology focuses on harnessing biological processes and systems for innovative applications, while artificial intelligence brings advanced computational techniques that can analyze vast data sets. Together, they offer numerous possibilities that advance science and benefit society.
As these two areas intersect, it is important to evaluate the innovations and methodologies being employed. Advances range from bioinformatics to precision medicine, creating a more responsive and efficient scientific landscape. Ethical considerations, however, also emerge as we develop these powerful tools. Understanding the implications is crucial for researchers and practitioners in both fields.
The following sections will delve deeper into the essential findings, methodologies, and insights that characterize this integration. It aims to serve as a thoughtful dialogue among professionals, educators, and students interested in this transformative topic.
Prelims to Biotechnology and Artificial Intelligence
The integration of biotechnology and artificial intelligence (AI) represents a significant shift in the scientific landscape. As we delve into this subject, it becomes clear that the marriage of these two fields offers not only innovative solutions but also a path towards greater efficiency and productivity in research. This article aims to explore the various aspects of this intersection, highlighting how such collaborations can enhance our understanding and manipulation of biological systems.
Biotechnology focuses on manipulating biological processes for various applications, including health, agriculture, and environmental sustainability. In recent years, the advent of AI has provided tools that can analyze vast amounts of data, predict outcomes, and streamline workflows. This synergy allows for a more profound comprehension of biological phenomena, ultimately leading to breakthroughs that were previously unimaginable.
Integrating AI into biotechnological applications fosters rapid advancements in drug development, genomic studies, and personalized medicine. However, this integration does present considerations regarding data management and ethical implications that cannot be overlooked. Understanding these nuances is crucial for professionals and researchers navigating this evolving field.
Therefore, by examining biotechnology and AI together, we can appreciate their combined power in transforming research methodologies and discovering new avenues for innovation. The following sections will unpack critical components of biotechnology, AI, and their interconnection.
Current Applications of AI in Biotechnology
The integration of artificial intelligence (AI) within biotechnology has revolutionized how research and development is conducted. This merging of technologies holds promise not only for enhancing scientific discovery but also for improving processes and outcomes in various biotechnological fields. AI's ability to analyze vast amounts of data efficiently allows researchers to obtain insights that were previously unattainable. The applications examined in this section showcase the incredible potential of AI in driving advancements in biotechnology.
Drug Discovery and Development
AI plays a pivotal role in drug discovery and development. Traditional methods are often time-consuming and costly, frequently taking years to bring a drug from concept to market. However, utilizing AI technologies can significantly accelerate this timeline.
One prevalent application is the identification of potential drug candidates through machine learning algorithms. These algorithms analyze molecular structures and biological data, allowing researchers to predict which compounds are most likely to succeed in treating specific conditions. Furthermore, platforms like Atomwise leverage AI to screen millions of compounds, targeting proteins and predicting their effectiveness against diseases like cancer and Alzheimer's. This not only optimizes the drug development process but also decreases the likelihood of failures in later stages.
Genomic Data Analysis
The vast amount of data generated in genomics presents both challenges and opportunities. AI techniques facilitate genomic data analysis, enabling researchers to identify patterns and correlations that would be difficult for humans to detect. This application is especially vital for personalized medicine, where understanding an individual's genetic makeup can lead to more tailored treatment plans.
Using AI, scientists can process genomic sequences rapidly. Tools such as DeepVariant utilize deep learning to call variants in genomic data with high accuracy. As a result, researchers can have a clearer understanding of genetic disorders and the development of therapies based on specific gene expressions. This emphasis on precision is transforming how genetic data is interpreted and utilized in clinical settings.
Synthetic Biology
Synthetic biology stands at the frontier of biotechnology, merging biology with engineering principles. AI enhances design and optimization processes in synthetic biology by modeling complex biological systems. These are often variables are difficult to quantify due to their complexity.
AI algorithms can simulate various genetic modifications, allowing researchers to predict the outcomes before they are implemented experimentally. This application helps to reduce trial and error in laboratory settings. Moreover, tools like Ginkgo Bioworks employ AI to design custom microbes for specific applications, such as biofuels or pharmaceuticals, showcasing a forward-thinking approach to biological engineering.
Personalized Medicine
The shift towards personalized medicine is greatly aided by AI technologies. By analyzing patient data, including genetic information, medical history, and environmental factors, AI can help predict how individual patients will respond to different treatments.
