Transforming Healthcare with Big Data Analytics
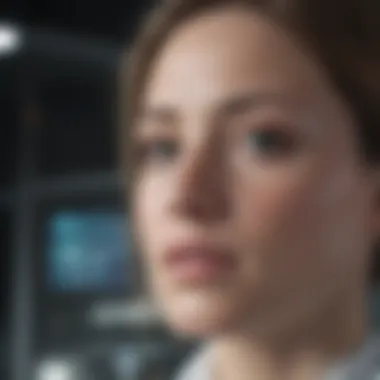
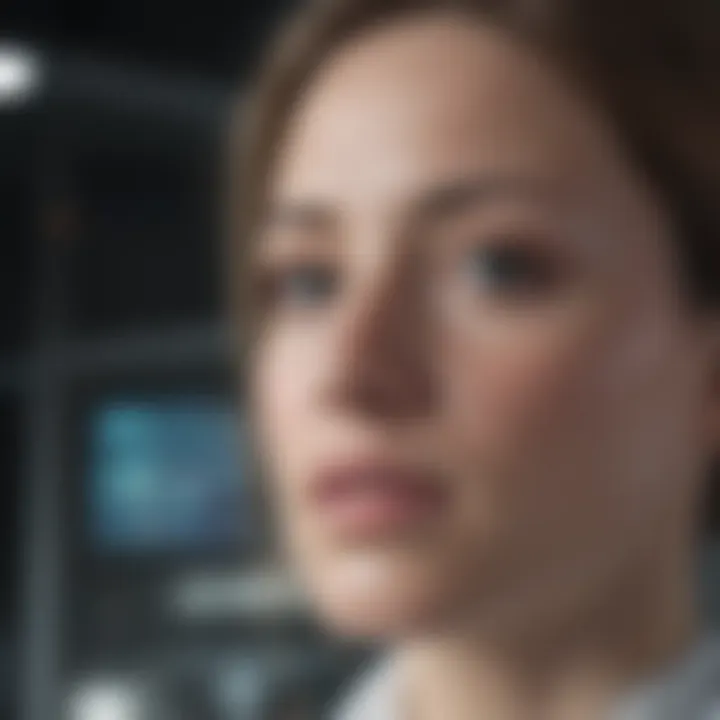
Intro
Research Overview
The studies addressing big data in healthcare analytics reveal several key findings regarding its impact on healthcare delivery and research methodologies. The integration of large datasets helps healthcare professionals to make informed decisions and to enhance patient outcomes. By leveraging data from electronic health records, wearables, and other sources, researchers can discover patterns that inform interventions at a population level.
Summary of Key Findings
- Improved Patient Care: Big data allows for personalized treatment plans based on the unique needs of patients, thus improving their overall care.
- Predictive Analytics: Utilizing predictive models can help anticipate future health issues, allowing for preventative actions.
- Enhanced Research Capabilities: Researchers can analyze vast datasets to identify trends and develop new treatment methodologies that can lead to significant advancements.
Methodologies Employed
- Machine Learning Algorithms: These are essential in analyzing data patterns and making predictions about patient outcomes.
- Natural Language Processing (NLP): NLP techniques allow for the extraction of meaningful information from unstructured text within health records.
- Data Mining Techniques: These are used to discover insights from large datasets, facilitating the identification of correlations and trends.
In-Depth Analysis
Exploring the results from big data implementation in health analytics gives insights into how these approaches have changed the healthcare landscape.
Detailed Examination of Results
The application of big data analytics has produced several significant results that warrant discussion. The findings indicate that health organizations that adopt big data strategies see a reduction in both operational costs and patient readmission rates. Moreover, organizations integrating these models report improved patient satisfaction and engagement.
Comparison with Previous Studies
Compared with earlier studies that relied on smaller datasets, recent research utilizing big data illustrates a broader context and deeper insights. Traditional approaches often focused on a singular treatment or condition, whereas big data allows for a holistic understanding of patient populations. Analyzing trends across various demographics and health conditions reveals the nuanced interactions that conventional methods often overlook.
"Big data analytics in healthcare provide not just insights, but actionable intelligence that can revolutionize treatment and patient engagement strategies."
End
Big data comprises vast volumes of structured and unstructured information generated from various sources, especially within healthcare. These include patient records, diagnostic tests, genomic data, and even social media interactions related to health. Analyzing this data can lead to more effective treatment protocols, personalized medicine, and informed preventative strategies. Thus, the implications of utilizing big data in health analytic are profound and far-reaching.