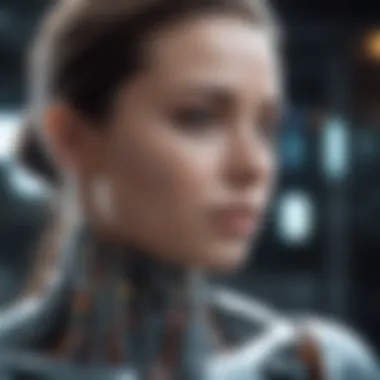
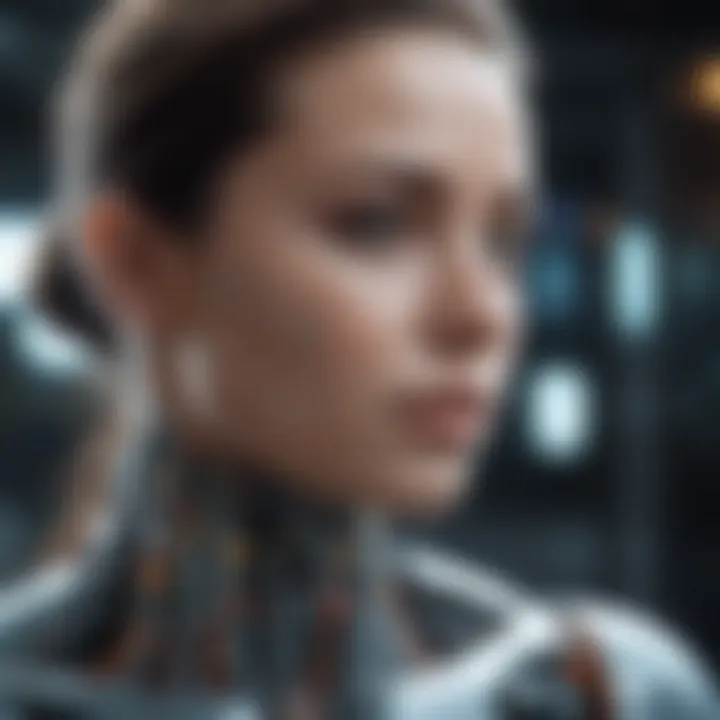
Machine learning models can identify associations between specific genetic markers and treatment outcomes, guiding doctors in selecting the most effective therapies for their patients. Additionally, companies like Tempus utilize AI to analyze clinical and molecular data to support oncologists in developing personalized cancer treatment plans. This tailored approach has the potential to improve patient outcomes and minimize side effects.
AI Technologies Transforming Biotechnology
The convergence of artificial intelligence (AI) and biotechnology presents groundbreaking opportunities for advancements in multiple domains. AI technologies play a crucial role in reshaping how biological data is interpreted, analyzed, and utilized. These technologies streamline complex processes, augment decision-making, and foster significant innovations. The integration of AI enriches biotechnology by enhancing productivity, speeding up research timelines, and enabling far-reaching applications across fields such as drug development, genomics, and quality control in manufacturing. In essence, the application of AI technologies transforms traditional biotechnological operations into more efficient and formidable systems.
Machine Learning in Biotech
Machine learning (ML) is a subset of AI that focuses on systems that learn from data to improve their performance over time without explicit programming. In the field of biotechnology, machine learning algorithms analyze vast datasets produced in experiments. For instance, in drug discovery, ML can identify potential drug candidates by analyzing chemical structures and biological data. This role is essential for predicting molecular interactions, which are often too complex for traditional computation methods. Furthermore, the ability of ML to recognize patterns in genetic data contributes significantly to genomics research, helping scientists understand disease traits and mechanisms.
By applying machine learning, researchers can reduce the time it takes to discover and develop new therapies, significantly impacting patient outcomes.
Natural Language Processing for Research
Natural Language Processing (NLP) is another powerful AI technology that assists researchers in managing large volumes of literature and data. NLP can extract meaningful information from research papers, clinical trial reports, and patents. This capability is particularly crucial in biology, where the amount of published data is expanding exponentially. Researchers can utilize NLP algorithms to streamline the literature review process, thus focusing more on innovation rather than information retrieval. Moreover, NLP enables better communication of complex findings to broader audiences, improving collaboration across disciplines.
Computer Vision for Quality Control
Computer vision, as an AI technology, assists in improving the quality control processes in biotechnology. It uses image processing techniques to automate the inspection of biological samples and products. For example, computer vision can identify abnormalities in cell culture or detect contamination in biopharmaceutical products. This technology allows for real-time monitoring, ensuring that only high-quality products reach the market. It provides significant cost savings by reducing the reliance on manual inspections, which are often prone to human error. Therefore, computer vision plays a vital role in maintaining regulatory compliance and enhancing product safety in the biotech industry.
Ethical Considerations in Biotech and AI Integration
The integration of artificial intelligence with biotechnology poses unique ethical challenges. As AI systems become more embedded in biotech processes, it is crucial to assess the potential consequences of their use. Ethical considerations are not just an afterthought; they are fundamental to ensuring responsible innovation. It is important to recognize that while AI can enhance efficiency, automate complex tasks, and drive discoveries, it also raises issues such as data privacy, the ramifications of genetic modification, and responsibility for AI-driven decisions.
Data Privacy Issues
Data privacy is a primary concern when AI is employed in biotechnology. Biotech research often involves sensitive data, such as genetic information and medical histories. The use of AI algorithms necessitates access to large datasets, which can be susceptible to breaches. Ethical frameworks should emphasize the need for stringent data protection measures to maintain confidentiality. It is essential for researchers to ensure that data is anonymized and secure. Moreover, users of AI in biotechnology must comply with regulations like the Health Insurance Portability and Accountability Act (HIPAA) to avoid potential misuse of personal data.
Ethical Implications of Genetic Modification
The application of AI in genetic modification offers tremendous potential to enhance agricultural yields and produce disease-resistant crops. However, the ethics of modifying genomes elicits significant debate. Concerns arise regarding biodiversity, ecosystem balance, and unintended consequences. Society will have to grapple with questions of what constitutes ethical genetic intervention. Responsible stewardship is vital. Therefore, researchers must engage with the public and policymakers in discussions on implications of genetic modification, fostering transparency and trust within communities.
AI Decision-Making Responsibilities
The increasing reliance on AI for decision-making in biotechnology raises questions about accountability. When AI systems are tasked with making decisions in clinical settings or research environments, who is responsible if things do not go as planned? It is imperative to establish clear guidelines that define the roles and responsibilities of humans versus machines. Researchers and practitioners should understand that while AI provides recommendations, human oversight remains essential. AI must assist, not replace, human judgment in crucial decisions. This ensures a necessary balance between technological advancements and ethical considerations.
"Balancing innovation with ethical considerations is essential for sustainable progress in the biotech sector."
As biotechnology and AI continue to evolve, maintaining an ongoing discourse about these ethical implications is critical. Engaging stakeholders from diverse backgrounds can enrich the understanding of potential risks and benefits. Involving ethicists, scientists, government bodies, and the public will lead to more comprehensive and responsible applications of AI in biotechnology.
Impact of AI on Research and Development Processes
The integration of artificial intelligence (AI) has fundamentally transformed research and development (R&D) processes in biotechnology. AI's capabilities enable researchers to better analyze vast datasets, thus accelerating the pace of discovery and enhancing the quality of insights. This intersection of technologies presents opportunities to improve productivity, reduce costs, and foster innovation in ways that were previously considered impractical or impossible. Understanding these elements is critical for stakeholders in the field as they navigate this evolving landscape.