Moreover, the rise of data-driven decision making marks a shift from instinctive judgments based on limited information to decisions backed by extensive evidence. Healthcare professionals, now more than ever, can make informed choices about patient care, resource allocation, and operational workflow.
The advent of advanced analytical techniques further empowers practitioners. With proper tools and methodologies, healthcare providers can extract meaningful insights from raw data, ultimately improving the quality of care. However, recognizing the challenges inherent in big data—a blend of data privacy concerns and technical integration—is crucial. Each evolution within this domain carries implications not just for care providers, but importantly, for patients as well.
Understanding these dynamics shapes the discourse on health analytics. As research continues to unfold, the significance of big data in enhancing healthcare delivery cannot be overstated. The sections that follow will delve into the nuances of this integration, articulating its definition, impact, data sources, challenges, and future trends.
Defining Big Data in Healthcare
Understanding what constitutes big data in healthcare is crucial for grasping its transformative potential in the sector. This section emphasizes defining big data specifically within the healthcare context. It helps in identifying how various characteristics interplay, enhancing operations and patient outcomes.
Characteristics of Big Data
Volume
Volume refers to the immense quantity of data generated in healthcare. It is estimated that the total volume of health data is growing at an unprecedented pace. The large-scale collection of data from sources like Electronic Health Records, genomic sequencing, and medical imaging is a prominent feature. This vast quantity is essential because it provides a rich dataset for analysis. However, managing such a large volume also comes with challenges, such as storage and processing capabilities. Therefore, while volume can offer deep insights, indadequate infrastructure may limit its effectiveness in health analytics.
Velocity
Velocity pertains to the speed at which data is generated, processed, and analyzed. In healthcare, data is often collected in real-time. For instance, wearable devices continuously collect patient data like heart rates or glucose levels. This rapid influx allows for timely interventions and improves patient management. Consequently, the ability to quickly analyze and respond to this data can significantly improve health outcomes. However, high velocity also necessitates robust data processing systems to avoid lag in decision-making, which could be detrimental in critical scenarios.
Variety
Variety reflects the different forms that data can take. Within healthcare, data comes in structured forms, like databases, as well as unstructured forms, like notes from doctors or patient reviews. This diversity enriches analytics. It allows for more comprehensive insights, considering various factors that contribute to patient health. However, integrating diverse data types poses challenges in standardization and analysis. Ensuring that all these forms contribute meaningfully to patient care remains an ongoing challenge.
Veracity
Veracity deals with the quality and trustworthiness of data. High-quality data is essential for making informed decisions in healthcare. Accurate patient data leads to better diagnoses and treatments. Inaccuracies, however, can lead to harmful consequences. Thus, ensuring data accuracy and establishing protocols for data validation is desirable. Though assessing veracity can be resource-intensive, it is critical for maintaining integrity in health analytics.
"Effective use of big data in healthcare hinges upon understanding and managing its fundamental characteristics."
In summary, defining big data in healthcare revolves around these four key characteristics: volume, velocity, variety, and veracity. Each of these aspects plays a vital role in the utility of big data, impacting how healthcare professionals can leverage analytics to improve patient care and operational efficiency.
The Impact of Big Data on Healthcare Delivery
The integration of big data in healthcare delivery has transformative potential. It reshapes existing paradigms and introduces new methodologies for treating patients. This section examines how big data contributes to enhanced patient care and operational efficiency, both critical aspects of healthcare delivery.

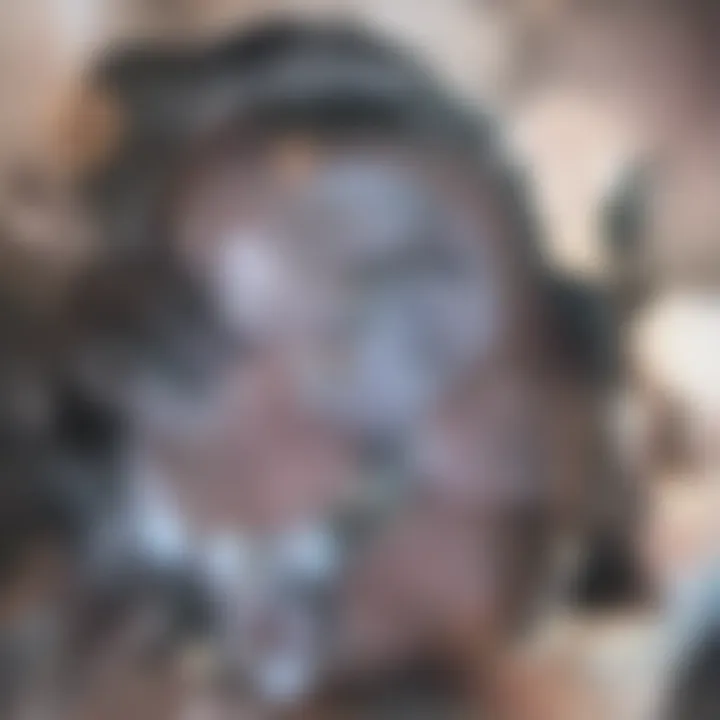
Enhancing Patient Care
In the realm of patient care, big data plays a crucial role. It allows healthcare providers to tailor treatments based on patient-specific data.
Personalized Medicine
Personalized medicine offers tailored medical treatments that consider individual patient characteristics. This approach is advantageous as it maximizes treatment efficacy by focusing on the unique genetic and physiological traits of patients. Personalized medicine can lead to better patient adherence and improved outcomes. With big data, healthcare providers can analyze large datasets to identify effective treatment strategies, paving the way for customized care pathways. However, challenges persist, such as the need for comprehensive genomic databases which can be resource-intensive to develop and maintain.
Preventative Strategies
Preventative strategies aim to reduce disease incidence before it occurs. The integration of big data significantly enhances the ability to identify at-risk populations and develop targeted interventions. By analyzing historical data, healthcare organizations can predict health trends, allowing for timely preventive measures that can save time and resources in the long run. This proactive approach is favored in the current healthcare landscape, yet it requires ongoing commitment and support from various stakeholders to ensure effectiveness.
Data-Driven Decision Making
Data-driven decision making represents a shift in how healthcare professionals make choices regarding patient care. Instead of relying solely on clinical experience or intuition, they can utilize extensive datasets to guide their decisions. This characteristic promotes transparency and accountability in healthcare outcomes. While it can enhance effectiveness, the reliance on data also raises concerns about over-dependence on technology, which might overlook the nuances of individual patient needs.
Operational Efficiency
Beyond patient care, operational efficiency is a game changer within healthcare systems. Big data enables organizations to streamline processes and enhance overall service delivery.
Resource Allocation
Resource allocation involves distributing resources effectively to maximize healthcare delivery. With big data analytics, health organizations can identify areas where resources are sparsely utilized. This analysis can lead to improved strategic planning and optimal usage of healthcare assets. Improved resource allocation is widely regarded as essential in saving costs and enhancing service delivery. However, misinterpretation of data could lead to significant operational failures, highlighting the importance of proper data stewardship.
Workflow Optimization
Workflow optimization focuses on improving the procedures and processes within healthcare settings. By analyzing data from various sources, healthcare providers can identify bottlenecks in workflows. This approach leads to enhancements in efficiency, potentially decreasing wait times and improving patient satisfaction. As workflows become increasingly data-driven, healthcare institutions may face challenges in training staff to adapt to these systems. Nevertheless, the benefits of streamlined processes can greatly outweigh these hurdles.
"Big data in healthcare is not just about amassing information, but effectively using it to enhance patient outcomes and operational efficiency."
In summary, the impact of big data on healthcare delivery is multifaceted. From improving patient care methodologies to refining organizational operations, big data represents a significant advancement in healthcare. The key will be to navigate the inherent challenges effectively, ensuring that the benefits are maximized while minimizing potential risks.
Data Sources in Health Analytics
Data sources in health analytics form the foundation of evidence-based decision making in healthcare. The integration of diverse data sets leads to more accurate insights and fosters innovations across the healthcare landscape. This section explores the significant data sources utilized in health analytics and evaluates their benefits, challenges, and implications on patient care and health outcomes.
Electronic Health Records
Electronic Health Records (EHRs) act as a pivotal element in health analytics. These digital versions of patients’ paper charts offer comprehensive data on patient histories, treatments, and outcomes. EHRs enable healthcare providers to access and share critical information swiftly.
Importance of EHRs:
- Comprehensive Data Collection: EHRs collect a vast array of data including patient demographics, medication history, and lab results.