Accelerating Research Timelines
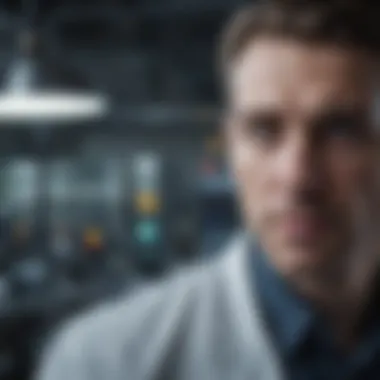
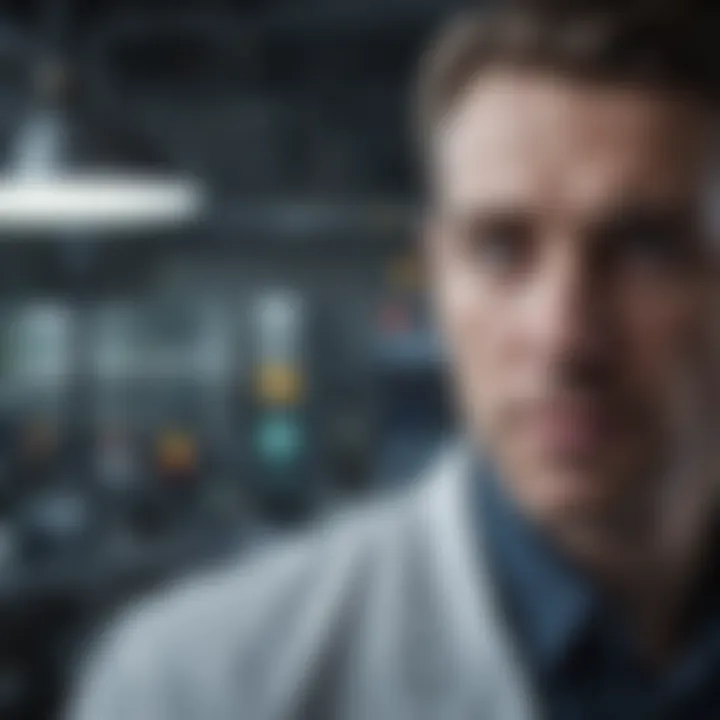
AI applications significantly shorten the timelines associated with biotech research. In traditional settings, lengthy research phases can delay crucial discoveries. With AI, tasks such as data interpretation and hypothesis testing become more efficient. Machine-learning algorithms can process large volumes of genetic and biochemical data far quicker than human researchers alone.
Moreover, AI-driven automation facilitates high-throughput screening, allowing for faster identification of viable drug candidates. This speed not only saves money but also has the potential to bring life-saving treatments to market more rapidly. As AI continues to improve, its role in expediting timelines will only become more pronounced, enabling a faster response to emerging health challenges.
Enhancing Experimental Design
AI is reshaping experimental design in biotechnology research. It allows for sophisticated modeling that predicts outcomes based on various genetic and environmental factors. Researchers can simulate experiments using AI tools, which helps in understanding potential results before practical implementation.
By leveraging algorithms that analyze past experiment data, researchers can refine their experimental processes, identifying the most promising paths while minimizing waste of resources. This predictive capability not only enhances the robustness of research but also ensures a more strategic allocation of effort, leading to more successful experiments.
Facilitating Collaborative Innovation
Collaboration in biotech research is crucial, and AI plays a vital role in fostering this collaboration. Utilizing platforms powered by AI, researchers can easily share insights and findings with a global community. Integrative data platforms facilitate knowledge exchange, breaking silos that often limit innovation.
Collaborative tools enabled by AI not only promote information sharing but also enhance interdisciplinary research, combining expertise from different fields to drive innovation.
Moreover, AI helps identify potential collaborators whose work aligns with a researcher's goals. As researchers increasingly depend on partnership, AI serves as both a connector and a facilitator of diverse skill sets and perspectives, enriching the overall research environment.
In summary, the application of AI in research and development processes within biotechnology is not just a trend; it represents a critical shift. By accelerating timelines, enhancing experimental designs, and facilitating collaboration, AI is setting a new standard for how biotechnological research is conducted and ultimately impacting outcomes in profound ways.
Challenges and Limitations
Understanding the challenges and limitations at the crossroads of biotechnology and artificial intelligence is crucial for both current and future advancements. These barriers can significantly affect the implementation and efficiency of AI in biotechnological processes. Addressing these concerns helps to navigate potential issues and optimize the integration of these transformative technologies.
Data Limitations and Quality Control
Data is the backbone of both biotechnology and AI. However, challenges related to data limitations can hinder progress. When datasets are either too small or not representative, the resulting AI models may yield inaccurate results. In biotechnology, this can lead to flawed insights, which in turn impacts research outcomes. Quality control becomes an even more pressing issue, as the integrity of data directly influences the success of machine learning algorithms. If the data contains errors, biases, or inconsistencies, the downstream applicationsโincluding drug discovery and personalized medicineโmay face significant setbacks.
Using reliable data sources is essential. This involves rigorous validation procedures to ensure that the input data is of high quality. Furthermore, continuous monitoring of data quality must be implemented throughout the research lifecycle to ensure that any changes in data collection practices do not compromise findings.