- Enhanced Communication: Health professionals can collaborate effectively, leading to streamlined care processes.
- Quality Improvement: They facilitate the implementation of clinical protocols that improve quality and safety.
Challenges exist, such as the need for standardized formats and practices across different institutions.
Wearable Devices and IoT
Wearable devices and the Internet of Things (IoT) also contribute significantly to health analytics. These technologies collect real-time data from users, providing insights into various health metrics.
Key Points about Wearable Devices and IoT:
- Continuous Monitoring: Patients can monitor chronic conditions more effectively with devices like heart rate monitors.
- Data Sharing: Users can share their health data with providers, supporting better-informed clinical decisions.
- Behavior Modification: These devices often motivate users towards healthier habits.
Despite their benefits, issues such as data accuracy and privacy concerns need to be addressed.
Genomic Data
Genomic data serves as another vital resource in health analytics. It encompasses the analysis of an individual's genetic information, helping researchers and clinicians to understand the genetic factors influencing health outcomes.
Significance of Genomic Data:
- Precision Medicine: Genomic data allows for tailored treatment plans based on a patient's genetic makeup.
- Disease Prediction: Identifying genetic predispositions can lead to early intervention strategies.
- Research Advancements: Genomic analytics contribute to discoveries in disease mechanisms and therapies.
However, the complexity and ethical implications of handling genomic data pose significant challenges.
Social Determinants of Health
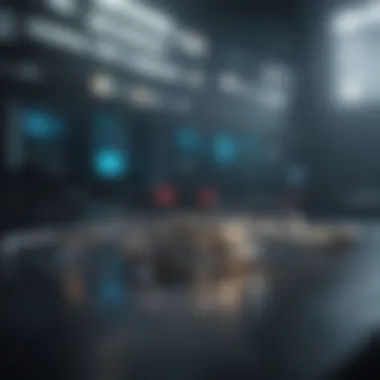
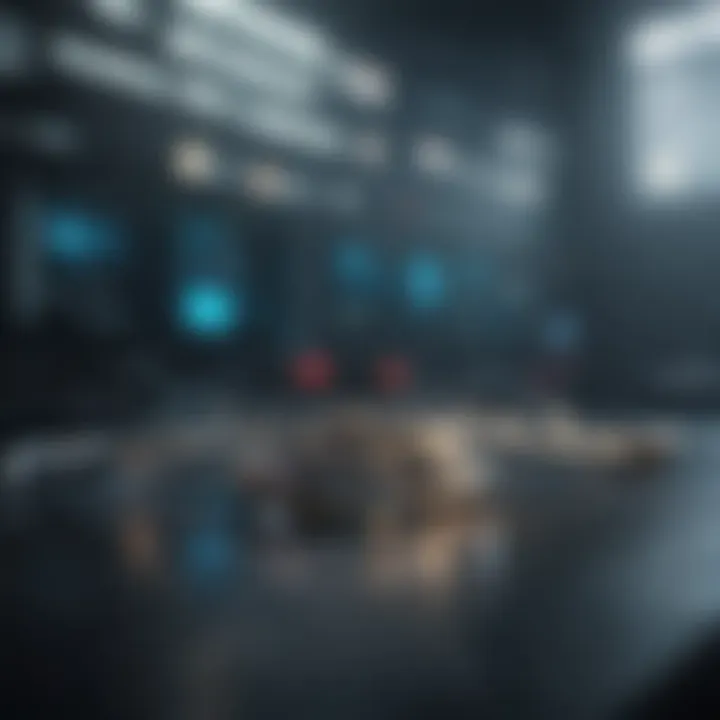
Social determinants of health include factors such as socioeconomic status, education, and environment. These determinants profoundly impact health outcomes and are vital to consider in health analytics.
Relevance of Social Determinants:
- Holistic Approach: Understanding social determinants enables a broader perspective on health issues.
- Targeted Interventions: Data on these factors helps in deploying focused programs to address health disparities.
- Policy Making: Integrating these determinants into analytics fosters informed decisions at the policy level.
The integration of social determinants must be approached carefully due to the complexity of these influences within healthcare.
Conclusion: Data sources such as EHRs, wearable devices, genomic information, and social determinants are crucial in health analytics. They provide valuable insights that enhance patient care and drive improvements in health systems. Health analysts must also consider the challenges associated with these data sources to maximize their benefits.
Analytical Techniques in Health Analytics
Predictive Analytics
Predictive analytics involve using historical data to forecast future outcomes. In health analytics, this can mean predicting patient readmissions or potential disease outbreaks. Machine learning algorithms and statistical models play a key role in this area. By evaluating past data on patient demographics, treatment responses, and other relevant factors, predictive analytics can identify risk factors for individual patients. This not only helps in early intervention but also enables tailored treatment plans.
One significant application is in managing chronic diseases. For instance, diabetes patients can benefit from predictive models that suggest lifestyle changes based on their health metrics. These proactive measures are vital in preventing further complications, making predictive analytics a valuable tool for healthcare providers.
Descriptive Analytics
Descriptive analytics focuses on providing insights into what has happened in the past. It is essential for summarizing data into meaningful metrics. This technique utilizes statistics to provide a snapshot of operational effectiveness and patient demographics.
For example, hospitals may use descriptive analytics to evaluate overall patient satisfaction scores or the average length of stay. This approach can reveal trends, such as an increase in emergency room visits during flu season. Understanding these trends allows healthcare administrators to allocate resources more effectively and forecast future needs.
Moreover, descriptive analytics can assist in evaluating treatment outcomes across various patient populations. This data segment can help identify which treatments are most effective in particular demographics, driving improvements in care delivery.
Prescriptive Analytics
Prescriptive analytics go a step further by not only predicting what might happen but also providing recommendations for actions. This technique utilizes optimization and simulation algorithms to suggest the best course of action for given scenarios. In healthcare, prescriptive analytics can be applied in various areas such as resource management and treatment planning.
For instance, a hospital might use this analytics type to determine the optimal staffing levels for different shifts based on predicted patient volumes. This ensures that patient needs are met without overstaffing or understaffing, thus balancing cost and quality of care. Additionally, prescriptive analytics can enhance clinical pathways by recommending specific treatment modalities for patients based on their conditions and treatment history.
"The shift from analysis to prescription signifies a movement towards actionable insights that can enhance healthcare outcomes."
In summary, these analytical techniques—predictive, descriptive, and prescriptive—play a pivotal role in enhancing health analytics. They enable healthcare organizations to harness vast amounts of data effectively, leading to better resource management, improved patient care, and fostering an environment of continuous improvement.
Challenges in Big Data Health Analytics
Data Privacy and Security Concerns
Data privacy and security are paramount in the health sector. With the increased volume of health data collected from various sources, the risk of data breaches also rises. Health organizations must comply with regulations like the Health Insurance Portability and Accountability Act (HIPAA) in the U.S. These regulations emphasize patient confidentiality and require organizations to implement strict data protection measures.
Ensuring the security of sensitive health information is not only about compliance but also about building trust with patients. If patients feel their data is not secure, they may hesitate to share information that could improve their care. Therefore, it is essential for health organizations to invest in robust cybersecurity measures and regular audits to identify vulnerabilities.
Data Integration Issues
Data integration can be a significant hurdle in utilizing big data for health analytics. The vast array of different data formats and sources creates complexity. For example, electronic health records from various systems may not align, leading to inconsistencies. Additionally, wearable devices might track different health metrics than those recorded in traditional medical records.
To address this issue, health organizations must develop strategies for effective data integration. This may involve adopting standardized data formats and using middleware solutions that allow disparate systems to communicate. By creating a unified data ecosystem, organizations can derive more comprehensive insights and improve patient outcomes.
Interoperability of Systems
Interoperability refers to the ability of different health information systems to work together. In many cases, systems used in hospitals and clinics do not communicate effectively. This leads to silos of information that hinder comprehensive patient care. For instance, if a patient's health history is not accessible across various providers, it could lead to misdiagnoses or redundant testing.
Achieving interoperability requires a concerted effort from all stakeholders in healthcare. This may include collaboration between technology vendors, healthcare providers, and regulatory bodies. Implementing interoperable systems can enhance care coordination, facilitate better decision-making, and ultimately improve health outcomes.
"Without interoperability, we are not fully utilizing the potential of big data – insight is limited by access."
Ethical Considerations in Data Usage
The increasing use of big data in healthcare brings several ethical considerations that must be addressed. As health analytics evolve, the focus shifts towards ensuring that data usage aligns with ethical principles. Understanding the implications of data handling is vital for maintaining trust and safeguarding patient rights. Ethical data practices are not just regulatory needs; they encompass a broader responsibility towards individuals whose data is analyzed.