Integration of AI Systems
Integrating AI systems into existing biotechnological frameworks presents its own set of complexities. Organizations often face compatibility issues where new AI technologies do not seamlessly fit with legacy systems. This challenge can lead to operational inefficiencies and increased costs.
Moreover, the skill set required to operate advanced AI systems may not always be readily available within a biotechnology organization. Training personnel or hiring experts can be resource-intensive.
Despite these obstacles, successful integration can result in substantial gains. AI can optimize workflows, increase speed, and enhance the accuracy of experiments. Being aware of potential integration issues and planning for them can help mitigate risks and establish more effective collaboration between biotechnology and AI technologies.
Regulatory Hurdles
The regulatory landscape surrounding biotechnology and artificial intelligence is complex and often vague. Different regions can impose varying regulations, causing additional challenges for researchers and companies attempting to comply.
For example, guidelines concerning genetic modification and data privacy can differ significantly across countries. Navigating these rules requires time and resources, often leading to delays in product development and the introduction of innovations to the market.
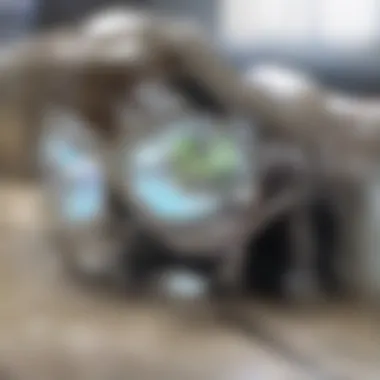
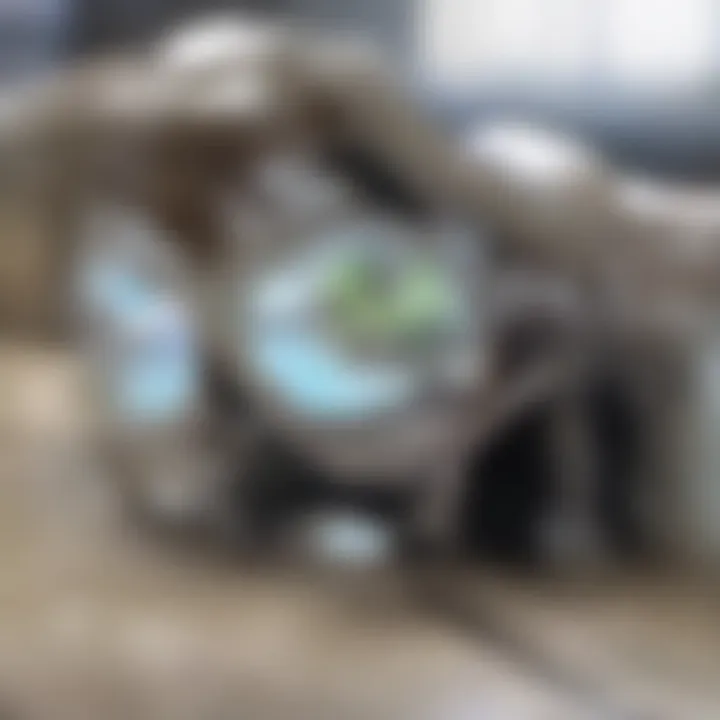
"Understanding and adapting to regulatory demands is essential for aligning AI applications with biotechnology practices."
Researchers and companies must stay informed about the legal environment relevant to their work. Engaging with regulatory bodies early in the research process can provide clarity and help streamline approval processes. Collaboration with legal experts who specialize in both fields can also help to alleviate uncertainty and facilitate compliance.
In summary, addressing these challenges and limitations is vital for the effective integration of AI into biotechnology. Recognizing the importance of data quality, successful integration, and regulatory considerations enables stakeholders to strategically navigate the complexities involved.
Future Directions of AI in Biotechnology
The integration of artificial intelligence with biotechnology represents a frontier filled with potential. This intersection is crucial for evolving research methodologies and improving the efficacy of biotechnological applications. Understanding future directions in this field helps stakeholders to anticipate challenges and harness opportunities that arise from new technological advancements.