Key elements to consider in this area include informed consent and the challenges of bias and fairness. These topics are essential in discussing how ethics weave into the healthcare fabric, impacting both patients and providers.
Informed Consent
Informed consent is a cornerstone of ethical research and data usage in healthcare. It refers to the process through which patients understand and agree to the data usage regarding their personal health information. This includes clarity on what data is collected, how it will be used, and the potential impacts on their privacy.
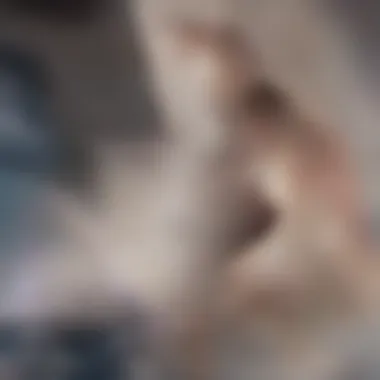
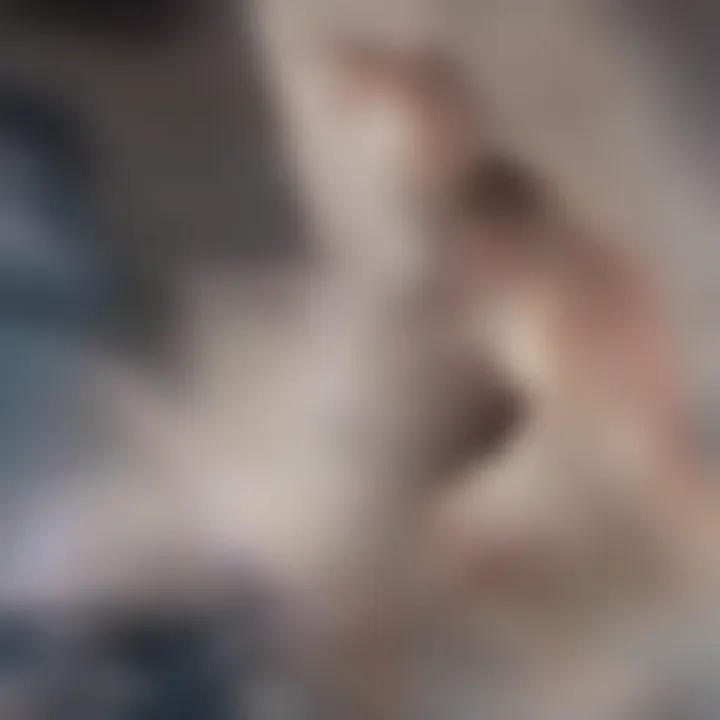
The importance of informed consent cannot be overstated. Patients must know that their data contributes to analyses that may enhance overall healthcare outcomes. Furthermore, patients should have the option to opt-out without repercussions. The challenge here lies in ensuring that the consent process is not merely a formality. It should be comprehensive yet comprehensible, balancing detail with simplicity.
Some benefits of informed consent include:
- Increased Trust: When patients know how their data is being used, they are more likely to participate in data-sharing initiatives.
- Empowerment: Patients feel more in control over their health information.
- Legal Compliance: Proper consent processes align with regulations such as the Health Insurance Portability and Accountability Act (HIPAA).
Bias and Fairness
Bias in health data can lead to unfair treatment outcomes and exacerbates existing health disparities. Large data sets sometimes contain imbalances that reflect societal inequalities, affecting predictive algorithms and adversely impacting certain demographics. Addressing bias is crucial to ensure equitable healthcare delivery.
Fairness in big data health analytics is a complex issue. Organizations must strive to eliminate biases that arise from various sources, including:
- Sampling Bias: Data gathered may not accurately represent the target population.
- Historical Bias: Prior inequalities reflected in data can perpetuate discriminatory practices.
- Algorithmic Bias: Machine learning models might favor one group over another based on non-representative training data.
To tackle bias, healthcare organizations can adopt several strategies, such as:
- Conducting regular audits of datasets for fairness and representation.
- Involving a diverse group of stakeholders in the design phases of data projects.
- Implementing transparent algorithms that allow for ongoing evaluation and adjustment.
"The future of healthcare will depend significantly on how we navigate the ethical complexities surrounding big data usage."
By addressing these ethical considerations, healthcare systems can improve patient outcomes while fostering trust and accountability within their communities.