Emerging Technologies
Emerging technologies are leading the charge in the evolution of biotech-enhanced AI applications. Techniques such as deep learning are being refined to analyze complex biological datasets more efficiently. For instance, companies like DeepMind are exploring how advanced neural networks can predict protein structures with unprecedented accuracy. This can revolutionize drug design and personalization in medicine.
Additionally, CRISPR technology is evolving in tandem with AI, enabling more precise gene editing capabilities. Machine learning algorithms will assist in identifying potential off-target effects, which is vital for ensuring safety in genetic modifications. Tools that employ AI for simulating biological processes also show promise, helping researchers visualize outcomes before executing experiments. This combination accelerates the pace of discovery significantly.
Long-term Research Trends
As we peer into the future, long-term research trends indicate a shift toward collaborative intelligence, where AI and human researchers work side-by-side. This partnership could help break down silos in research disciplines, fostering interdisciplinary approaches. Biotechnological advancements in areas such as regenerative medicine and microbiome studies are increasingly reliant on data-driven decisions. Thus, the cultivation of AI tools that standardize and analyze diverse datasets will be essential.
Moreover, synthetic biology continues to gain traction and will likely see increased AI integration. The ability to design and construct new biological parts will depend heavily on AI's analytic capabilities. Trends show a growing interest in biomimicry, where nature is emulated in technological solutions. AI can aid in understanding these natural systems more profoundly, leading to innovative applications.
The Role of Public Policy
Public policy plays a pivotal role in framing the landscape for AI in biotechnology. Policymakers must engage with scientists and industry leaders to create guidelines that not only drive innovation but also protect public interests. Developing frameworks for responsible use of AI in biotechnological applications is crucial. This includes addressing ethical considerations, regulatory compliance, and data governance.
Furthermore, effective public policy can streamline the approval of AI-driven biotechnological products, ensuring they meet safety and efficacy standards. This is essential as the speed of technological advancements can sometimes outpace existing regulations. Encouraging open dialogue between government entities, biotech firms, and research institutions will foster an environment where responsible innovation can thrive.
In summary, the future directions of AI in biotechnology promise advancements that could reshape health care, environmental management, and various industries. Embracing emerging technologies, understanding long-term research trends, and establishing sound public policy will be fundamental in navigating this transformative landscape.
Ending
First, the ongoing integration of AI technologies into biotechnology increases the efficiency and accuracy of research. For instance, AI algorithms can process large datasets significantly faster than human counterparts. This capability is essential in fields such as genomic data analysis and drug discovery, where understanding complex biological information quickly is paramount.
Second, the ethical considerations surrounding biotechnological applications of AI merit attention. As AI systems become more integral to decision-making processes in biotechnology, careful consideration of data privacy, accountability, and the implications of genetic modifications is necessary. Ensuring ethical standards is vital in maintaining public trust and fostering responsible innovation.
Thirdly, the article emphasizes the future directions that AI can steer biotechnology. Emerging technologies, long-term research trends, and the role of public policy will shape this evolving landscape. Actively engaging with these aspects will help in navigating the challenges and harnessing the potential benefits that AI offers.
Overall, the integration of AI into biotechnology has profound implications. It enhances productivity and enables groundbreaking discoveries. Through thoughtful discourse and continued research, this integration can pave the way for the next generation of biotechnological innovation.
Summarizing Key Insights
In summary, we explored various dimensions of the convergence between biotechnology and artificial intelligence. Key insights include the remarkable advancements that AI brings to drug discovery, genomic analysis, and personalized medicine. Additionally, the article highlighted the ethical challenges that arise from such integrations, specifically relating to data privacy and decision-making.
Furthermore, the potential future directions of this field reveal an exciting horizon filled with possibilities. The landscape is rapidly changing, with new technologies continually emerging and reshaping our understanding of both biotechnology and AI.
Encouraging Further Discussion
As we conclude, it is imperative to encourage further discussion on the implications of AI in biotechnology within academic settings, research communities, and policy-making forums. Engaging diverse perspectives facilitates a comprehensive examination of the challenges and opportunities presented in this field. By fostering inclusive conversations, stakeholders can collaboratively navigate this intricate landscape, addressing ethical considerations and determining best practices that will govern this transformative relationship.
Moreover, platforms such as Reddit and Facebook can serve as valuable resources for sharing knowledge and insights among professionals, researchers, and enthusiasts alike. Such discussions can stimulate new ideas and collaborative projects, paving the way for revolutionary advancements.