Future Trends in Health Analytics
The landscape of health analytics is constantly evolving. Understanding the future trends is essential for stakeholders in the healthcare industry. These trends not only shape how data is analyzed but also influence how patient care is delivered. As technology continues to advance, new methodologies and tools emerge, leading to enhanced outcomes. This section will explore two significant trends: artificial intelligence and machine learning, as well as blockchain technology.
Artificial Intelligence and Machine Learning
Artificial intelligence (AI) and machine learning (ML) are pivotal in transforming health analytics. Their ability to analyze vast amounts of data can uncover insights that traditional methods may overlook. For instance, predictive analytics driven by AI helps in early diagnosis and personalized treatment plans.
Benefits of AI in healthcare include:
- Enhancing accuracy in diagnosis.
- Predicting patient outcomes based on historical data.
- Optimizing resource management within healthcare systems, allowing for improved efficiency.
Implementing AI solutions requires careful consideration of data privacy. With better algorithms, the potential for bias also poses questions about fairness in health outcomes. To mitigate these risks, ongoing research and robust validation processes are necessary.
In summary, AI and ML represent a significant shift in health analytics. Their future integration into healthcare practices promises improved patient management and more customized healthcare solutions, thus underscoring their relevance in contemporary health analytics.
Blockchain Technology
Blockchain technology presents a novel approach to security and interoperability in health data management. Its decentralized and immutable nature ensures that patient data remains secure and tamper-proof. This is essential in an age where data breaches are common.
Key advantages of blockchain in health analytics include:
- Increased data integrity by providing a verified history of all transactions.
- Enhanced interoperability as various healthcare systems can share information securely.
- Empowerment of patients to control their own health records, fostering trust and transparency.
While blockchain holds promise, challenges remain in its implementation. Technical barriers, such as scalability and the standardization of protocols, require attention. Additionally, regulatory frameworks need to adapt to accommodate this emerging technology.
As healthcare continues to leverage both artificial intelligence and blockchain, stakeholders must remain aware of the ethical implications and ensure that technology serves the ultimate goal of improving patient care.
Case Studies
Successful Implementations
Successful implementations of big data analytics in healthcare illustrate how various organizations have harnessed data for tangible benefits. One notable example is the use of predictive analytics in hospitals, which helps in forecasting patient admission rates. By analyzing historical data, healthcare facilities can allocate resources more effectively, ensuring that they are prepared during peak times. This proactive approach reduces wait times for patients and improves overall satisfaction.
Furthermore, organizations like Mount Sinai Health System have adopted machine learning algorithms to analyze electronic health records. This approach has allowed them to identify high-risk patients for conditions such as diabetes or heart disease. Early intervention has led to significant healthcare savings and better health outcomes for the patient population.
Lessons Learned
Analyzing the successes from case studies also uncovers critical lessons learned throughout various implementations. One of the most significant takeaways is the importance of data quality and integration. Even the most sophisticated analytics tools will only yield effective results if the underlying data is accurate and comprehensive. Organizations must prioritize establishing robust data governance frameworks to ensure high data quality.
Additionally, it is clear that engaging with clinical staff and stakeholders is crucial. The success of big data initiatives relies on the end-users being trained and comfortable with analytics tools. Hospitals that prioritize user-friendly systems and ongoing education for staff experience smoother transitions and better outcomes.
"Without effective collaboration between data scientists and clinicians, the potential of health analytics can be underutilized."
The End
Big data analytics is revolutionizing health care by enabling data-driven decision-making. This becomes evident when we consider how vast amounts of data from electronic health records, wearable devices, and even social determinants are converged to provide deeper insights. The ability to predict patient outcomes and personalize treatment plans can improve patient care dramatically. Furthermore, this approach aids in the identification of trends and patterns that were previously obscure to health professionals.
Another element to consider is the operational efficiency that comes from utilizing big data. Healthcare institutions leverage analytics to optimize workflows and allocate resources more effectively. This competence ultimately results in cost reductions and increased focus on patient care, leading to better health outcomes.
Yet, while the benefits are substantial, there are also challenges that must be addressed. These range from data privacy and security to ethical and integration issues. Future trends, such as advancements in artificial intelligence and blockchain technology, offer promising solutions but also introduce new complexities that require careful navigation.
"As big data continues to evolve, its influence on healthcare delivery and research methodologies underscores its potential to transform patient care fundamentally."